Integration of ASTER and Soil Survey Data by Principal Components Analysis and One-Class Support Vector Machine for Mineral Prospectivity Mapping in Kerkasha, Southwestern Eritrea
NATURAL RESOURCES RESEARCH(2023)
摘要
This study evaluates the potential for mineral prospectivity mapping (MPM) within the Kerkesha area, southwestern Eritrea, using remote sensing and geochemical data analysis. The Advanced Spaceborne Thermal Emission and Reflection Radiometer (ASTER) remote sensing data were used for mapping zones of hydrothermal alteration, while assessment of geologic structures was based on automated extraction of lineaments from a digital elevation model (DEM). Integration of these alteration and structural datasets with surface geochemical data was carried out to identify pathfinder elements associated with Au–Cu–Zn mineralization as well as to evaluate and delineate anomalous regions in this relatively underexplored region of the Arabia Nubia Shield (ANS). Specifically, the modeling approach for the extraction and interpretation of mineralization-related spectral footprints was the selective principal component analysis (SPCA), and the lineament features extracted from DEM derivatives were integrated with the soil geochemical data and modeled by principal component analysis (PCA). The results revealed a NE–SW trend of lineaments, delineated zones of hydrothermal alteration indicating the presence of multi-deposit type mineralization, and identified pathfinder elements. In addition, Au–Cu–Zn anomalous zones were extracted by one-class support vector machine (OCSVM) and the performance of such classification was validated by the area under the curve (AUC) and the Youden index computed from receiver operating characteristic (ROC) curves and the Kruskal–Wallis test. The results showed significance in differences between the anomalous and non-anomalous zones and existence of a relationship between known mineral deposits and predicted anomalies. The proposed MPM shows promising results for robust automated delineation and understanding of mineralization processes.
更多查看译文
关键词
SPCA,One-class support vector machine,Lineaments,Predictive PCA,Geochemical anomaly,ASTER
AI 理解论文
溯源树
样例
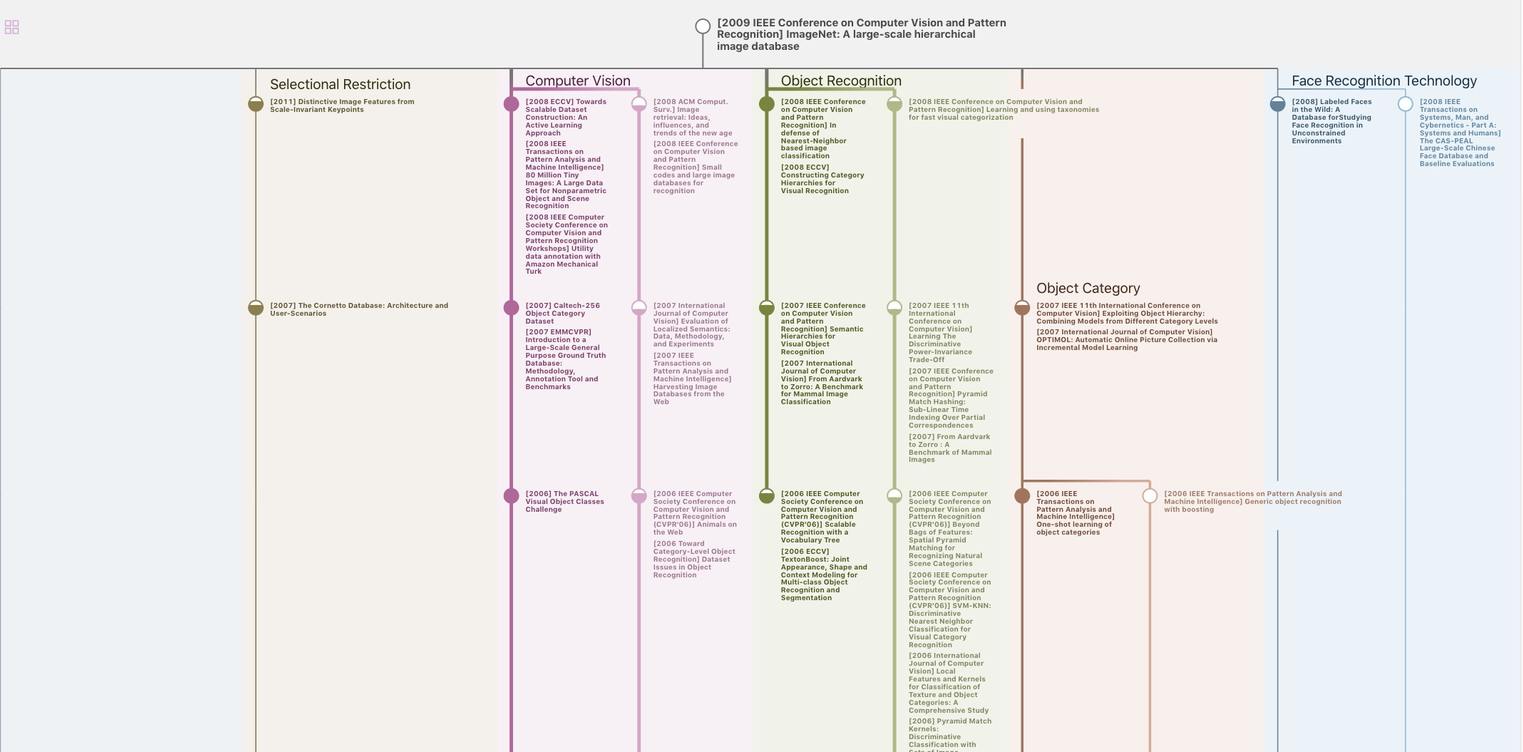
生成溯源树,研究论文发展脉络
Chat Paper
正在生成论文摘要