Back-propagating errors through artificial neural networks for variable selection
INTERNATIONAL JOURNAL OF WAVELETS MULTIRESOLUTION AND INFORMATION PROCESSING(2024)
摘要
Variable selection is one of the most important contents of machine learning research and a large number of variable selection methods have been proposed at present. From deep learning perspective, we propose a new variable selection method based on multilayer artificial neural networks in this paper. The method propagates errors gained form a trained network model through the network from output layer to input layer and the values gained by input layer give information about the relative contribution. The variables with big contribution value are selected, which we call neural inverse propagation (NIP). Specifically, after training a neural network, the gained errors between the actual network outputs and the desired outputs go through the network from top to down and arrive at the input layer. The absolute values gained by input units provide information about the relative importance (contribution) of input units, which is that the larger the value is, the more important the corresponding input variable is. We remove k variables corresponding to the first k minimum absolute value gained by input units to the predictive variables, to perform variable selection. The efficiency of the proposed method is illustrated through its application to classification and regression tasks on a number of synthetic datasets and a real-world image classification dataset.
更多查看译文
关键词
Artificial neural network,variable selection,regression,classification
AI 理解论文
溯源树
样例
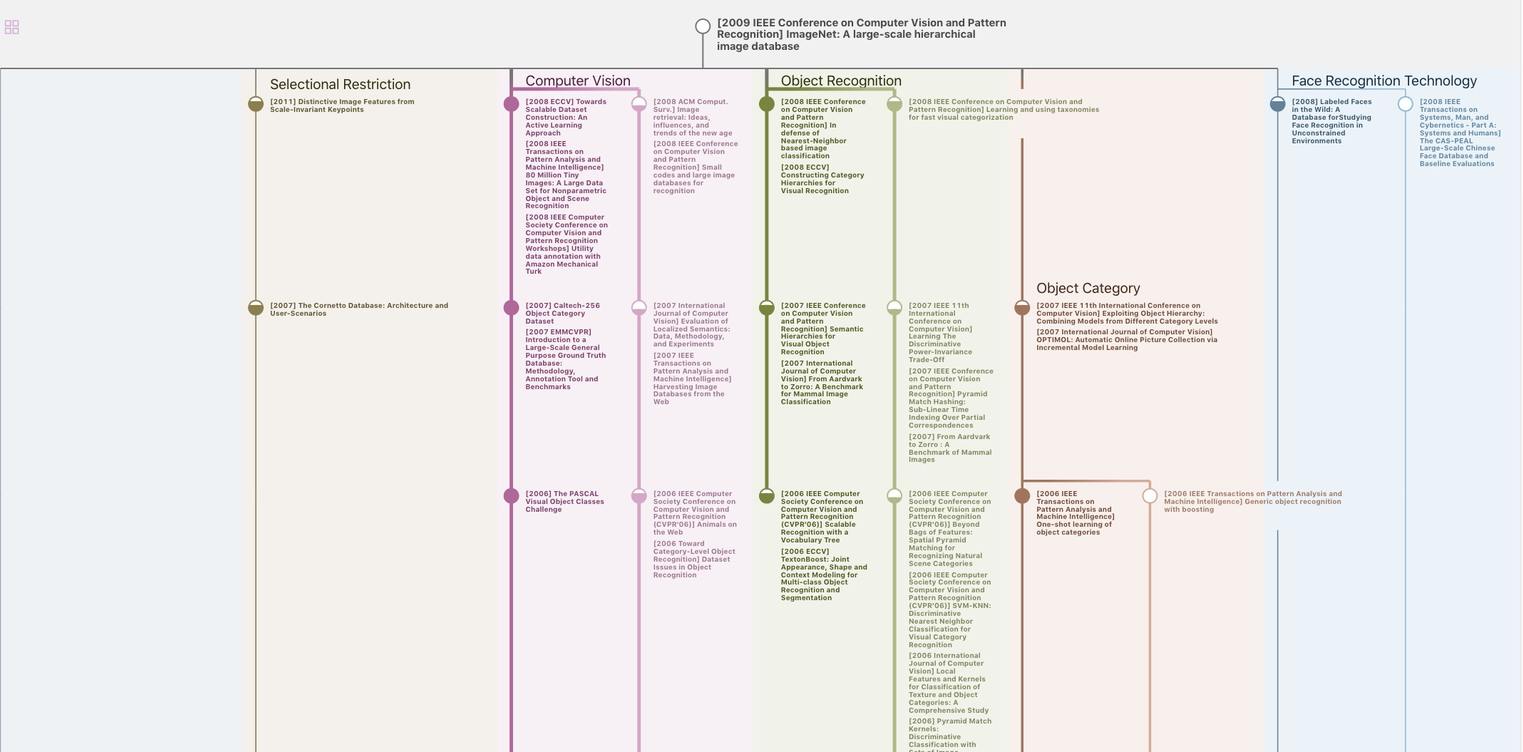
生成溯源树,研究论文发展脉络
Chat Paper
正在生成论文摘要