Neurophysiological correlates of short-term recognition of sounds: Insights from magnetoencephalography
biorxiv(2023)
摘要
Understanding the brain's dynamic retrieval and updating of encoded information is a key focus in memory research. This study employed a same versus different auditory paradigm to investigate short-term auditory recognition within a predictive coding (PC) framework, concerning the perceptual interplay between experience-informed predictions and incoming sensory information. Using magnetoencephalography (MEG), we captured the neurophysiological correlates associated with a single-sound, short-term memory task. Twenty-six healthy participants were tasked with recognizing whether presented sounds were same or different compared to strings of standard stimuli. To prompt conscious memory retention, a white noise interlude separated these sounds from the standards. MEG sensor-level results revealed that recognition of same sounds elicited two significantly stronger negative components of the event-related field compared to different sounds. The first one was the N1, peaking 100ms post-sound onset, while the second one corresponded to a slower negative component arising between 300 and 600ms after sound onset. This effect was observed in several significant clusters of MEG sensors, especially temporal and parietal regions of the scalp. Conversely, different sounds produced scattered and smaller clusters of stronger activity than same sounds, peaking later than 600ms after sound onset. Source reconstruction using beamforming algorithms revealed the involvement of auditory cortices, hippocampus, and cingulate gyrus in both conditions. Overall, the results are coherent with PC principles and previous results on the brain mechanisms underlying auditory recognition, highlighting the relevance of early and later negative brain responses for successful prediction of previously listened sounds in the context of conscious short-term memory.
### Competing Interest Statement
The authors have declared no competing interest.
更多查看译文
AI 理解论文
溯源树
样例
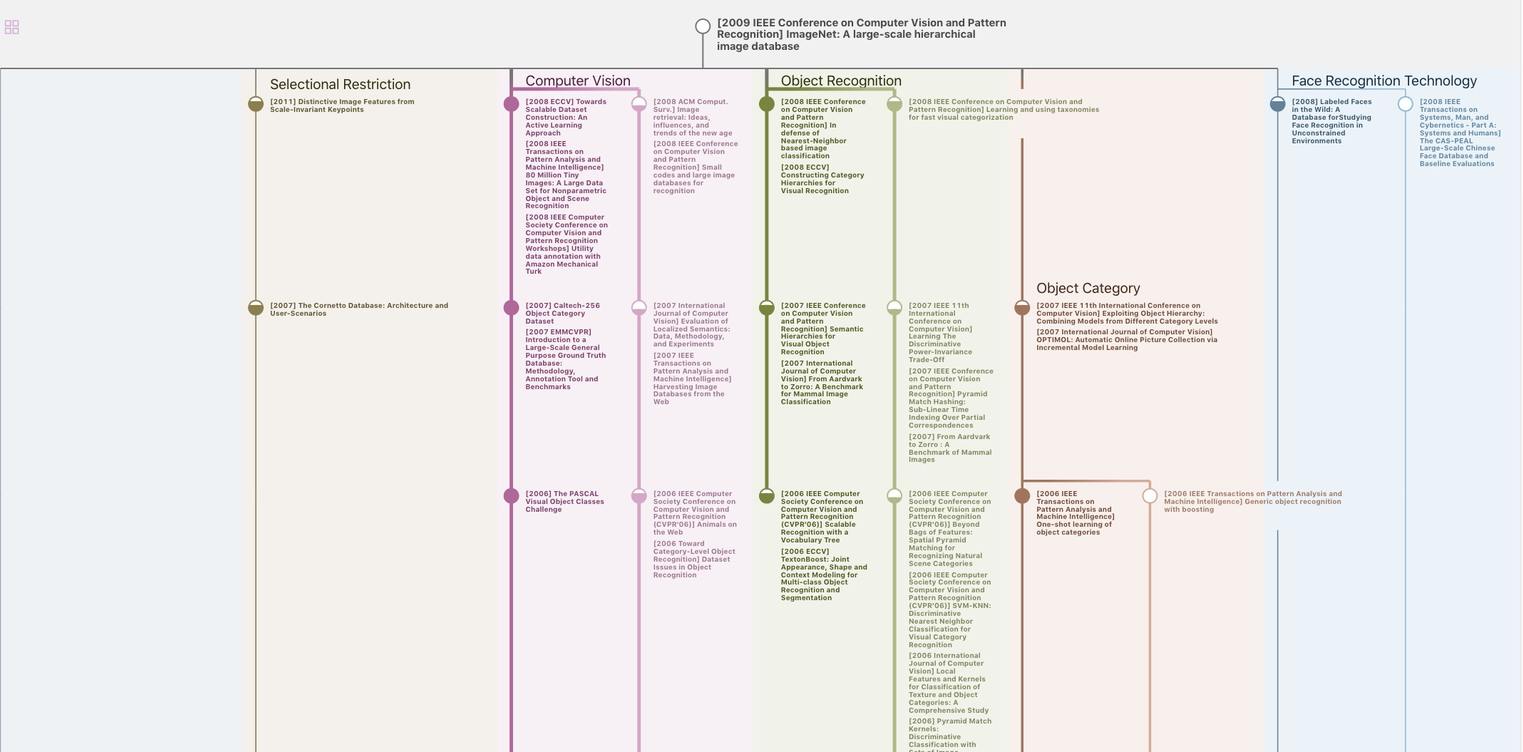
生成溯源树,研究论文发展脉络
Chat Paper
正在生成论文摘要