Curvature-driven Multi-stream Network for Feature-preserving Mesh Denoising
COMPUTER GRAPHICS FORUM(2024)
摘要
Mesh denoising is a fundamental yet challenging task. Most of the existing data-driven methods only consider the zero-order information (vertex location) and first-order information (face normal). However, higher-order geometric information (such as curvature) is more descriptive for the shape of the mesh. Therefore, in order to impose such high-order information, this paper proposes a novel Curvature-Driven Multi-Stream Graph Convolutional Neural Network (CDMS-Net) architecture. CDMS-Net has three streams, including curvature stream, face normal stream and vertex stream, where the curvature stream focuses on the high-order Gaussian curvature information. Moreover, CDMS-Net proposes a novel block based on residual dense connections, which is used as the core component to extract geometric features from meshes. This innovative design improves the performance of feature-preserving denoising. The plug-and-play modular design makes CDMS-Net easy to be implemented. Multiple sets of ablation study are carried out to verify the rationality of the CDMS-Net. Our method establishes new state-of-the-art mesh denoising results on publicly available datasets. A novel multi-stream graph convolutional network for 3D mesh feature-preserving denoising. Gaussian curvature is introduced into the 3D mesh denoising deep network, which improves the feature-preserving denoising performance.image
更多查看译文
关键词
curvature,GCN,mesh denoising
AI 理解论文
溯源树
样例
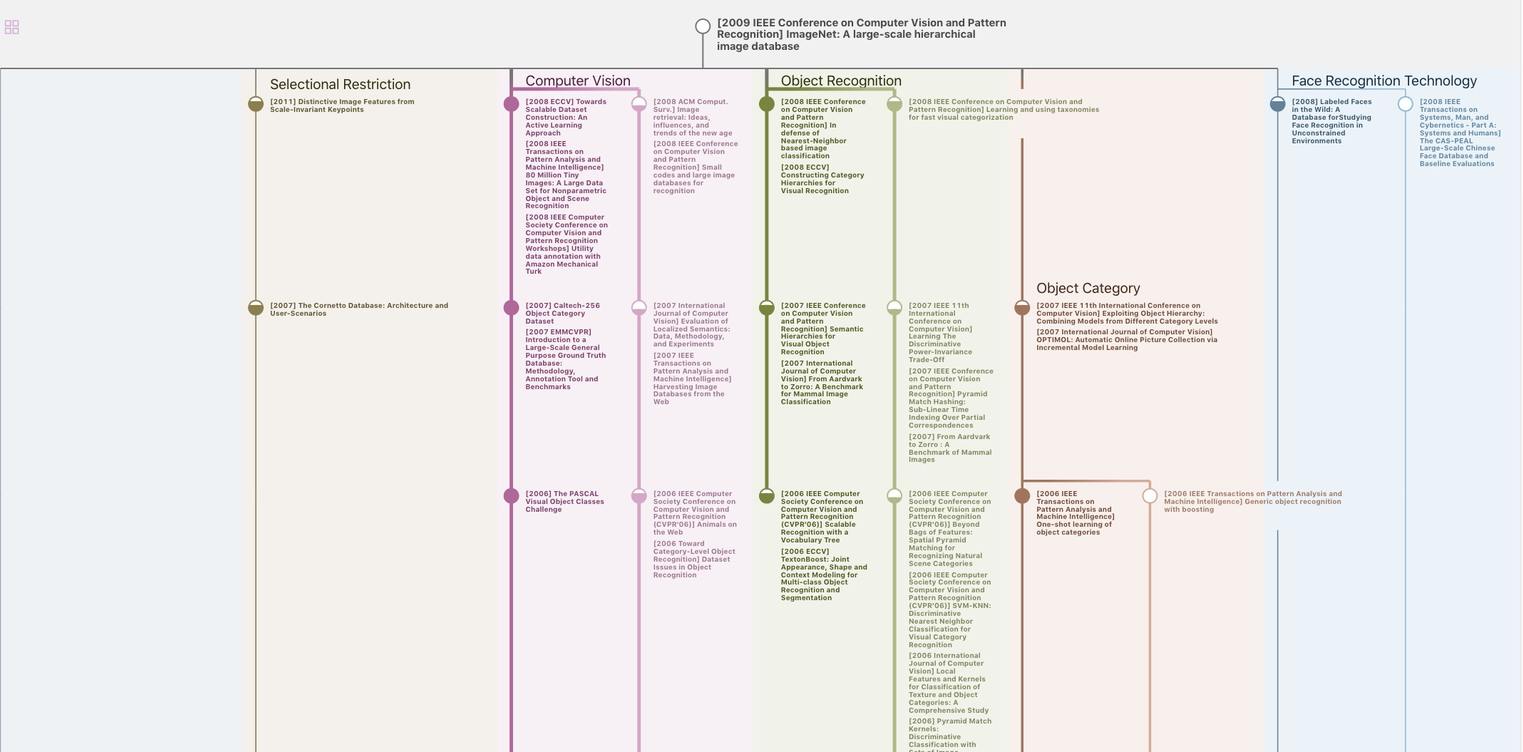
生成溯源树,研究论文发展脉络
Chat Paper
正在生成论文摘要