BiC-Net: Learning Efficient Spatio-temporal Relation for Text-Video Retrieval
ACM TRANSACTIONS ON MULTIMEDIA COMPUTING COMMUNICATIONS AND APPLICATIONS(2024)
摘要
The task of text-video retrieval aims to understand the correspondence between language and vision and has gained increasing attention in recent years. Recent works have demonstrated the superiority of local spatio-temporal relation learning with graph-based models. However, most existing graph-based models are handcrafted and depend heavily on expert knowledge and empirical feedback, which may be unable to mine the high-level fine-grained visual relations effectively. These limitations result in their inability to distinguish videos with the same visual components but different relations. To solve this problem, we propose a novel cross-modal retrieval framework, Bi-Branch Complementary Network (BiC-Net), which modifies Transformer architecture to effectively bridge text-video modalities in a complementary manner via combining local spatio-temporal relation and global temporal information. Specifically, local video representations are encoded using multiple Transformer blocks and additional residual blocks to learn fine-grained spatio-temporal relations and long-term temporal dependency, calling the module a Fine-grained Spatio-temporal Transformer (FST). Global video representations are encoded using a multi-layer Transformer block to learn global temporal features. Finally, we align the spatio-temporal relation and global temporal features with the text feature on two embedding spaces for cross-modal text-video retrieval. Extensive experiments are conducted on MSR-VTT, MSVD, and YouCook2 datasets. The results demonstrate the effectiveness of our proposed model. Our code is public at https://github.com/lionel-hing/BiC-Net.
更多查看译文
关键词
Text-video retrieval,spatio-temporal relation,bi-branch complementary network
AI 理解论文
溯源树
样例
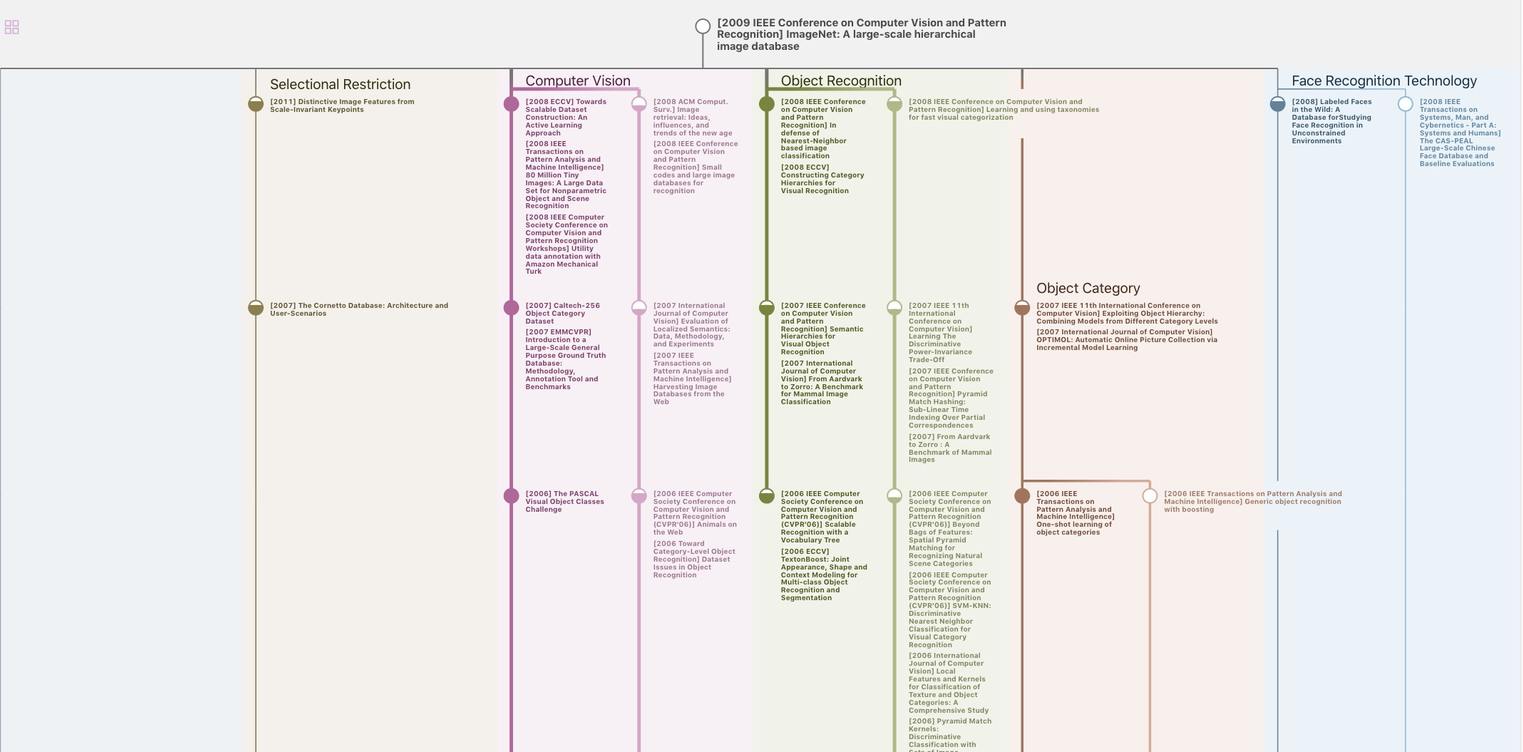
生成溯源树,研究论文发展脉络
Chat Paper
正在生成论文摘要