Variable transformation strategies in forest biomass modeling
SCIENTIA FORESTALIS(2023)
摘要
In linear regression, residual normality and homoscedasticity assumptions must be satisfied for correct statistical inferences, which are commonly ignored in forest biomass modeling. In these cases, variable transformations can be applied as corrective measures, whose knowledge represents a scientific gap in forest science. This study aimed to evaluate data transformation strategies in linear regression models to predict total forest biomass and its components. Data for fitting linear regression models were sampled in native stands of Mimosa scrabella in Southern Brazil. Trees were sampled to determine the mass of stem, branch, leaf, bark, and root components. Data transformations were assessed to fit biomass with the diameter as a predictor. Models that simultaneously satisfied the residual normality and homoscedasticity assumptions were statistically evaluated. Modeling of total biomass and its components resulted in improved accuracy by applying variable transformations. The Root-Root transformation was indicated for predicting total and stem biomass. In addition, Log-Log transformation was recommended for estimating branch and bark biomass, as well as Manly for leaf biomass and Dual power for root biomass.
更多查看译文
关键词
Allometry, Native forest, Statistical assumptions, Homoscedasticity, Residual normality
AI 理解论文
溯源树
样例
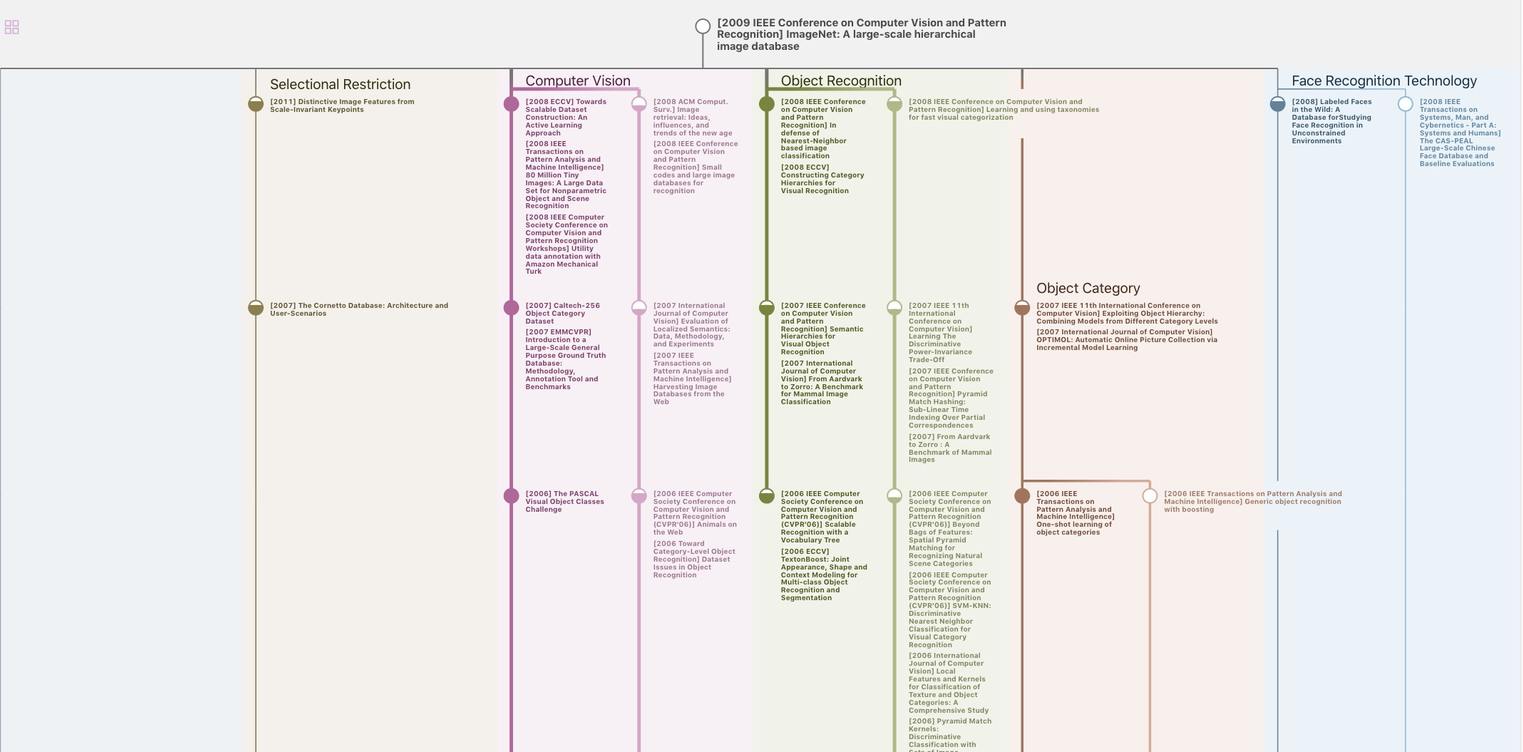
生成溯源树,研究论文发展脉络
Chat Paper
正在生成论文摘要