Surface Defect Detection for No-Service Rails With Skeleton-Aware Accurate and Fast Network
IEEE TRANSACTIONS ON INDUSTRIAL INFORMATICS(2023)
摘要
Vision-based surface defect detection for no-service rails provides a fast and effective way to monitor product quality. However, most of the existing surface defect detection algorithms ${1)}$ prioritize enhancing detection accuracy at the expense of processing speed and ${2)}$ lack compatibility with various input image types [RGB images or RGB-depth (RGBD) images]. To address these issues, we propose a skeleton-aware accurate and fast network for pixelwise surface defect detection. The skeleton is first used in defect detection tasks to aid in locating defects and to guide the growth of more accurate defect predictions by utilizing its continuity. In addition, a simple and efficient feature fusion module, information prominence fusion, is proposed for cross-layer feature representation. A compatibility module, depth-aware fusion, is devised to introduce and integrate depth information. Experiments have proven that our network can achieve excellent detection results while its detection speed can reach 537.2 fps for RGB detection and 423.8 fps for RGBD detection at 20 input batch sizes. Generalizability experiments verify that our network still performs competitively on the surface defect detection of strip steel and salient object detection of RGB and RGBD natural images.
更多查看译文
关键词
Accurate and fast detection,rail surface defects,salient object detection (SOD),surface defect detection
AI 理解论文
溯源树
样例
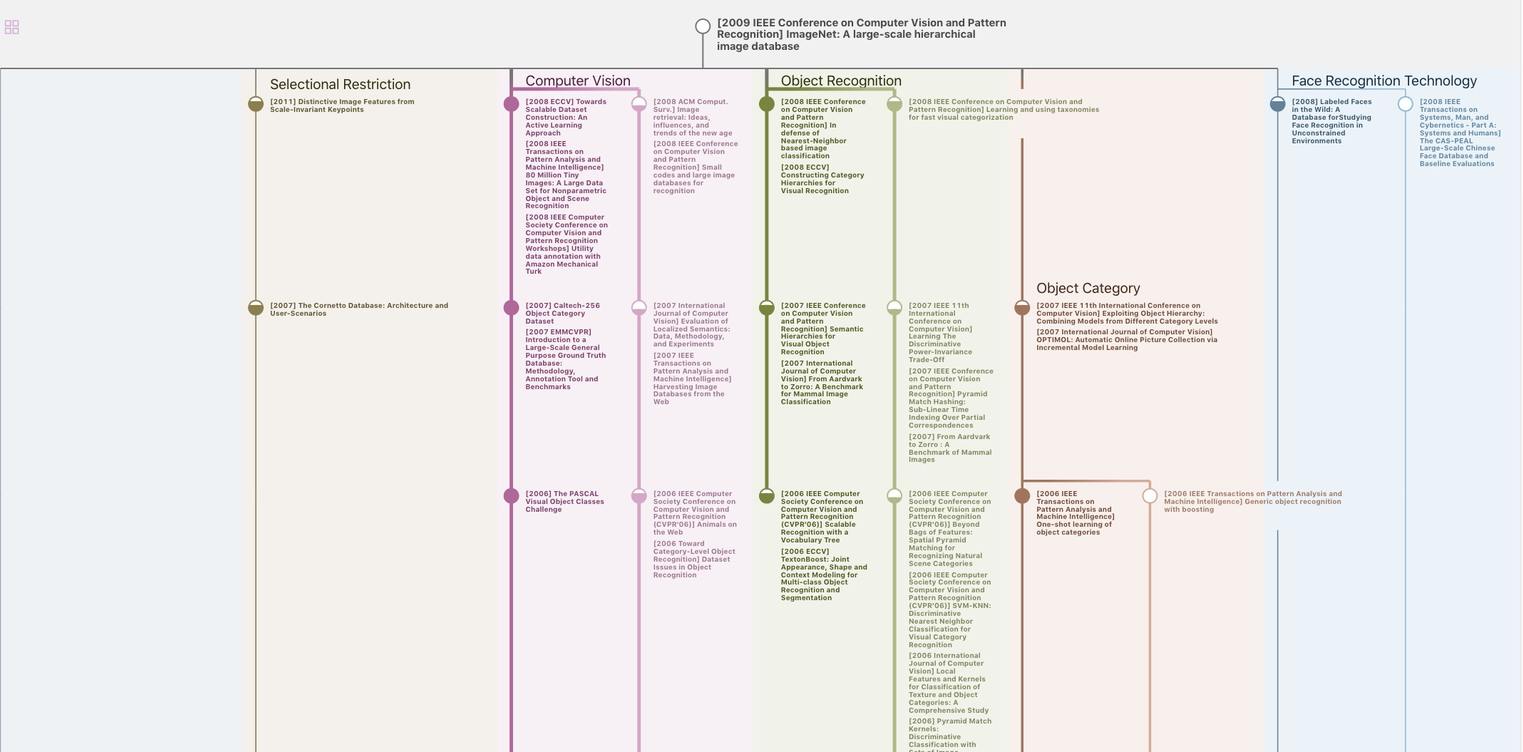
生成溯源树,研究论文发展脉络
Chat Paper
正在生成论文摘要