Disentanglement with Hyperspherical Latent Spaces using Diffusion Variational Autoencoders.
arXiv (Cornell University)(2020)
摘要
A disentangled representation of a data set should be capable of recovering the underlying factors that generated it. One question that arises is whether using Euclidean space for latent variable models can produce a disentangled representation when the underlying generating factors have a certain geometrical structure. Take for example the images of a car seen from different angles. The angle has a periodic structure but a 1-dimensional representation would fail to capture this topology. How can we address this problem? The submissions presented for the first stage of the NeurIPS2019 Disentanglement Challenge consist of a Diffusion Variational Autoencoder ($\Delta$VAE) with a hyperspherical latent space which can, for example, recover periodic true factors. The training of the $\Delta$VAE is enhanced by incorporating a modified version of the Evidence Lower Bound (ELBO) for tailoring the encoding capacity of the posterior approximate.
更多查看译文
关键词
diffusion variational autoencoders,hyperspherical latent spaces
AI 理解论文
溯源树
样例
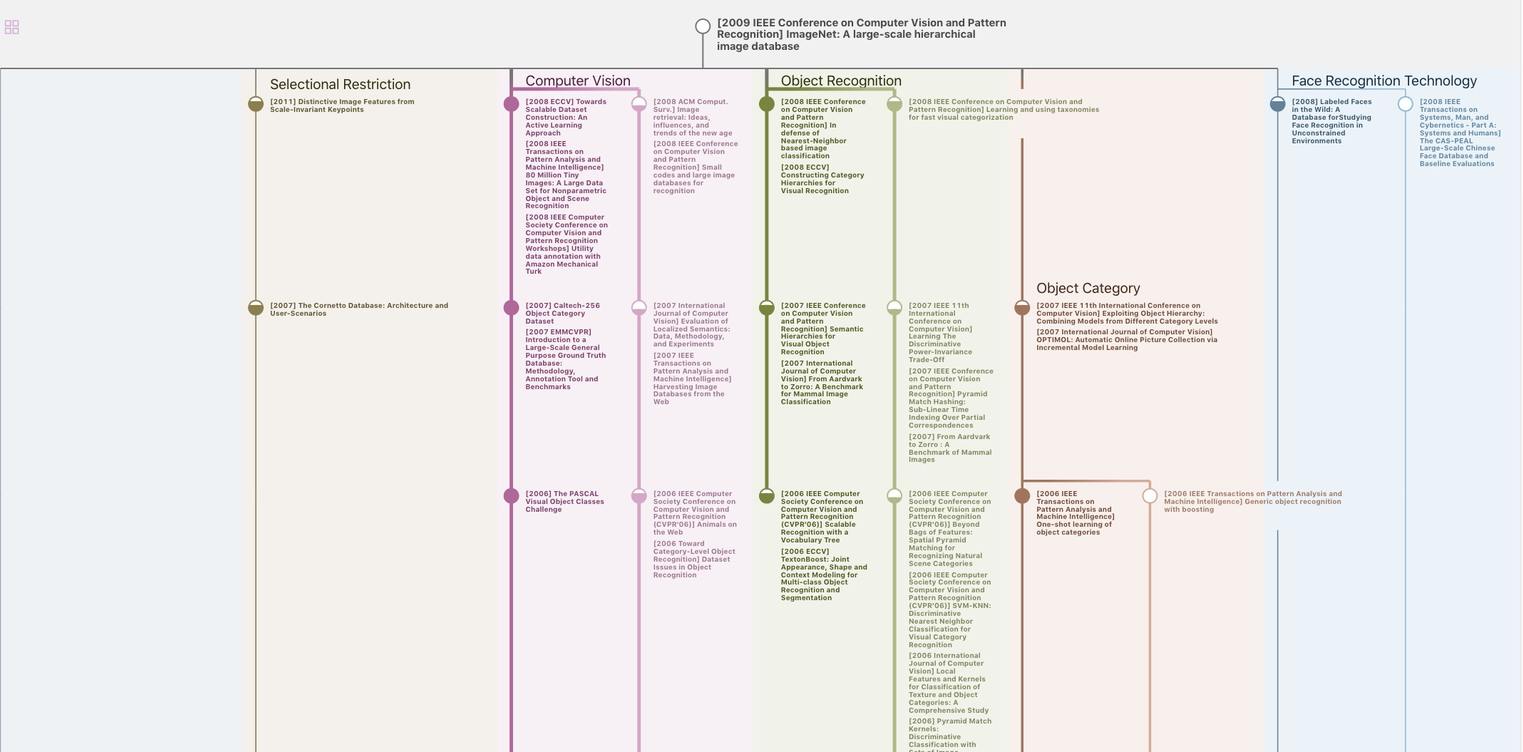
生成溯源树,研究论文发展脉络
Chat Paper
正在生成论文摘要