Intelligent Autonomous Vehicle Computation Using Deep Learning with Grasshopper Optimization
HUMAN-CENTRIC COMPUTING AND INFORMATION SCIENCES(2023)
摘要
Autonomous vehicles (AV) are in-demand future technologies for intelligent driving applications. The fast-decision-making techniques like artificial intelligence (AI) are required to train AV. Recently deep learning achieves tremendous results in fast computation and training models. Due to the sensing devices' data generation, AI has been used to process big data for making the appropriate decision in motion. Full automation system of AV can be achieved using AI techniques, and it is the recent research focus. In this paper, AI and deep learning-based approaches are used for AV in the applications such as vehicle detection, localization & mapping, and decision-making. With the large structural and variations of vehicle appearances, vehicle detection is a challenging task. In this paper, we proposed an efficient vehicle object detection method using a multi-task 2D deep convolution neural network (CNN) and a Cartesian product. Then the network loss is optimized with an evolutionary algorithm called grasshopper optimization. In this paper, localization and mapping are performed using the Kalman filter with fast correlation-based elephant herding optimization. The Kalman filter is used here to address the limitation on the sensory unit. Decision-making on vehicle parking of AV is done using deep bagging CNN. This proposed system works better than the traditional AV approach.
更多查看译文
关键词
Autonomous Vehicles, Artificial Intelligence, Deep Learning, Convolutional Neural Network, Kalman Filter, Object Detection, Localization, Decision-Making
AI 理解论文
溯源树
样例
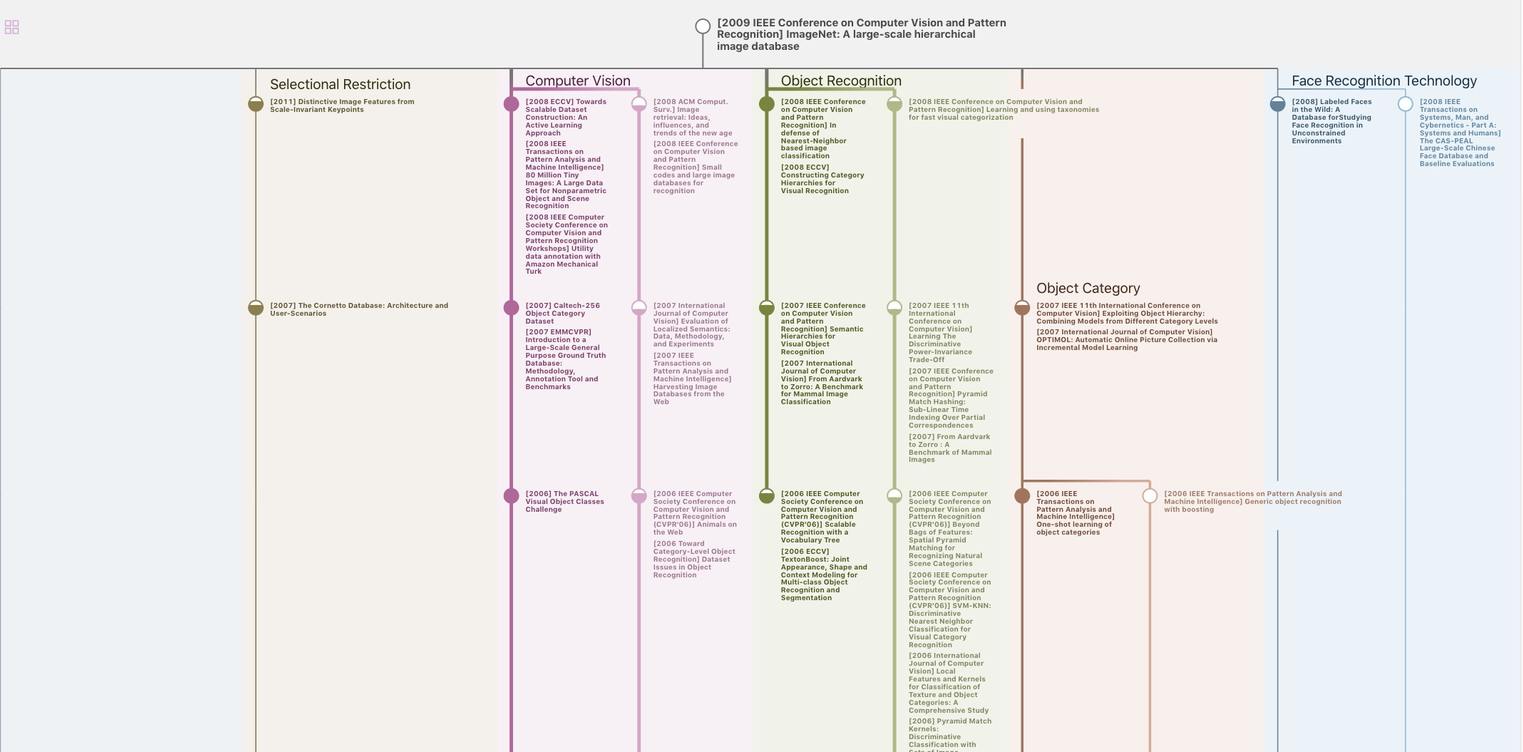
生成溯源树,研究论文发展脉络
Chat Paper
正在生成论文摘要