SSGCN: a sampling sequential guided graph convolutional network
INTERNATIONAL JOURNAL OF MACHINE LEARNING AND CYBERNETICS(2023)
摘要
Graph convolutional networks(GCNs) have become one of the important technologies for solving graph structured data problems. GCNs utilize convolutional networks to learn node and spatial features in the graph, and fully fuse them for node classification tasks. Consequently, for most GCNs, “graph convolution” operation over the set of nodes is the key. Nevertheless, such an operation is frequently embedded into a fixed graph without considering the dynamic variation of the set of nodes. Immediately, the incremental learning mechanism can be considered. From this viewpoint, a Sampling Sequential Guided Graph Convolutional Network (SSGCN) is developed. Firstly, through random sampling over the set of nodes, multiple minibatch graphs can be obtained. Secondly, by the proposed sequential guidance, the weight matrices can be updated incrementally by using “graph convolution” over minibatch graphs one by one. That is, the trained weight matrix of the previous minibatch graph is saved, which in turn is used as an input for training the next minibatch graph. Finally, the prediction results from all minibatch graph learners are integrated. We conducted experiments based on the standard variance of different τ number of losses, and over three common citation network datasets (Cora, Citeseer and Pubmed) to evaluate the performance of SSGCN in node classification tasks. The experimental results show that, in comparison study and ablation study,in terms of both efficiency and effectiveness, the performance of SSGCN is superior to most state-of-the-art methods. In addition, SSGCN shows good convergence in visualization.
更多查看译文
关键词
Graph convolutional networks,Incremental learning,Minibatch graph,Random sampling,Sequential guidance
AI 理解论文
溯源树
样例
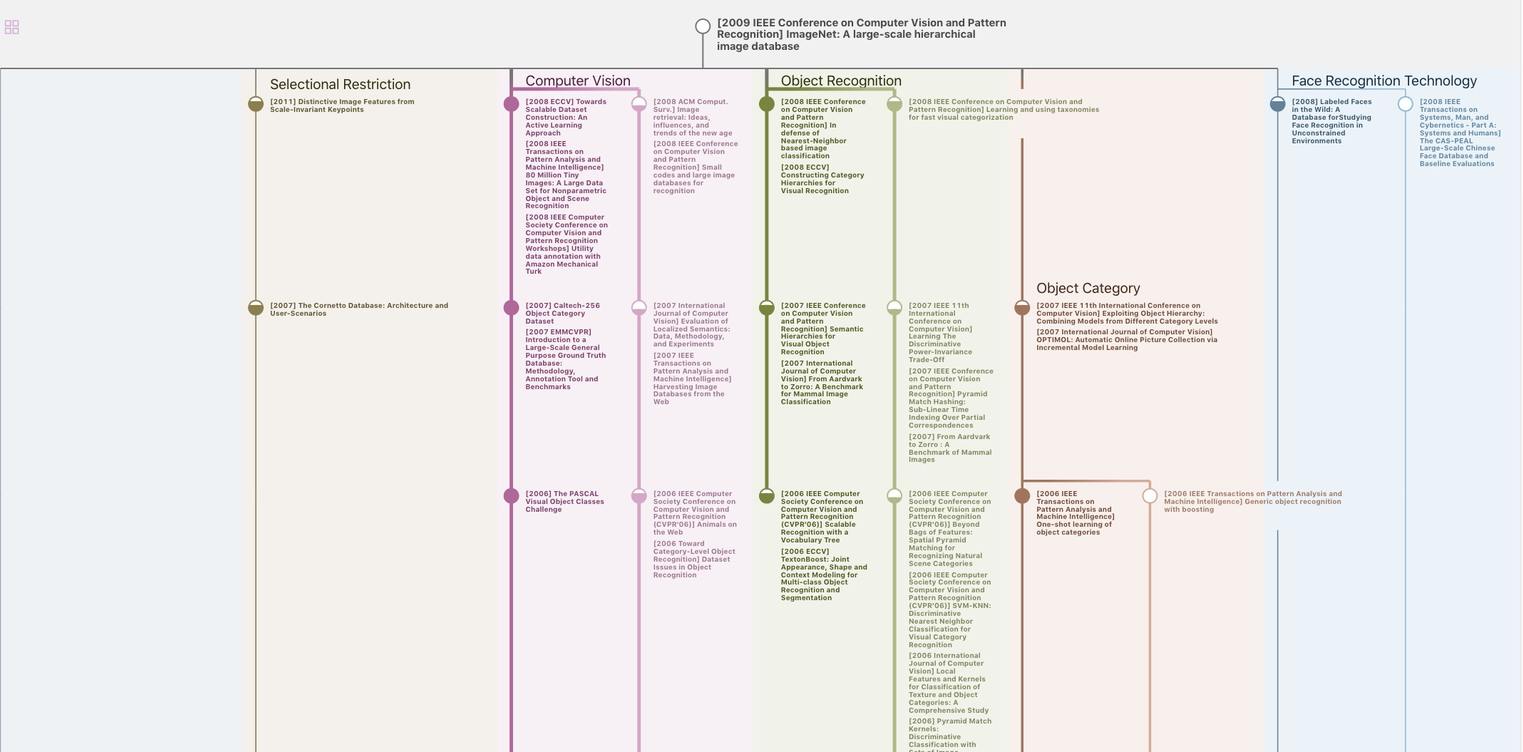
生成溯源树,研究论文发展脉络
Chat Paper
正在生成论文摘要