Short-Term Power Load Forecasting Using MOGOA and ConvBiLSTM During COVID-19 Pandemic
2023 International Conference on Power System Technology (PowerCon)(2023)
摘要
Due to the high restrictions of the global COVID-19 on society, economy and production, the electrical supply-demand balance is facing a great challenge. This paper proposes a short-term load forecasting (STLF) method based on multi-factor fusion during the COVID-19. After fully analyzing and screening the nonlinear coupling interactions among cross-domain weather and public health safety features, a convolutional bi-directional long short-term memory neural network (ConvBiLSTM) is developed based on the convolutional and bidirectional loop enhancements of long short-term memory (LSTM). To avoid early termination of training the global optimal solution, multi-objective grasshopper optimization algorithm (MOGOA) is adopted to select the inherent hyperparameters of the forecasting network. Attention mechanism is further introduced to enhance the learning ability of the key features in temporal data, improving the forecasting accuracy and robustness of ConvBiLSTM. Comparisons on cross-domain COVID-19 dataset over classical methods are performed to verify its effective and superior performances.
更多查看译文
关键词
Load forecasting,coronavirus disease,cross-domain
AI 理解论文
溯源树
样例
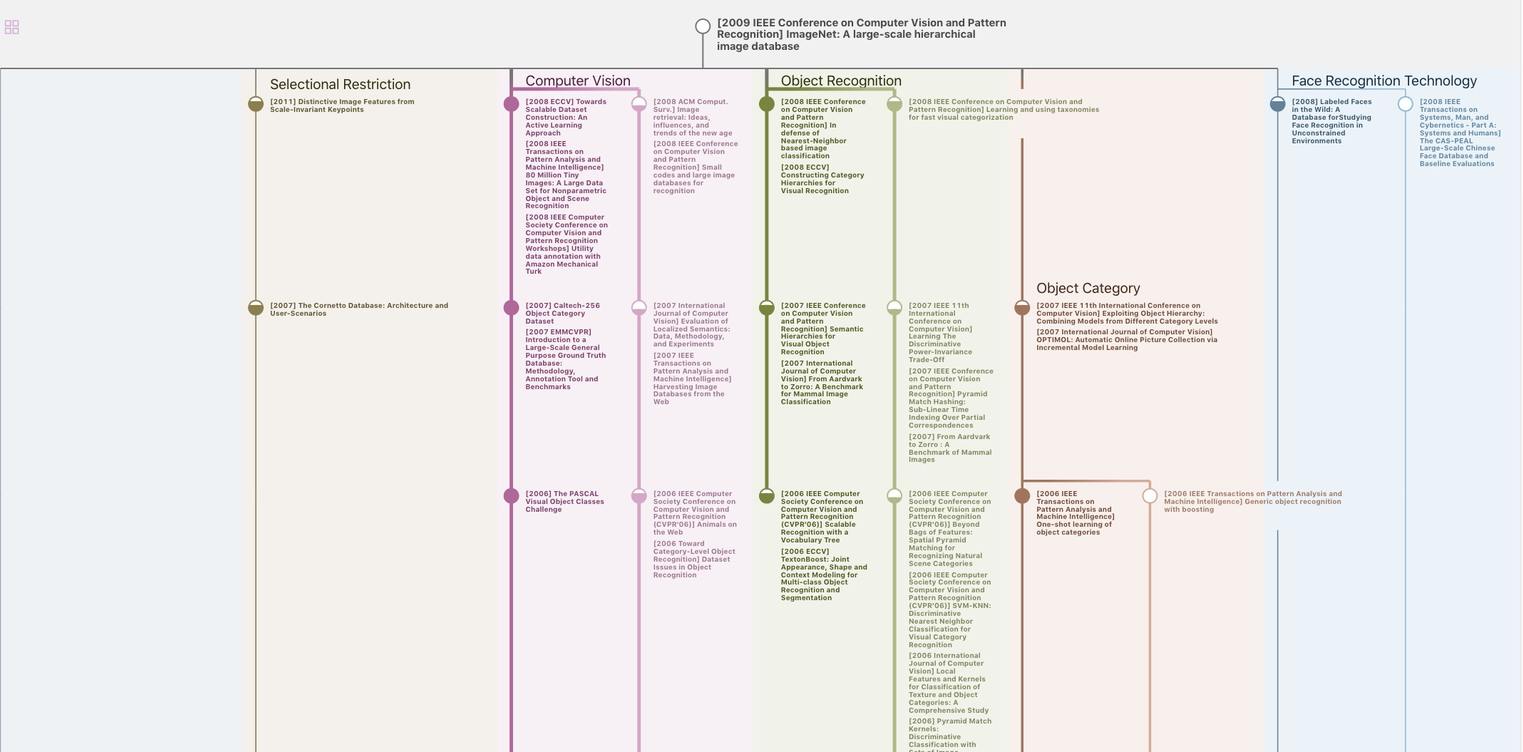
生成溯源树,研究论文发展脉络
Chat Paper
正在生成论文摘要