MixLight: Mixed-Agent Cooperative Reinforcement Learning for Traffic Light Control
IEEE TRANSACTIONS ON INDUSTRIAL INFORMATICS(2024)
摘要
Optimizing traffic light configuration is viewed as a method to increase the traffic throughput in urban cities. Recent studies have employed reinforcement learning to optimize the traffic light configuration. However, the assumption of these studies is oversimplified as all traffic lights are controlled by one unified policy. In the real world, the situation becomes more complicated as a city may deploy more than one traffic light policy due to the different development stages of the city. In this work, we propose a novel multiagent reinforcement learning method, called MixLight, which aims to learn the traffic light configuration under an environment of mixed policies. Our contribution is twofold. First, we propose an executor-guide dual network, in which the guide network changes the executor network optimization direction via reward shaping. Second, we improve the centralized training and decentralized execution framework for the traffic light environment, which reduces the exploration space of agents and decreases the nonstationary during training process. This assists the agents in achieving a cooperative strategy based on their local observations during the execution. Experiments on real-world and synthetic datasets verify the superiority of our proposed method.
更多查看译文
关键词
Cooperative systems,deep neural networks,multiagent systems,reinforcement learning (RL),traffic light control
AI 理解论文
溯源树
样例
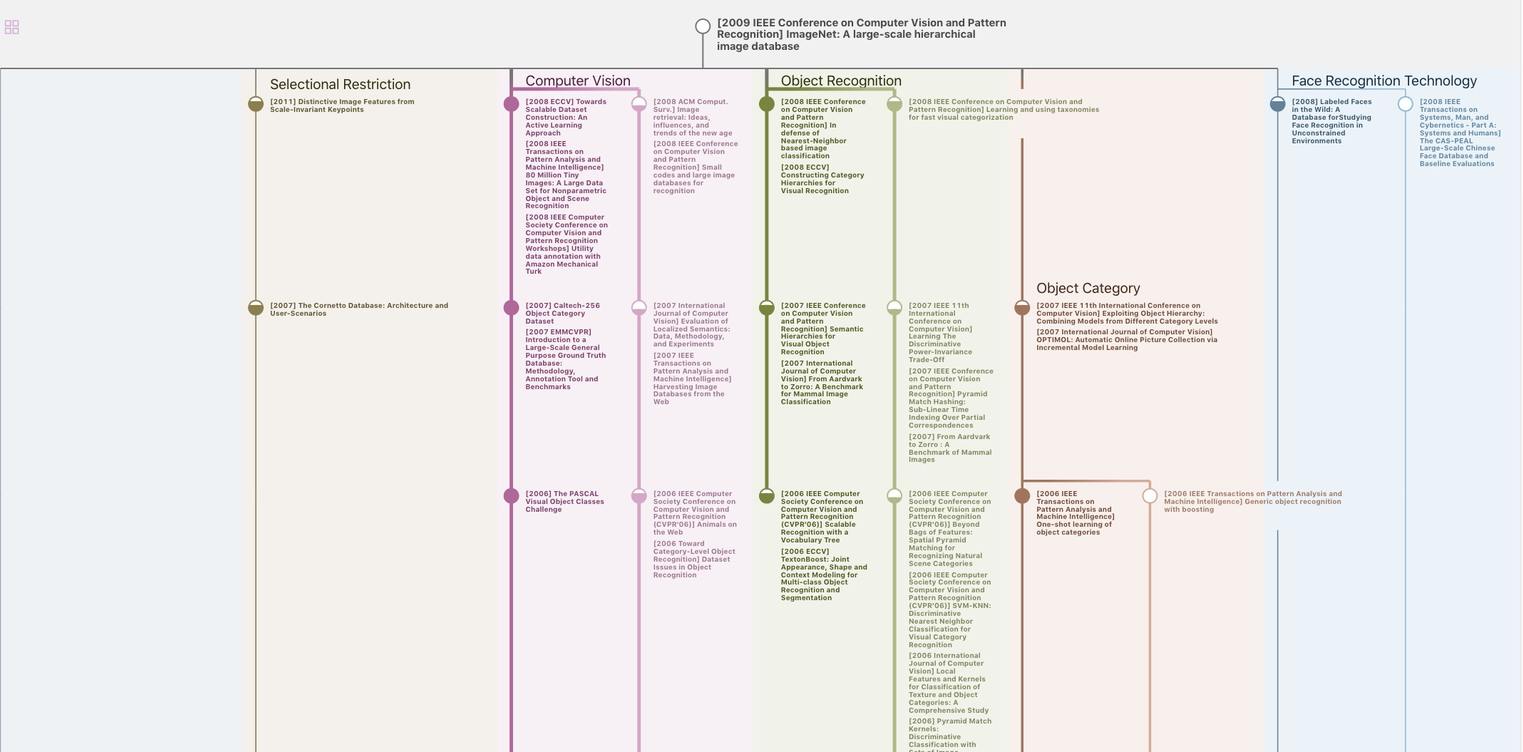
生成溯源树,研究论文发展脉络
Chat Paper
正在生成论文摘要