TRIP: Accelerating Document-level Multilingual Pre-training via Triangular Document-level Pre-training on Parallel Data Triplets.
EMNLP 2023(2023)
摘要
Despite the success of multilingual sequence-to-sequence pre-training, most existing approaches rely on document-level monolingual corpora in many different languages, sentence-level bilingual corpora,\footnote{In this paper, we use bilingual corpora to denote parallel corpora with bilingual translation pairs in many different language pairs, each consisting of two sentences/documents with the same meaning written in different languages. We use trilingual corpora to denote parallel corpora with trilingual translation pairs in many different language combinations, each consisting of three sentences/documents.} and sometimes synthetic document-level bilingual corpora. This hampers the performance with cross-lingual document-level tasks such as document-level translation. Hence, we propose to mine and leverage document-level trilingual parallel corpora to improve sequence-to-sequence multilingual pre-training. We present \textbf{Tri}angular Document-level \textbf{P}re-training (\textbf{TRIP}) as the first in the field to accelerate the conventional monolingual and bilingual objectives into a trilingual objective with a novel method called Grafting. Experiments show that TRIP achieves several strong state-of-the-art (SOTA) scores on three multilingual document-level machine translation benchmarks and one cross-lingual abstractive summarization benchmark, including consistent improvements by up to 3.11 d-BLEU points and 8.9 ROUGE-L points.
更多查看译文
AI 理解论文
溯源树
样例
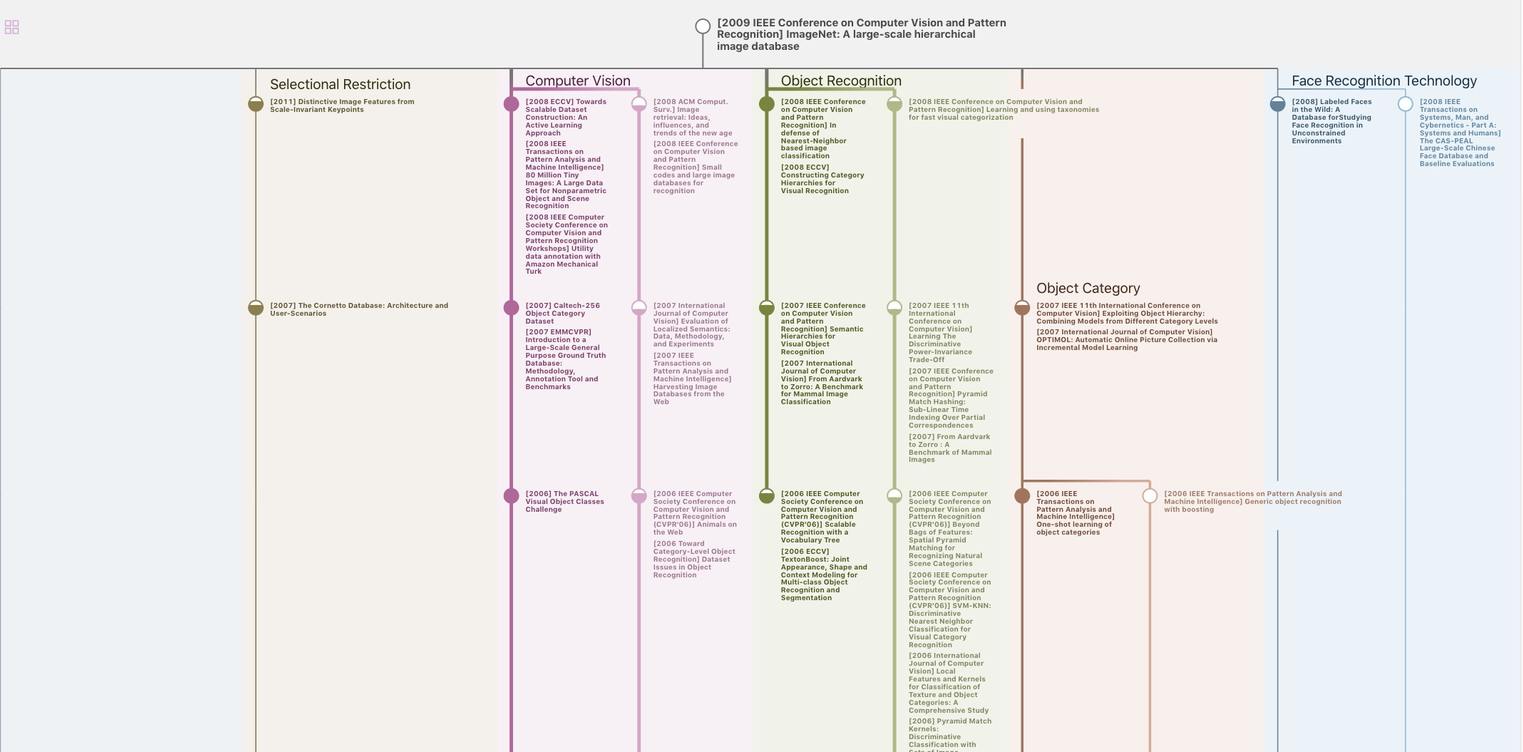
生成溯源树,研究论文发展脉络
Chat Paper
正在生成论文摘要