CLAPS: Curriculum Learning-based Adaptive Bitrate and Preloading for Short video streaming
2023 IEEE 25TH INTERNATIONAL WORKSHOP ON MULTIMEDIA SIGNAL PROCESSING, MMSP(2023)
摘要
To provide high user QoE while maintaining low bandwidth waste, it is important to design adaptive bitrate and preloading algorithms for short video streaming. Current solutions either have relatively low performance, as observed in heuristic algorithms, or suffer the problem of poor generalization, as seen in deep reinforcement learning (DRL)-based algorithms. To address this issue, we propose CLAPS, a curriculum learning-based DRL model that enhances the generalization of the DRL model across a wide range of data, while ensuring high performance. CLAPS introduces a comprehensive metric that measures the curriculum difficulty by combing the performance gap between an existing heuristic algorithm and the DRL model with the prediction error of network bandwidth. Moreover, we design a training scheduler to control sampling proportion based on Markov transition probabilities to address the model forgetting problem. Extensive evaluations using real video datasets and network traces including 5G, 4G, and Wi-Fi demonstrate that CLAPS outperforms all the baseline algorithms. Specifically, CLAPS improves the overall performance by 10.04%-13.84% and the generalization by 22.52%-35.77% compared to the best-performing DRL baseline.
更多查看译文
关键词
short video streaming,curriculum learning,preloading,adaptive bitrate
AI 理解论文
溯源树
样例
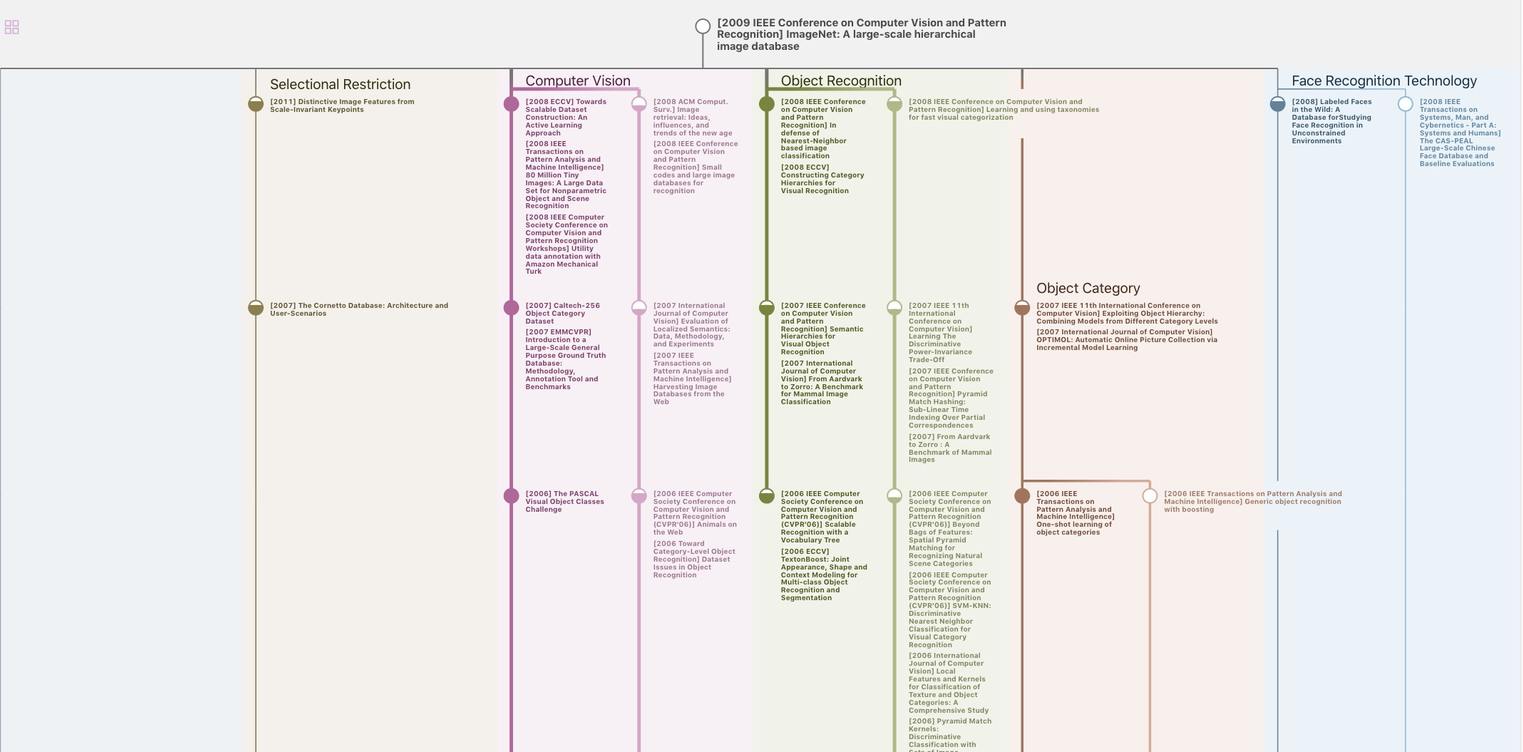
生成溯源树,研究论文发展脉络
Chat Paper
正在生成论文摘要