A Two-Level Adaptive Test Battery
JOURNAL OF EDUCATIONAL AND BEHAVIORAL STATISTICS(2023)
摘要
A test battery with two different levels of adaptation is presented: a within-subtest level for the selection of the items in the subtests and a between-subtest level to move from one subtest to the next. The battery runs on a two-level model consisting of a regular response model for each of the subtests extended with a second level for the joint distribution of their abilities. The presentation of the model is followed by an optimized MCMC algorithm to update the posterior distribution of each of its ability parameters, select the items to Bayesian optimality, and adaptively move from one subtest to the next. Thanks to extremely rapid convergence of the Markov chain and simple posterior calculations, the algorithm can be used in real-world applications without any noticeable latency. Finally, an empirical study with a battery of short diagnostic subtests is shown to yield score accuracies close to traditional one-level adaptive testing with subtests of double lengths.
更多查看译文
关键词
ability estimation,adaptive testing,Bayesian optimality,Gibbs sampler,item response models,MCMC algorithm
AI 理解论文
溯源树
样例
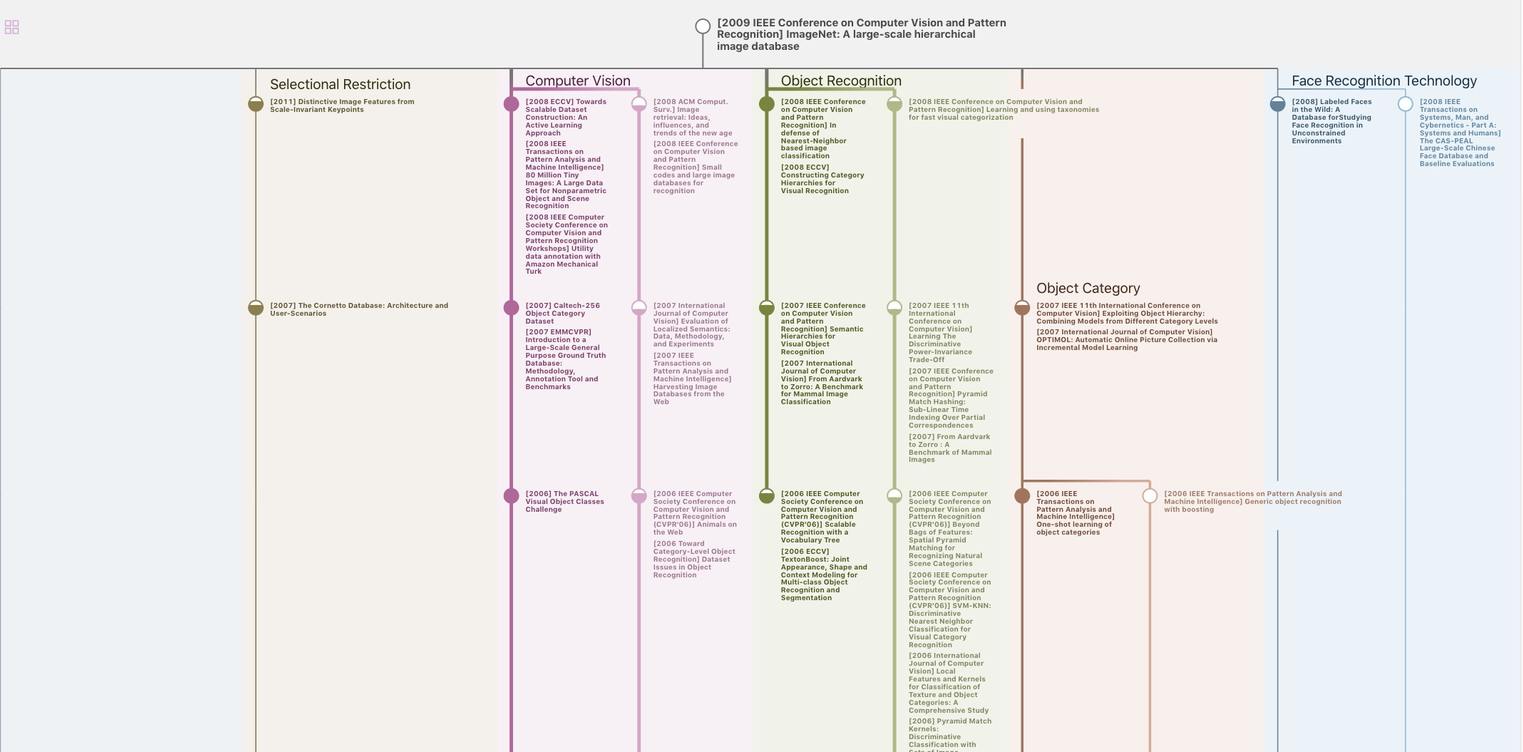
生成溯源树,研究论文发展脉络
Chat Paper
正在生成论文摘要