Deep Unsupervised Learning for Optimization With Box and Monotone Matrix Based Polytope Constraints: A Case-Study of D2D Wireless Networks
IEEE WIRELESS COMMUNICATIONS LETTERS(2023)
摘要
In this letter, we consider an optimization problem with box constraints coupled with polytope constraints. The existing deep learning methodologies for solving such constrained optimization problems are incapable to ensure feasible solution, require a resource-intensive projection step, or involve iterations. To this end, we propose a novel and efficient deep unsupervised learning (DUL)-based approach that minimizes the loss function while guaranteeing satisfaction of both box and polytope constraints involving a monotone matrix. In particular, we utilize properties of a monotone matrix to transform the original constrained optimization problem into an equivalent problem which can easily be optimized using DUL. We have conducted a performance comparison between our proposed approach and existing DUL-based benchmarks considering a device-to-device (D2D) wireless network. Simulation results have shown that our proposed scheme outperforms the benchmark schemes in terms of both constraint satisfaction probability and achievable average sum-rate.
更多查看译文
关键词
Box constraints,convex polytope,deep unsupervised learning,monotone matrix,neural network,power allocation
AI 理解论文
溯源树
样例
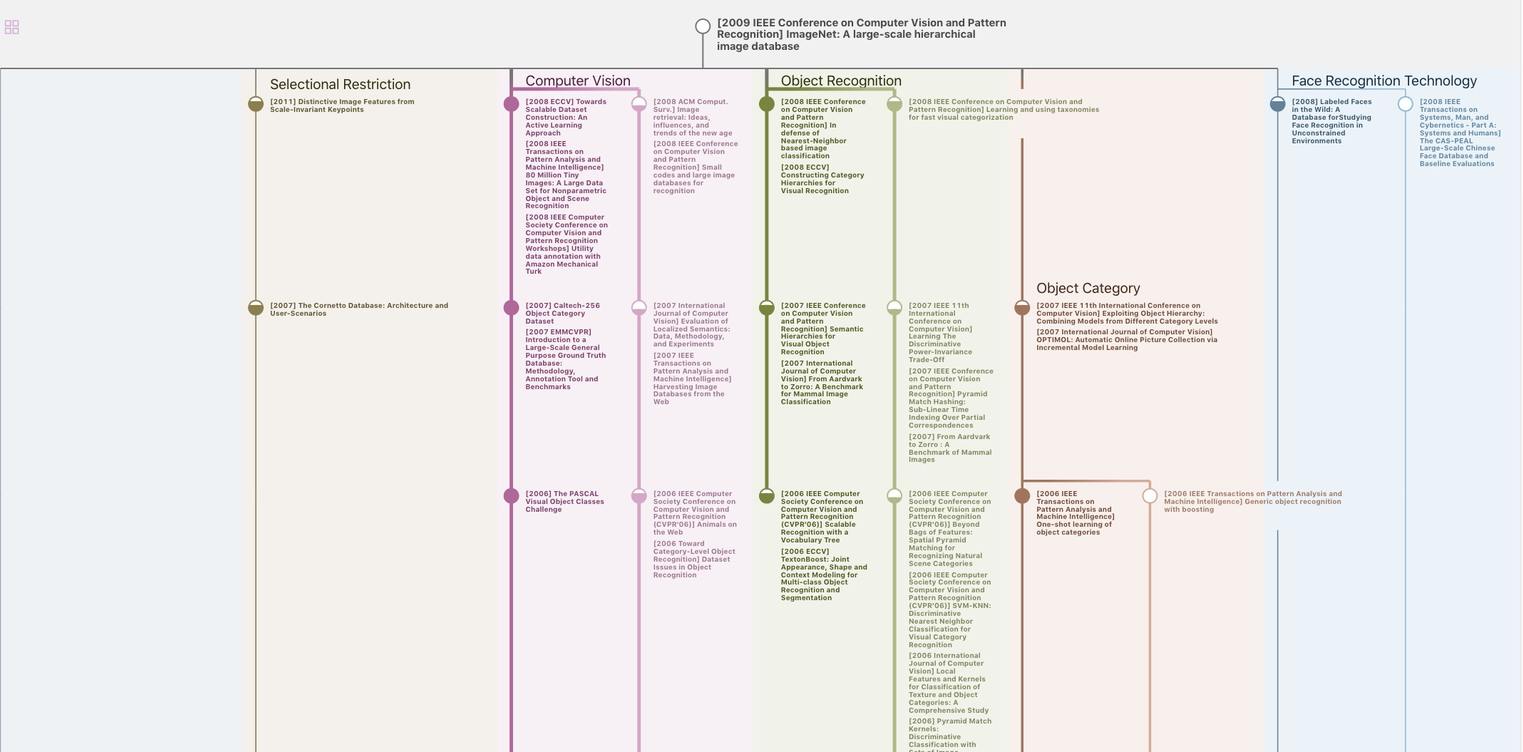
生成溯源树,研究论文发展脉络
Chat Paper
正在生成论文摘要