Detecting atomic-scale surface defects in STM of TMDs with ensemble deep learning
MRS Advances(2024)
摘要
Atomic-scale defect detection is shown in scanning tunneling microscopy images (STM) of single-crystal WSe2 using an ensemble of U-Net-like convolutional neural networks. Standard deep learning test metrics indicated good detection performance with an average F1 score of 0.8 and demonstrated ensemble generalization to conductive atomic force microscopy images of WSe2 and STM images of MoSe2. Defect coordinates were automatically extracted from defect detections maps showing that STM image analysis enhanced by machine learning can be used to dramatically increase sample characterization throughput.
更多查看译文
AI 理解论文
溯源树
样例
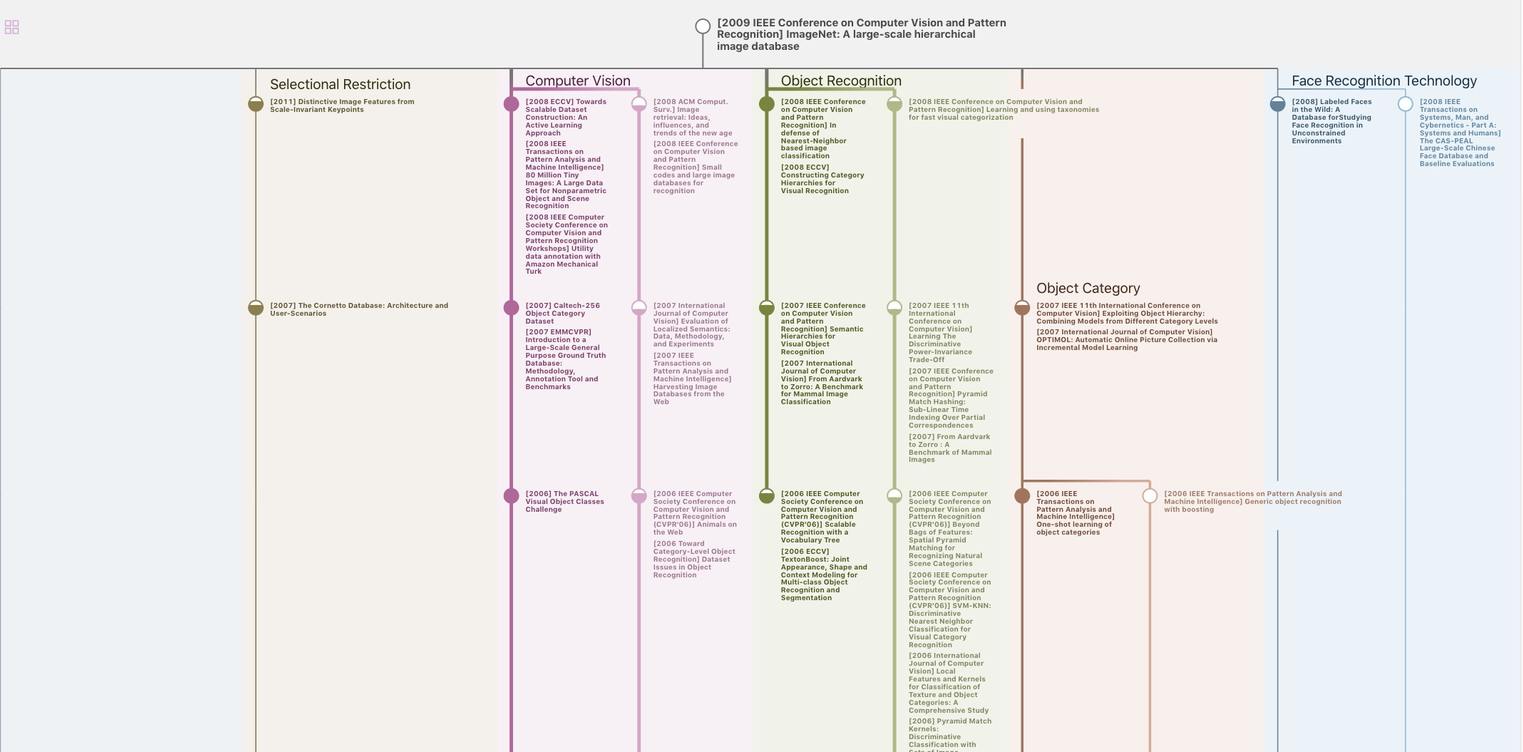
生成溯源树,研究论文发展脉络
Chat Paper
正在生成论文摘要