Deep learning at base-resolution reveals cis-regulatory motif syntax
bioRxiv (Cold Spring Harbor Laboratory)(2020)
摘要
Genes are regulated by cis-regulatory sequences, which contain transcription factor (TF) binding motifs in specific arrangements (syntax). To understand how motif syntax influences TF binding, we train a deep learning model, BPNet, that uses DNA sequence to predict base-resolution ChIP-nexus binding profiles of four pluripotency TFs Oct4, Sox2, Nanog, and Klf4. We interpret the model to accurately map hundreds of thousands of motifs in the genome, learn predictive motif representations, and identify rules by which specific motifs interact. We find that instances of strict motif spacing are largely due to retrotransposons, but that soft motif syntax influences TF binding in a directional manner. Most strikingly, Nanog shows a strong preference for binding with helical periodicity. We validate our model using CRISPR-induced point mutations, demonstrating that interpretable deep learning models are a powerful approach to uncover the motifs and syntax of cis-regulatory sequences.
更多查看译文
关键词
deep learning,base-resolution,cis-regulatory
AI 理解论文
溯源树
样例
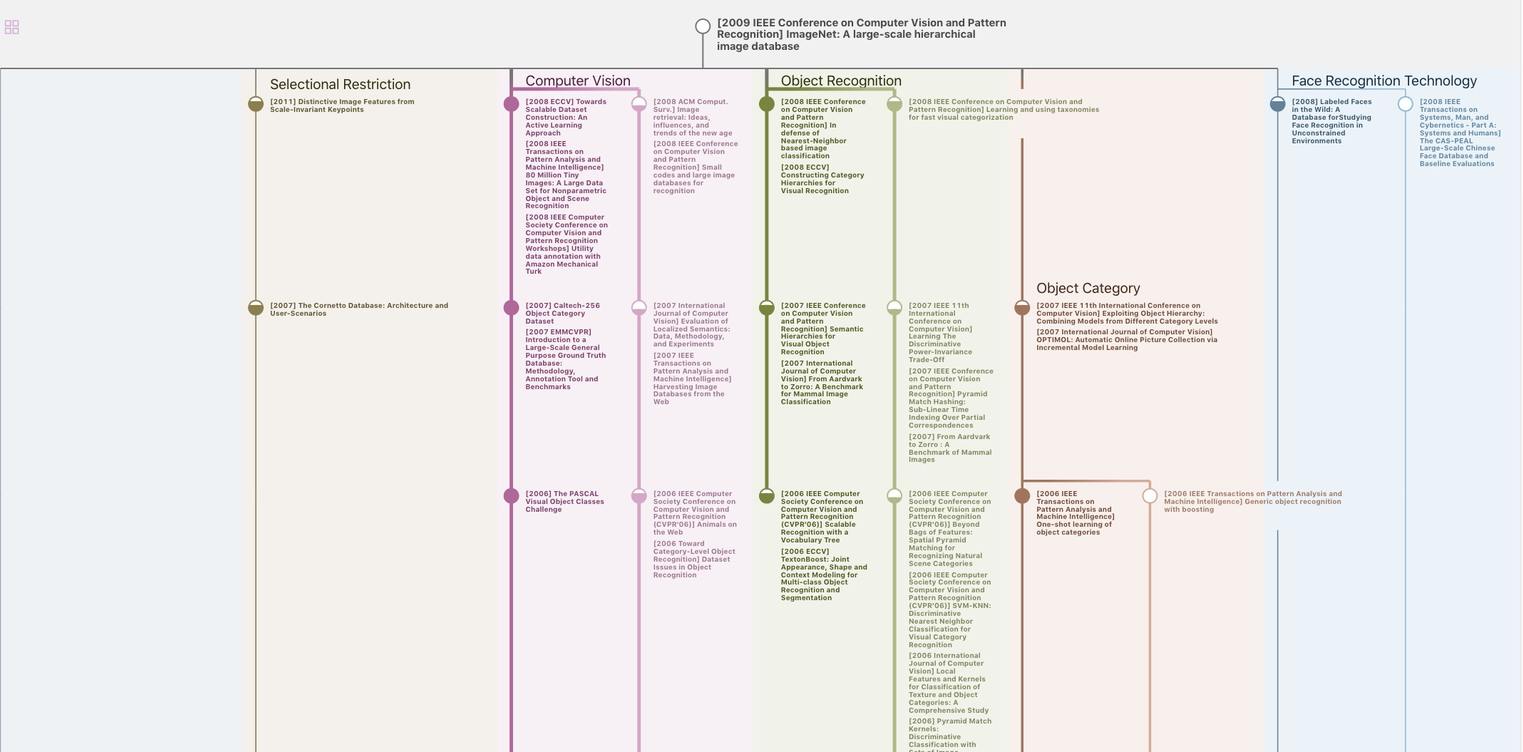
生成溯源树,研究论文发展脉络
Chat Paper
正在生成论文摘要