Text encoders bottleneck compositionality in contrastive vision-language models.
EMNLP 2023(2023)
摘要
Performant vision-language (VL) models like CLIP represent captions using a single vector. How much information about language is lost in this bottleneck? We first curate CompPrompts, a set of increasingly compositional image captions that VL models should be able to capture (e.g., single object, to object+property, to multiple interacting objects). Then, we train text-only recovery probes that aim to reconstruct captions from single-vector text representations produced by several VL models. This approach does not require images, allowing us to test on a broader range of scenes compared to prior work. We find that: 1) CLIP’s text encoder falls short on more compositional inputs, including object relationships, attribute-object association, counting, and negations; 2) some text encoders work significantly better than others; and 3) text-only recovery performance predicts multimodal matching performance on ControlledImCaps: a new evaluation benchmark we collect and release consisting of fine-grained compositional images and captions. Specifically, our results suggest text-only recoverability is a necessary (but not sufficient) condition for modeling compositional factors in contrastive VL models. We release our datasets and code.
更多查看译文
关键词
text encoders,vision-language
AI 理解论文
溯源树
样例
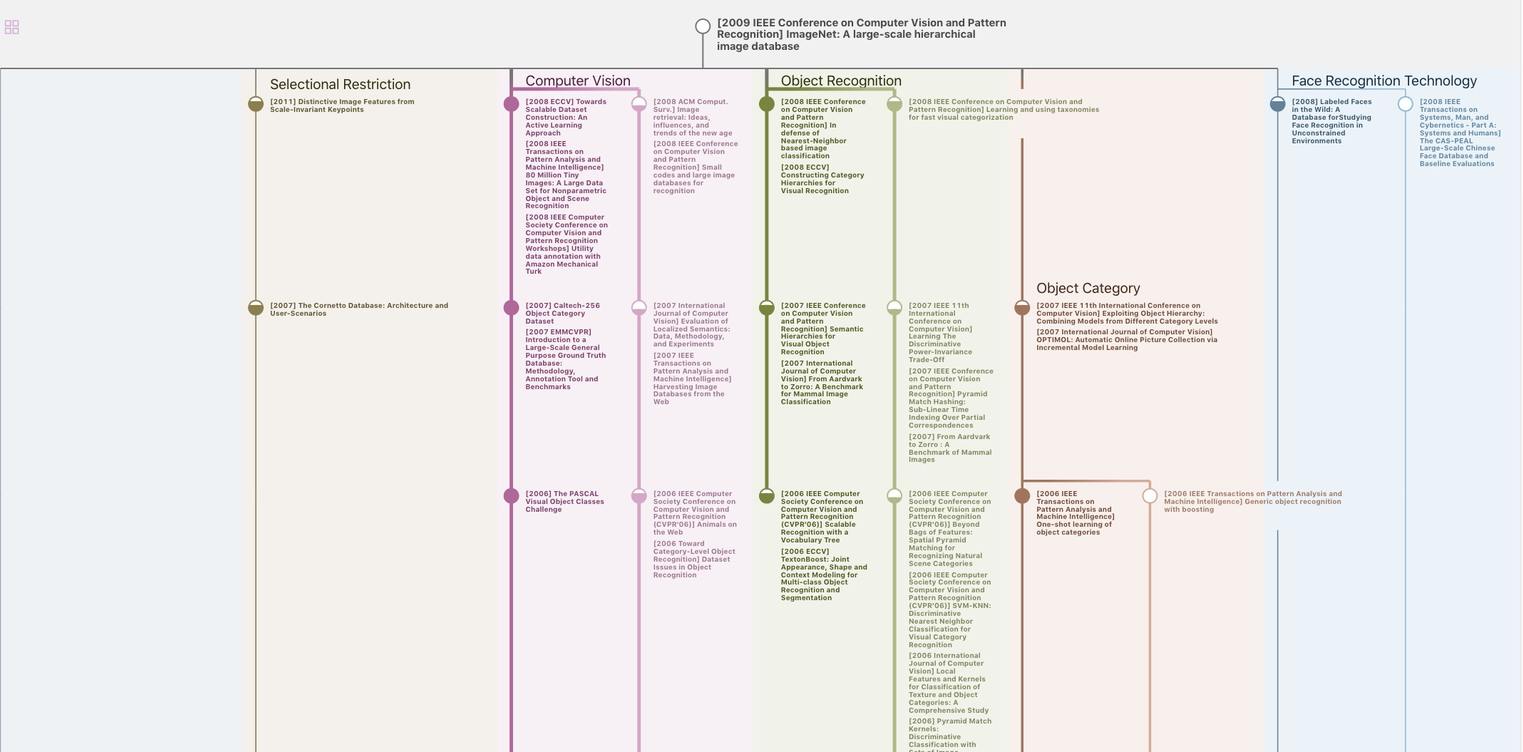
生成溯源树,研究论文发展脉络
Chat Paper
正在生成论文摘要