Learning Invariances in Neural Networks
arXiv (Cornell University)(2020)
摘要
Invariances to translations have imbued convolutional neural networks with powerful generalization properties. However, we often do not know a priori what invariances are present in the data, or to what extent a model should be invariant to a given symmetry group. We show how to \emph{learn} invariances and equivariances by parameterizing a distribution over augmentations and optimizing the training loss simultaneously with respect to the network parameters and augmentation parameters. With this simple procedure we can recover the correct set and extent of invariances on image classification, regression, segmentation, and molecular property prediction from a large space of augmentations, on training data alone.
更多查看译文
关键词
invariances,learning,networks
AI 理解论文
溯源树
样例
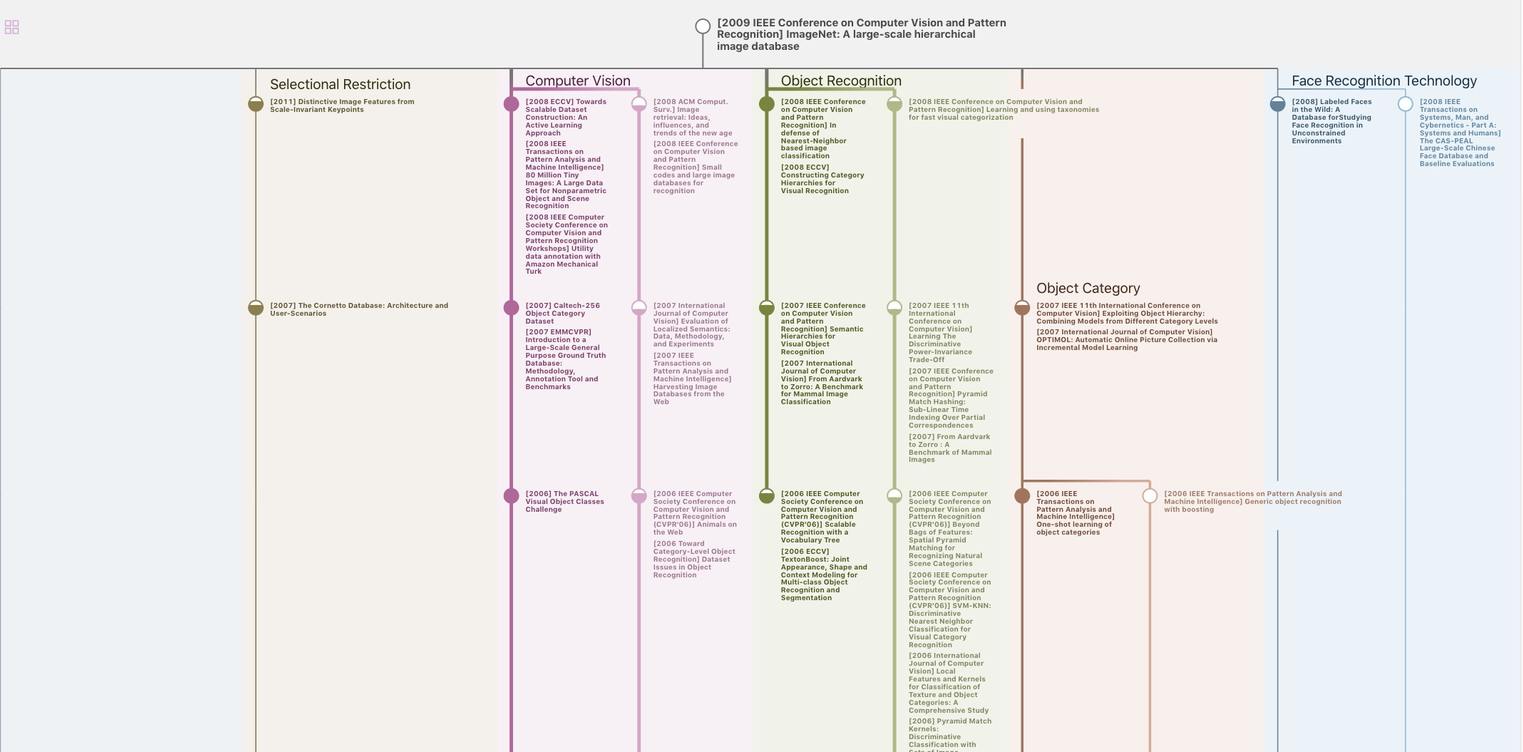
生成溯源树,研究论文发展脉络
Chat Paper
正在生成论文摘要