Field-informed Reinforcement Learning of Collective Tasks with Graph Neural Networks
2023 IEEE INTERNATIONAL CONFERENCE ON AUTONOMIC COMPUTING AND SELF-ORGANIZING SYSTEMS, ACSOS(2023)
摘要
Coordinating a multi-agent system of intelligent situated agents is a traditional research problem, impacted by the challenges posed by the very notion of distributed intelligence. These problems arise from agents acquiring information locally, sharing their knowledge, and acting accordingly in their environment to achieve a common, global goal. These issues are even more evident in large-scale collective adaptive systems, where agent interactions are necessarily proximity-based, thus making the emergence of controlled global collective behaviour harder. In this context, two main approaches have been proposed for creating distributed controllers out of macro-level task/goal descriptions: manual design, in which programmers build the controllers directly, and automatic design, which involves synthesizing programs using machine learning methods. In this paper, we consider a new hybrid approach called Field-Informed reinforcement learning (FIRL). We utilise manually designed computational fields (globally distributed data structures) to manage global agent coordination. Then, using Deep Q-learning in combination with Graph Neural Networks we enable the agents to learn the necessary local behaviour automatically to solve collective tasks, relying on those fields through local perception. We demonstrate the effectiveness of this new approach in simulated use cases where tracking and covering tasks for swarm robotics are successfully solved.
更多查看译文
关键词
Aggregate Computing,Graph Neural Networks,Cyber-Physical Swarms,Many Agent Reinforcement Learning
AI 理解论文
溯源树
样例
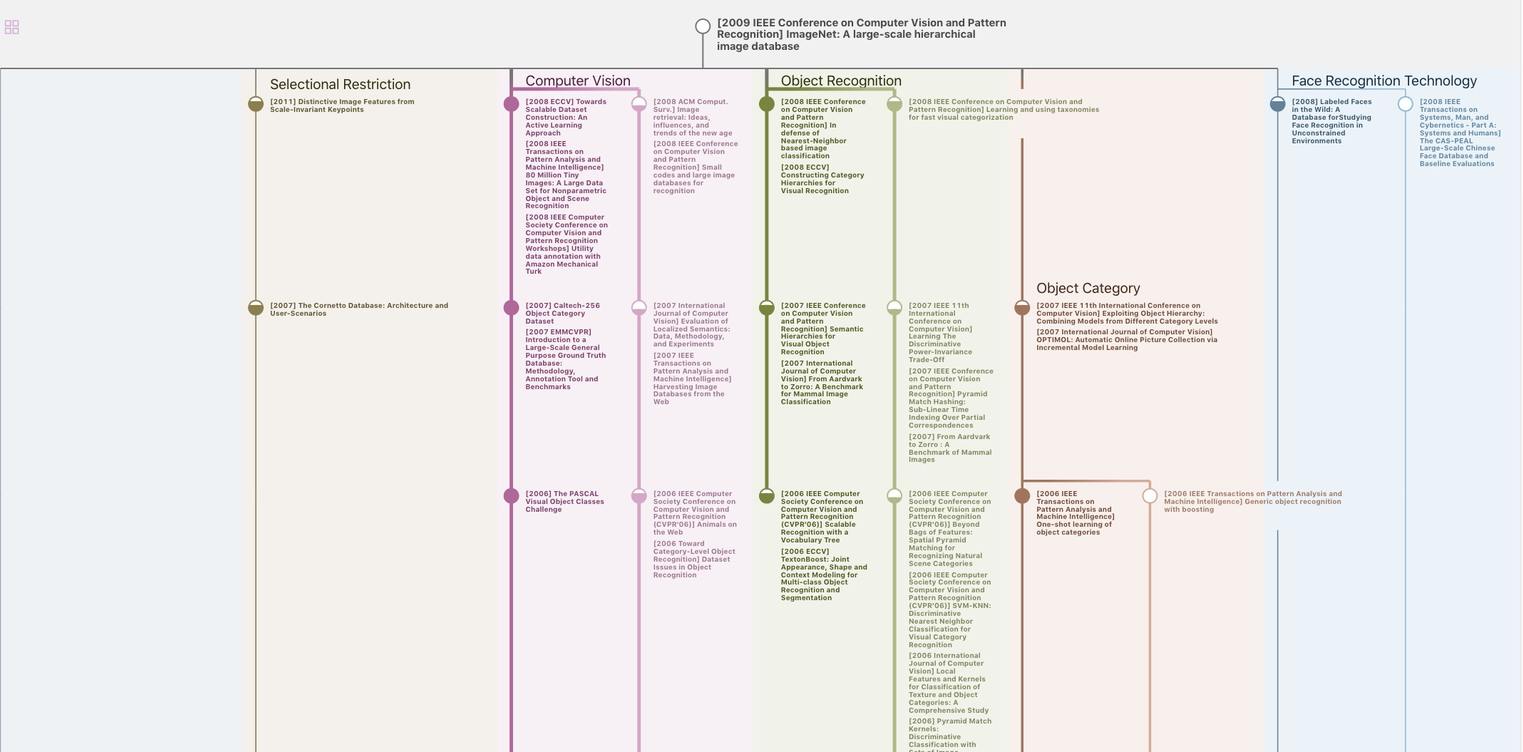
生成溯源树,研究论文发展脉络
Chat Paper
正在生成论文摘要