Leveraging Affect Transfer Learning for Behavior Prediction in an Intelligent Tutoring System
arXiv (Cornell University)(2020)
摘要
In this work, we propose a video-based transfer learning approach for predicting problem outcomes of students working with an intelligent tutoring system (ITS). By analyzing a student's face and gestures, our method predicts the outcome of a student answering a problem in an ITS from a video feed. Our work is motivated by the reasoning that the ability to predict such outcomes enables tutoring systems to adjust interventions, such as hints and encouragement, and to ultimately yield improved student learning. We collected a large labeled dataset of student interactions with an intelligent online math tutor consisting of 68 sessions, where 54 individual students solved 2,749 problems. The dataset is public and available at https://www.cs.bu.edu/faculty/betke/research/learning/ . Working with this dataset, our transfer-learning challenge was to design a representation in the source domain of pictures obtained "in the wild" for the task of facial expression analysis, and transferring this learned representation to the task of human behavior prediction in the domain of webcam videos of students in a classroom environment. We developed a novel facial affect representation and a user-personalized training scheme that unlocks the potential of this representation. We designed several variants of a recurrent neural network that models the temporal structure of video sequences of students solving math problems. Our final model, named ATL-BP for Affect Transfer Learning for Behavior Prediction, achieves a relative increase in mean F-score of 50% over the state-of-the-art method on this new dataset.
更多查看译文
关键词
affect transfer learning,behavior prediction
AI 理解论文
溯源树
样例
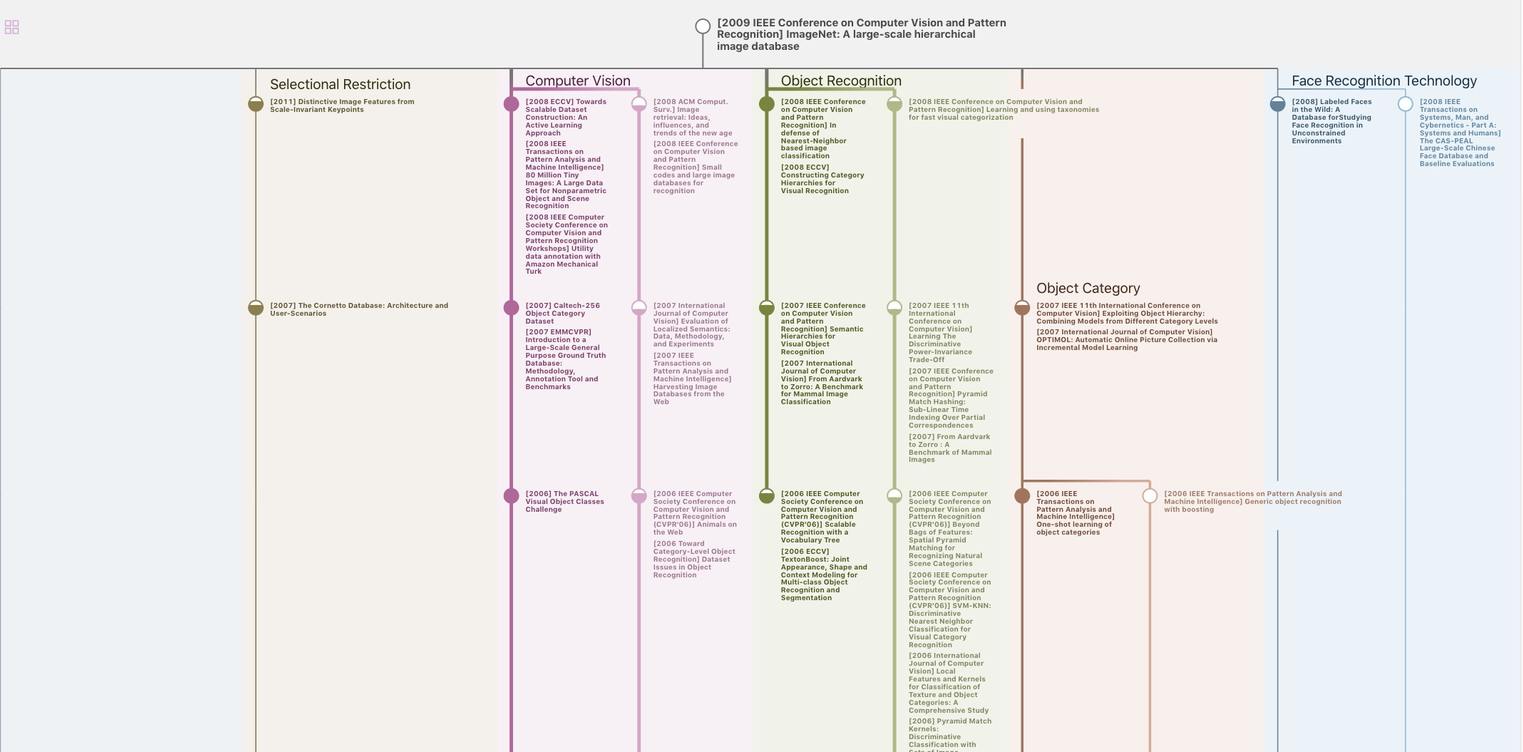
生成溯源树,研究论文发展脉络
Chat Paper
正在生成论文摘要