Can Large Language Models Capture Dissenting Human Voices?
EMNLP 2023(2023)
摘要
Large language models (LLMs) have shown impressive achievements in solving a broad range of tasks. Augmented by instruction fine-tuning, LLMs have also been shown to generalize in zero-shot settings as well. However, whether LLMs closely align with the human disagreement distribution has not been well-studied, especially within the scope of natural language inference (NLI). In this paper, we evaluate the performance and alignment of LLM distribution with humans using two different techniques to estimate the multinomial distribution: Monte Carlo Estimation (MCE) and Log Probability Estimation (LPE). As a result, we show LLMs exhibit limited ability in solving NLI tasks and simultaneously fail to capture human disagreement distribution. The inference and human alignment performances plunge even further on data samples with high human disagreement levels, raising concerns about their natural language understanding (NLU) ability and their representativeness to a larger human population.
更多查看译文
关键词
large language models infer
AI 理解论文
溯源树
样例
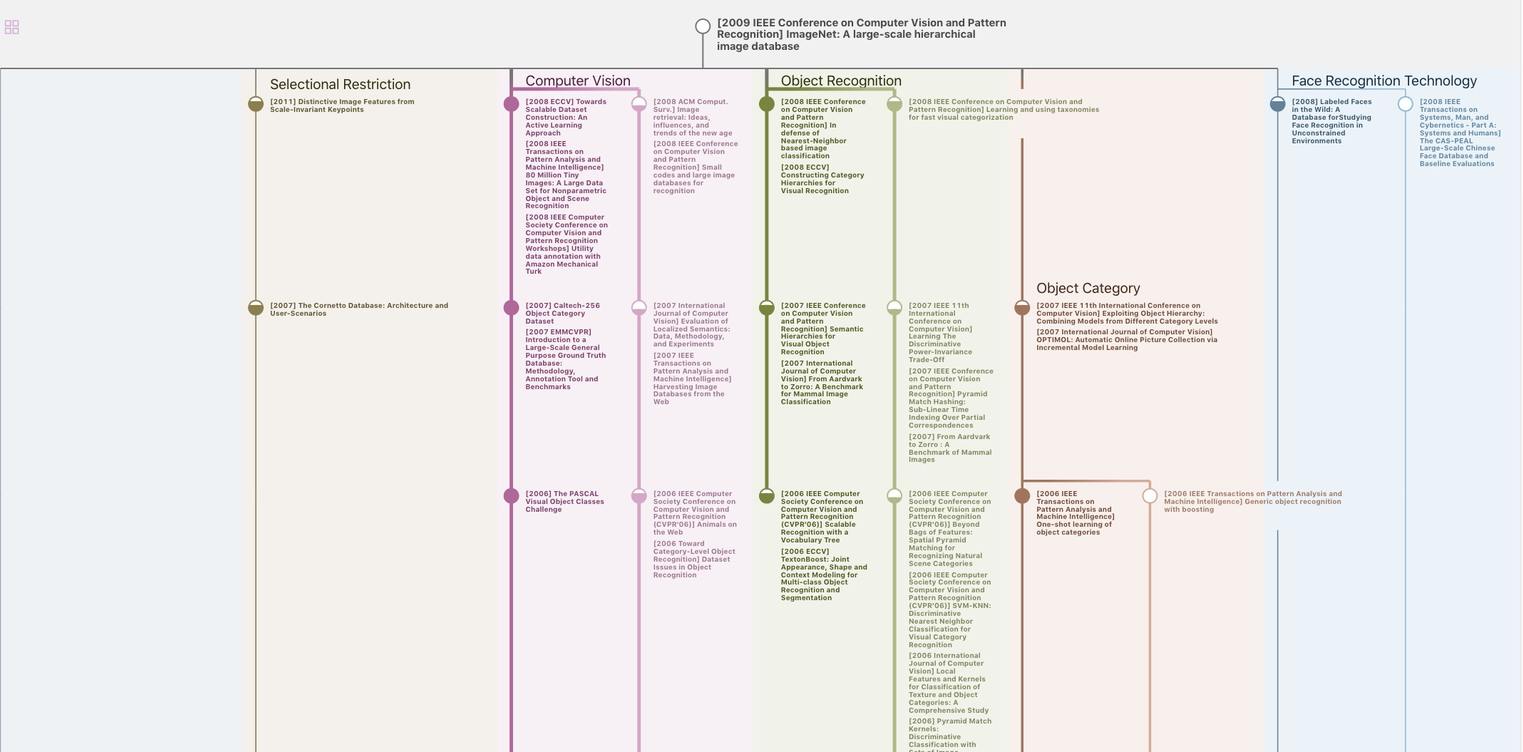
生成溯源树,研究论文发展脉络
Chat Paper
正在生成论文摘要