Rheology-informed hierarchical machine learning model for the prediction of printing resolution in extrusion-based bioprinting
INTERNATIONAL JOURNAL OF BIOPRINTING(2023)
摘要
In this study, a rheology-informed hierarchical machine learning (RIHML) model was developed to improve the prediction accuracy of the printing resolution of constructs fabricated by extrusion-based bioprinting. Specifically, the RIHML model, as well as conventional models such as the concentration-dependent model and printing parameter-dependent model, was trained and tested using a small dataset of bioink properties and printing parameters. Interestingly, the results showed that the RIHML model exhibited the lowest error percentage in predicting the printing resolution for different printing parameters such as nozzle velocities and pressures, as well as for different concentrations of the bioink constituents. Besides, the RIHML model could predict the printing resolution with reasonably low errors even when using a new material added to the alginate-based bioink, which is a challenging task for conventional models. Overall, the results indicate that the RIHML model can be a useful tool to predict the printing resolution of extrusion-based bioprinting, and it is versatile and expandable compared to conventional models since the RIHML model can easily generalize and embrace new data.
更多查看译文
关键词
hierarchical machine learning model,machine learning,rheology-informed,extrusion-based
AI 理解论文
溯源树
样例
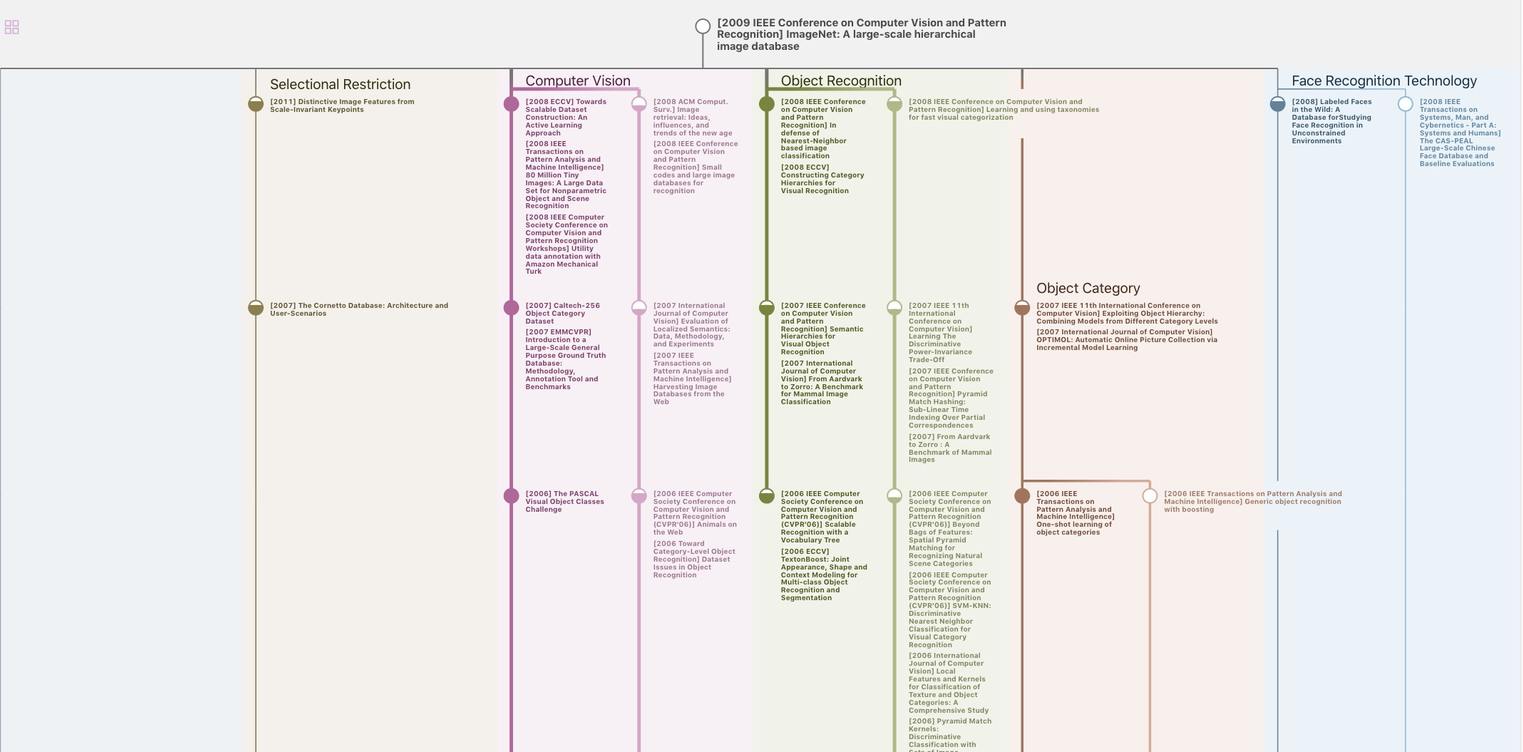
生成溯源树,研究论文发展脉络
Chat Paper
正在生成论文摘要