Improved Bayesian Learning Detectors for Uplink Grant-Free MIMO-NOMA
IEEE Wireless Communications Letters(2023)
摘要
Grant-free non-orthogonal multiple access (GF-NOMA) and multiple-input multiple-output (MIMO) techniques are key enablers for massive machine-type communications (mMTC) in 5G cellular Internet of Things. On the other hand, compressed sensing (CS) is widely accepted for multiuser detection, due to the sporadic traffic of mMTC. In this letter, we propose two Bayesian-based CS algorithms for uplink grant-free MIMO-NOMA. Exploiting the iterative expectation maximization (EM) and maximum ratio combining techniques, the spatially enhanced sparse Bayesian learning (SE-SBL) algorithm is developed to alternately update hyperparameter values and combined observation signals. Furthermore, we embed the generalized approximate message passing technique into the SE-SBL and propose a low-complexity computational approach. In particular, the aforementioned Bayesian algorithms do not require any prior knowledge of user activity level and noise power. Simulation results show that the proposed Bayesian methods exhibit a superior performance gain over the state-of-the-art.
更多查看译文
关键词
Matching pursuit algorithms,Massive machine-type communications,grantfree,non-orthogonal multiple access,multiple-input multipleoutput,Bayesian compressed sensing
AI 理解论文
溯源树
样例
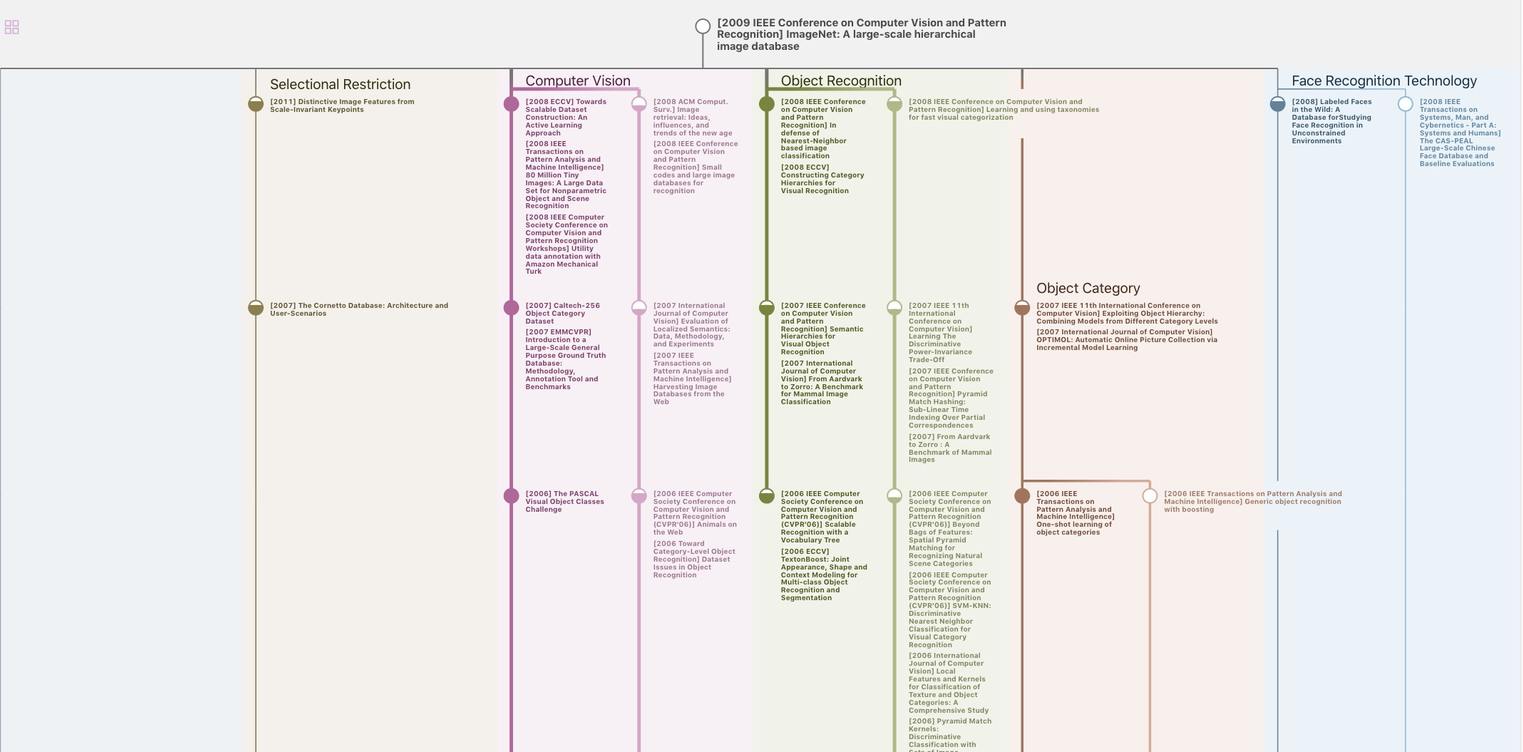
生成溯源树,研究论文发展脉络
Chat Paper
正在生成论文摘要