MimicDiffusion: Purifying Adversarial Perturbation via Mimicking Clean Diffusion Model
CoRR(2023)
摘要
Deep neural networks (DNNs) are vulnerable to adversarial perturbation, where
an imperceptible perturbation is added to the image that can fool the DNNs.
Diffusion-based adversarial purification focuses on using the diffusion model
to generate a clean image against such adversarial attacks. Unfortunately, the
generative process of the diffusion model is also inevitably affected by
adversarial perturbation since the diffusion model is also a deep network where
its input has adversarial perturbation. In this work, we propose
MimicDiffusion, a new diffusion-based adversarial purification technique, that
directly approximates the generative process of the diffusion model with the
clean image as input. Concretely, we analyze the differences between the guided
terms using the clean image and the adversarial sample. After that, we first
implement MimicDiffusion based on Manhattan distance. Then, we propose two
guidance to purify the adversarial perturbation and approximate the clean
diffusion model. Extensive experiments on three image datasets including
CIFAR-10, CIFAR-100, and ImageNet with three classifier backbones including
WideResNet-70-16, WideResNet-28-10, and ResNet50 demonstrate that
MimicDiffusion significantly performs better than the state-of-the-art
baselines. On CIFAR-10, CIFAR-100, and ImageNet, it achieves 92.67\%, 61.35\%,
and 61.53\% average robust accuracy, which are 18.49\%, 13.23\%, and 17.64\%
higher, respectively. The code is available in the supplementary material.
更多查看译文
AI 理解论文
溯源树
样例
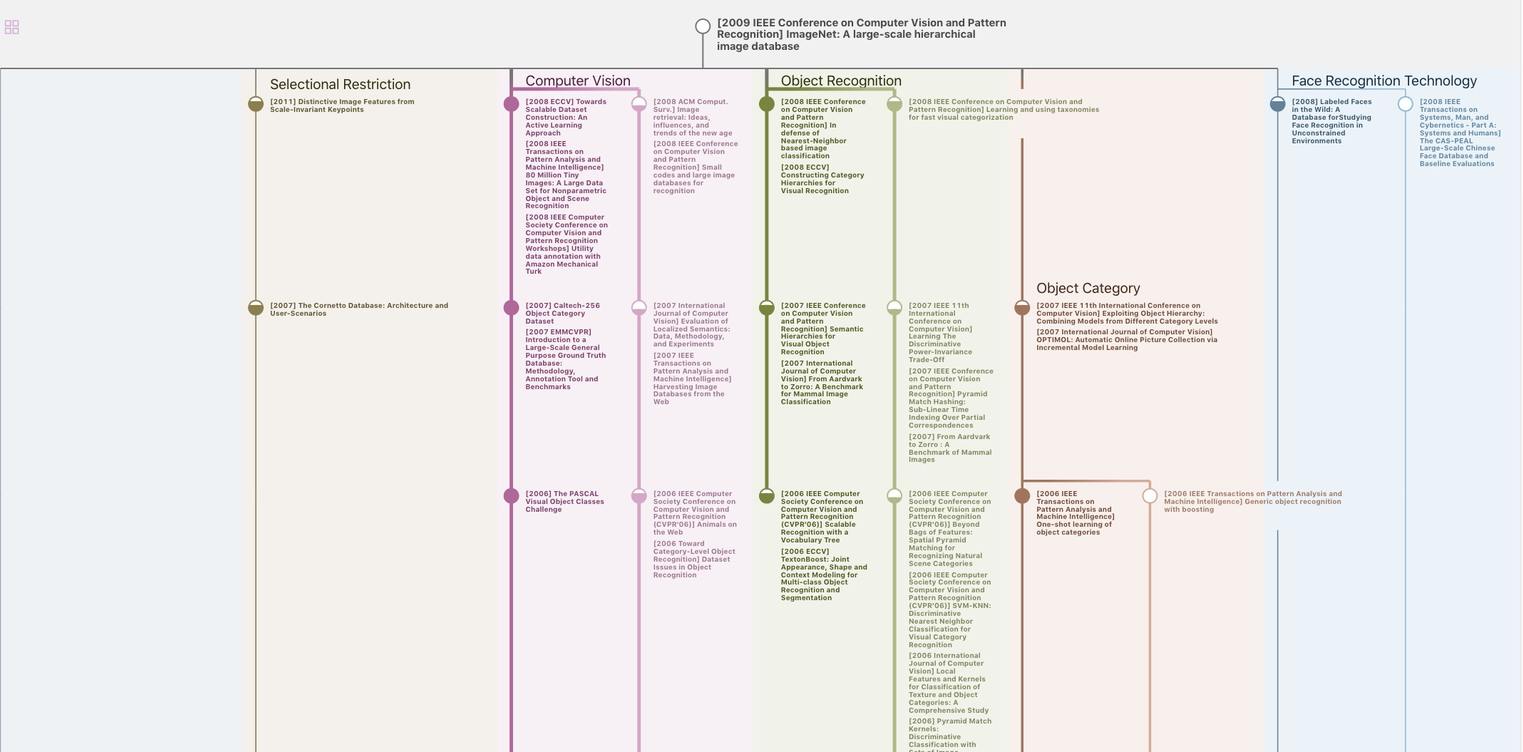
生成溯源树,研究论文发展脉络
Chat Paper
正在生成论文摘要