Evaluating Evaluation Metrics: A Framework for Analyzing NLG Evaluation Metrics using Measurement Theory.
EMNLP 2023(2023)
摘要
We address a fundamental challenge in Natural Language Generation (NLG) model evaluation---the design and evaluation of evaluation metrics. Recognizing the limitations of existing automatic metrics and noises from how current human evaluation was conducted, we propose MetricEval, a framework informed by measurement theory, the foundation of educational test design, for conceptualizing and evaluating the reliability and validity of NLG evaluation metrics. The framework formalizes the source of measurement error and offers statistical tools for evaluating evaluation metrics based on empirical data. With our framework, one can quantify the uncertainty of the metrics to better interpret the result. To exemplify the use of our framework in practice, we analyzed a set of evaluation metrics for summarization and identified issues related to conflated validity structure in human-eval and reliability in LLM-based metrics. Through MetricEval, we aim to promote the design, evaluation, and interpretation of valid and reliable metrics to advance robust and effective NLG models.
更多查看译文
关键词
nlg evaluation metrics,measurement
AI 理解论文
溯源树
样例
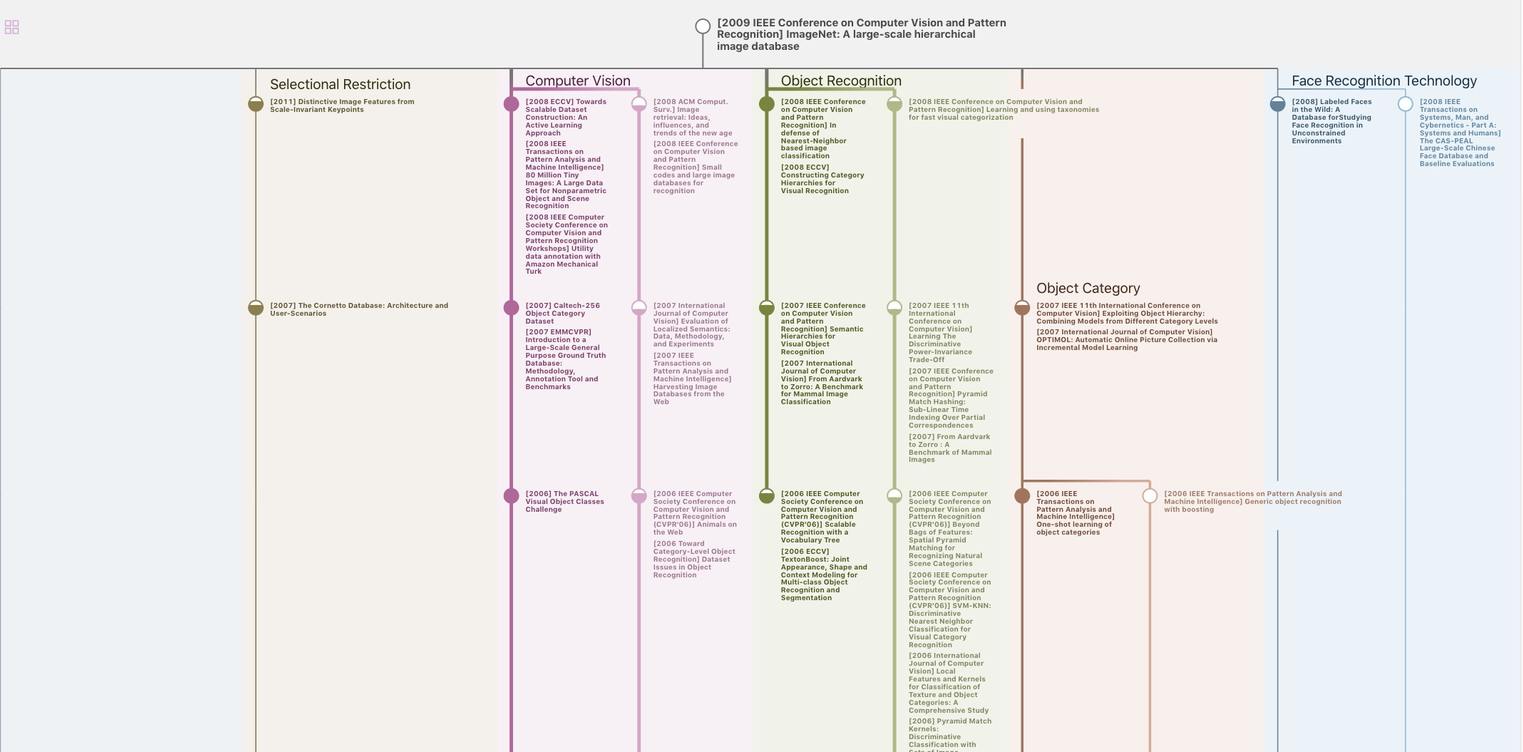
生成溯源树,研究论文发展脉络
Chat Paper
正在生成论文摘要