Representation Projection Invariance Mitigates Representation Collapse.
EMNLP 2023(2023)
摘要
Fine-tuning contextualized representations learned by pre-trained language models remains a prevalent practice in NLP. However, fine-tuning can lead to representation degradation (also known as representation collapse), which may result in instability, sub-optimal performance, and weak generalization. In this paper, we propose Representation Projection Invariance (REPINA), a novel regularization method to maintain the information content of representation and reduce representation collapse during fine-tuning by discouraging undesirable changes in the representations. We study the empirical behavior of the proposed regularization in comparison to 5 comparable baselines across 13 language understanding tasks (GLUE benchmark and six additional datasets). When evaluating in-domain performance, REPINA consistently outperforms other baselines on most tasks (10 out of 13). Additionally, REPINA improves out-of-distribution performance. We also demonstrate its effectiveness in few-shot settings and robustness to label perturbation. As a by-product, we extend previous studies of representation collapse and propose several metrics to quantify it. Our empirical findings show that our approach is significantly more effective at mitigating representation collapse.
更多查看译文
关键词
representation,invariance
AI 理解论文
溯源树
样例
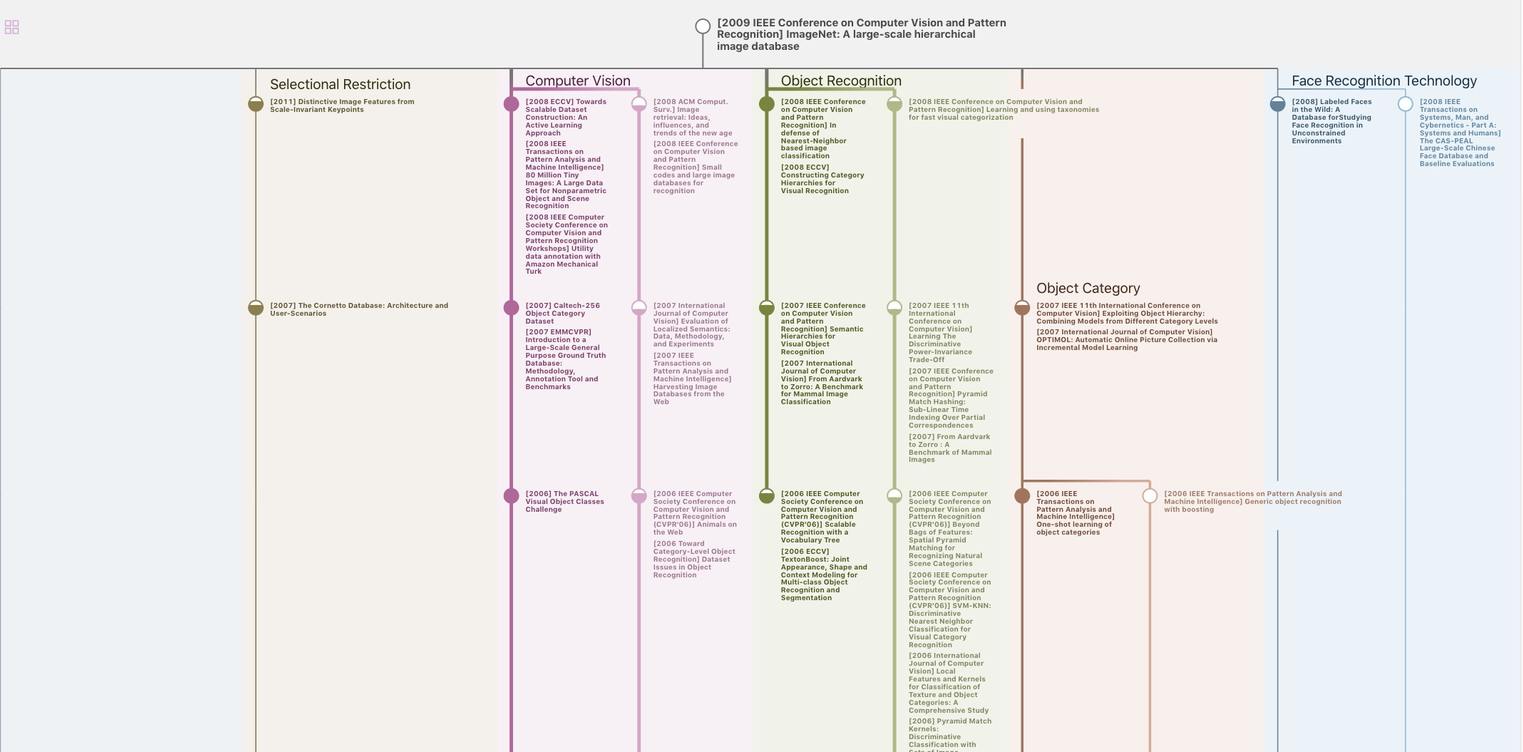
生成溯源树,研究论文发展脉络
Chat Paper
正在生成论文摘要