Optimized MPPT for Aero-generator System built on Autonomous Squirrel Cage Generators Using Feed-Forward Neural Network
INTERNATIONAL JOURNAL OF RENEWABLE ENERGY RESEARCH(2023)
摘要
The research on Maximum Power Point Tracking (MPPT) techniques for wind turbine installation (WTI) is an ongoing effort to improve the output power of wind systems. AI-based controllers, particularly Neural network controllers, are becoming popular choices for capturing maximum power from wind generators. However, obtaining accurate data for training and finetuning the Artificial Neural Network (ANN) model remains a significant challenge in establishing effective MPPT methods. Our study proposes a novel approach using feed-forward function neural networks (FF-NN) for MPPT in WTI based on Autonomous Squirrel Cage Generators (ASCGs). Our study contributes to the advancement of MPPT techniques in the wind energy industry by presenting a comprehensive comparative analysis of various MPPT techniques, including VSS-P&O, VSS-INC, OTC, GA, and GWO. The FF-NN approach maximizes MPPT by regulating the duty cycle and accurately tracking the maximum power point (MPP) without requiring knowledge of wind turbine power characteristics. The results of our simulations in the MATLAB/Simulink environment show that the FF-NN method performs better under diverse loads and environmental disturbances, sustains the ASCG's voltage build-up under severe loads, and has high responsiveness to noisy wind speeds. Moreover, our study highlights the improved performance metrics of using FF-NN, such as its lower complexity, easy maintenance, and better MPP tracking accuracy compared to the other MPPT techniques. The proposed approach using FF-NN is a novel and comprehensive solution that adds to the existing body of knowledge in the field of wind energy by presenting a new perspective for MPPT techniques in ASCG-based WTI.
更多查看译文
关键词
ASCG, MPPT, FF-NN, OTC, VSS-INC, VSS-P&O, GA, GWO
AI 理解论文
溯源树
样例
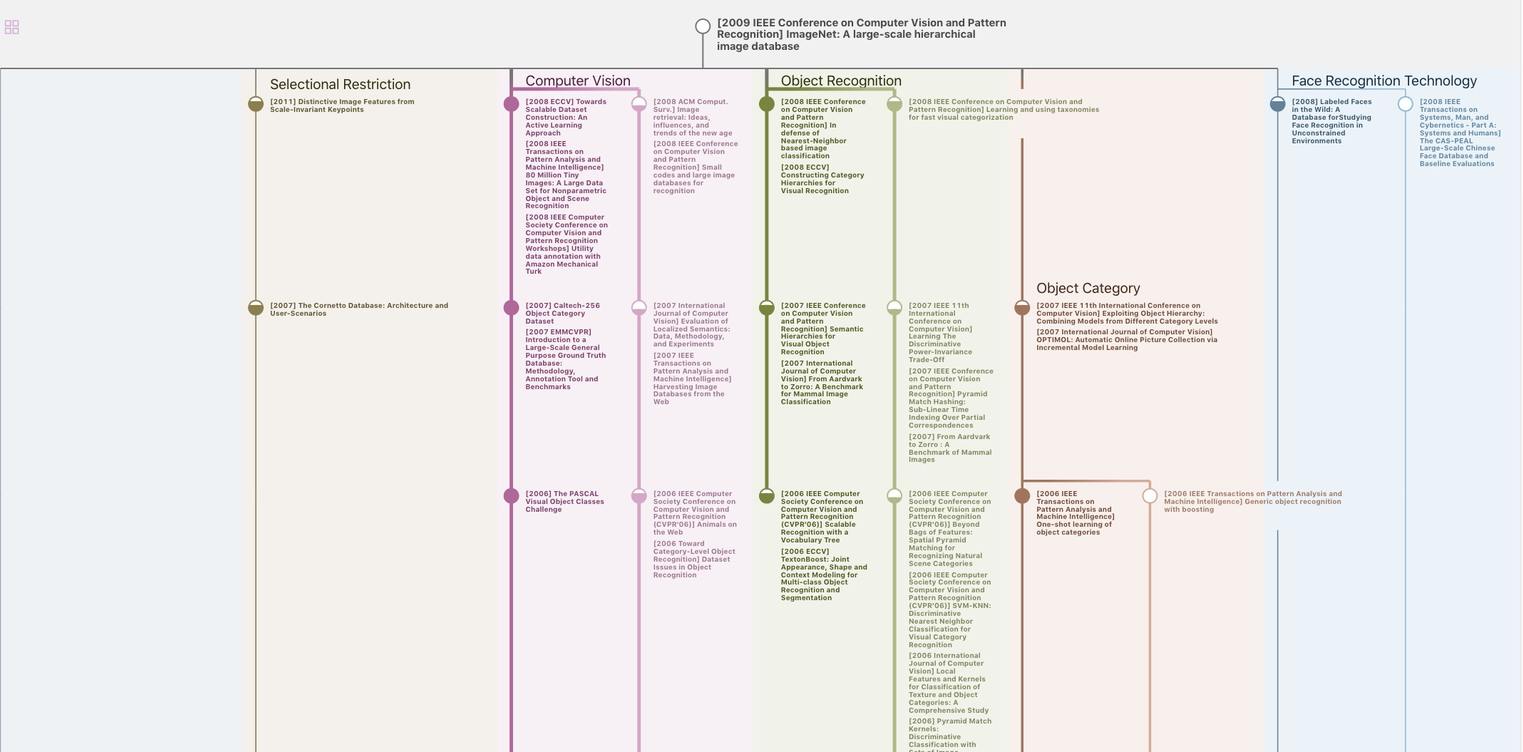
生成溯源树,研究论文发展脉络
Chat Paper
正在生成论文摘要