Retrieval-Augmented Parsing for Complex Graphs by Exploiting Structure and Uncertainty.
EMNLP 2023(2023)
摘要
Retrieval augmentation enhances generative language models by retrieving informative exemplars relevant for output prediction.
However, in realistic graph parsing problems where the output space is large and complex, classic retrieval methods based on input-sentence similarity can fail to identify the most informative exemplars that target graph elements the model is most struggling about, leading to suboptimal retrieval and compromised prediction under limited retrieval budget.
In this work, we improve retrieval-augmented parsing for complex graph problems by exploiting two unique sources of information (1) structural similarity and (2) model uncertainty.
We propose $\textit{\textbf{S}tructure-aware and \textbf{U}ncertainty-\textbf{G}uided \textbf{A}daptive \textbf{R}etrieval} \textbf{(SUGAR)} $ that first quantify the model uncertainty in graph prediction and identify its most uncertain subgraphs, and then retrieve exemplars based on their structural similarity with the identified uncertain subgraphs.
On a suite of real-world parsing benchmarks with non-trivial graph structure (SMCalflow and E-commerce), SUGAR exhibits a strong advantage over its classic counterparts that do not leverage structure or model uncertainty.
更多查看译文
AI 理解论文
溯源树
样例
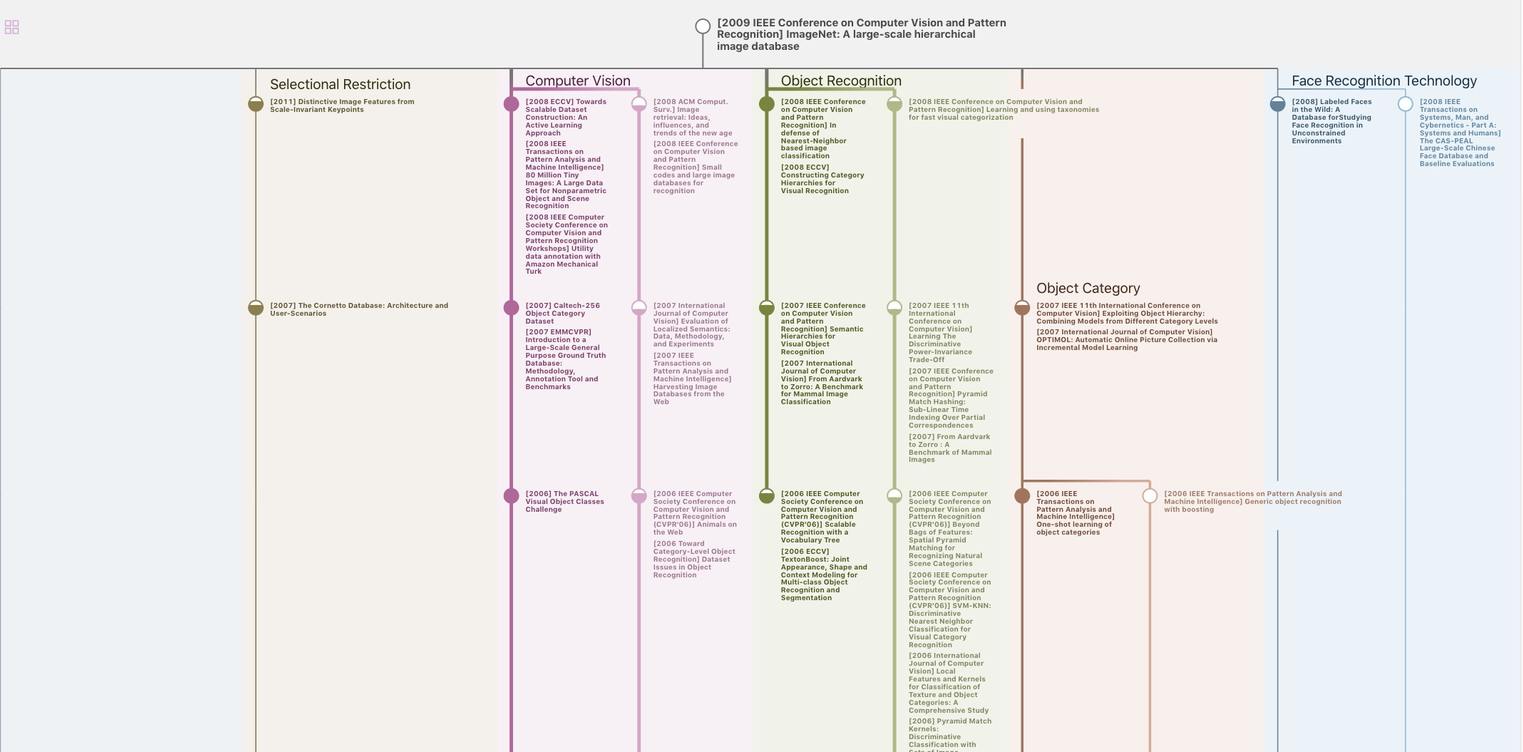
生成溯源树,研究论文发展脉络
Chat Paper
正在生成论文摘要