FactSpotter: Evaluating the Factual Faithfulness of Graph-to-Text Generation.
EMNLP 2023(2023)
摘要
Graph-to-text (G2T) generation takes a graph as input and aims to generate a fluent and faith- ful textual representation of the information in the graph. The task has many applications, such as dialogue generation and question an- swering. In this work, we investigate to what extent the G2T generation problem is solved for previously studied datasets, and how pro- posed metrics perform when comparing generated texts. To help address their limitations, we propose a new metric that correctly identifies factual faithfulness, i.e., given a triple (subject, predicate, object), it decides if the triple is present in a generated text. We show that our metric FactSpotter achieves the highest correlation with human annotations on data correct- ness, data coverage, and relevance. In addition, FactSpotter can be used as a plug-in feature to improve the factual faithfulness of existing models. Finally, we investigate if existing G2T datasets are still challenging for state-of-the-art models. Our code is available online: https://github.com/guihuzhang/FactSpotter.
更多查看译文
关键词
factual faithfulness,generation,graph-to-text
AI 理解论文
溯源树
样例
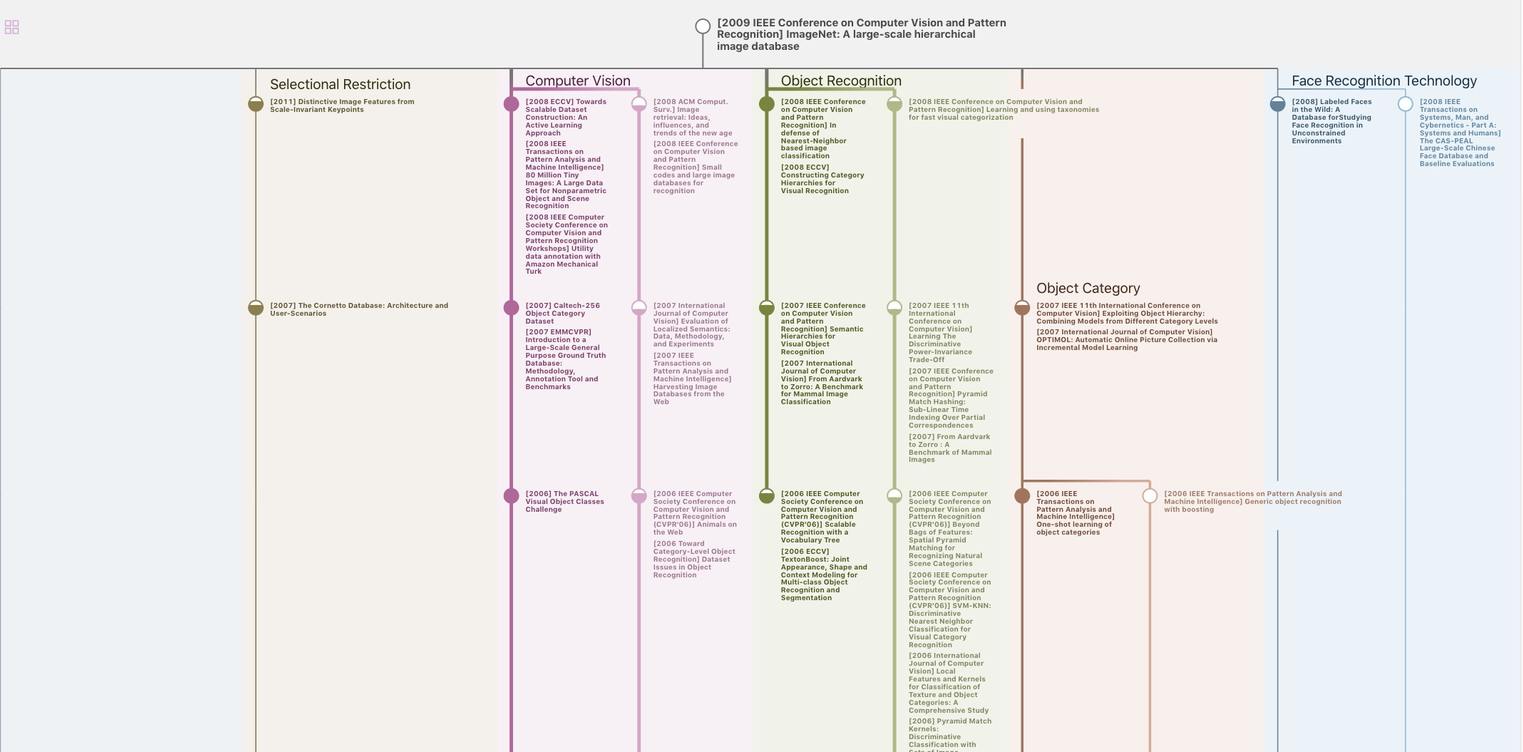
生成溯源树,研究论文发展脉络
Chat Paper
正在生成论文摘要