TransVG plus plus : End-to-End Visual Grounding With Language Conditioned Vision Transformer
IEEE TRANSACTIONS ON PATTERN ANALYSIS AND MACHINE INTELLIGENCE(2023)
摘要
In this work, we explore neat yet effective Transformer-based frameworks for visual grounding. The previous methods generally address the core problem of visual grounding, i.e., multi-modal fusion and reasoning, with manually-designed mechanisms. Such heuristic designs are not only complicated but also make models easily overfit specific data distributions. To avoid this, we first propose TransVG, which establishes multi-modal correspondences by Transformers and localizes referred regions by directly regressing box coordinates. We empirically show that complicated fusion modules can be replaced by a simple stack of Transformer encoder layers with higher performance. However, the core fusion Transformer in TransVG is stand-alone against uni-modal encoders, and thus should be trained from scratch on limited visual grounding data, which makes it hard to be optimized and leads to sub-optimal performance. To this end, we further introduce TransVG++ to make two-fold improvements. For one thing, we upgrade our framework to a purely Transformer-based one by leveraging Vision Transformer (ViT) for vision feature encoding. For another, we devise Language Conditioned Vision Transformer that removes external fusion modules and reuses the uni-modal ViT for vision-language fusion at the intermediate layers. We conduct extensive experiments on five prevalent datasets, and report a series of state-of-the-art records.
更多查看译文
关键词
Transformers, Visualization, Grounding, Proposals, Pipelines, Convolutional neural networks, Cognition, Deep learning, transformer network, vision and language, visual grounding
AI 理解论文
溯源树
样例
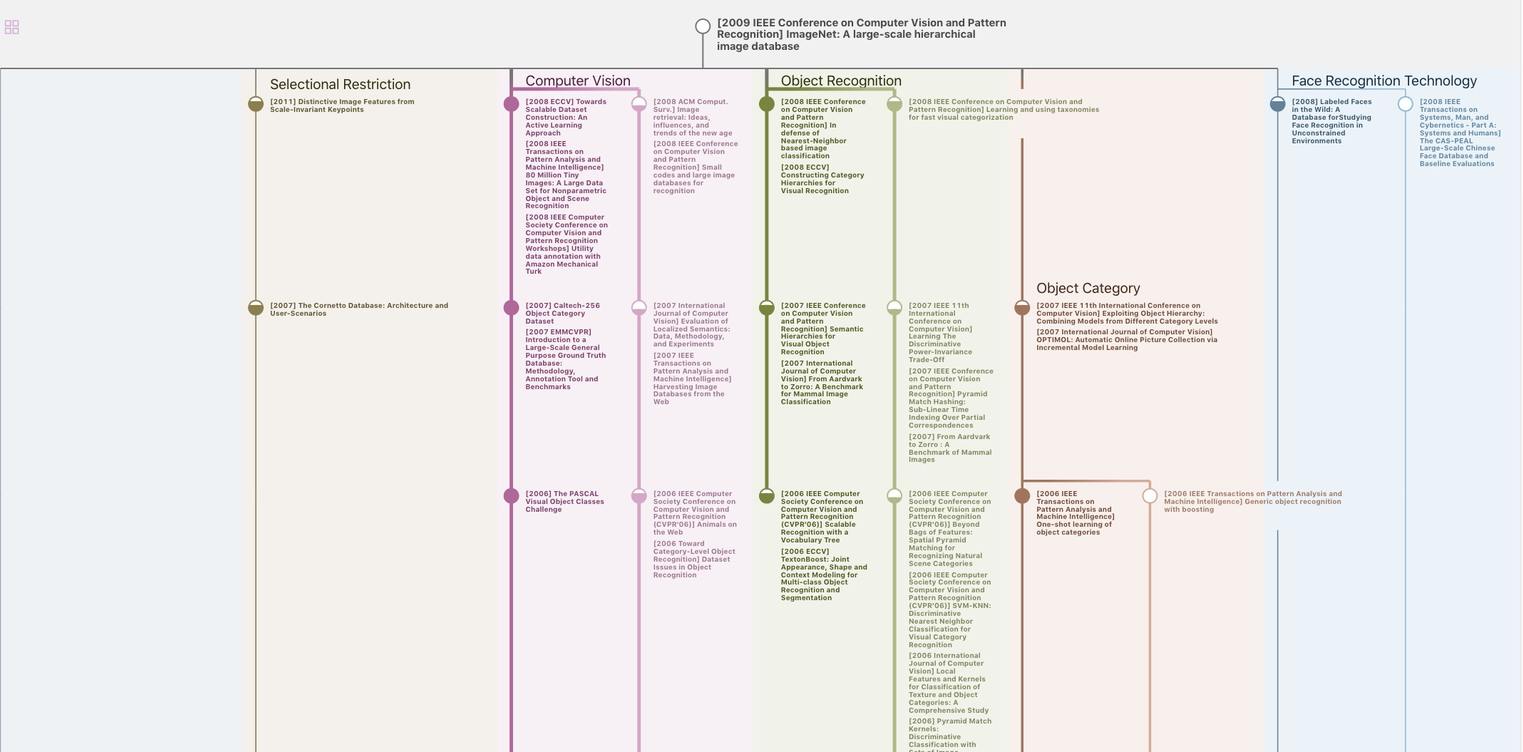
生成溯源树,研究论文发展脉络
Chat Paper
正在生成论文摘要