Stochastic projective splitting
COMPUTATIONAL OPTIMIZATION AND APPLICATIONS(2024)
摘要
We present a new, stochastic variant of the projective splitting (PS) family of algorithms for inclusion problems involving the sum of any finite number of maximal monotone operators. This new variant uses a stochastic oracle to evaluate one of the operators, which is assumed to be Lipschitz continuous, and (deterministic) resolvents to process the remaining operators. Our proposal is the first version of PS with such stochastic capabilities. We envision the primary application being machine learning (ML) problems, with the method's stochastic features facilitating "mini-batch" sampling of datasets. Since it uses a monotone operator formulation, the method can handle not only Lipschitz-smooth loss minimization, but also min-max and noncooperative game formulations, with better convergence properties than the gradient descent-ascent methods commonly applied in such settings. The proposed method can handle any number of constraints and nonsmooth regularizers via projection and proximal operators. We prove almost-sure convergence of the iterates to a solution and a convergence rate result for the expected residual, and close with numerical experiments on a distributionally robust sparse logistic regression problem.
更多查看译文
关键词
Proximal operator splitting,Monotone inclusions,Convex optimization,Stochastic gradient descent
AI 理解论文
溯源树
样例
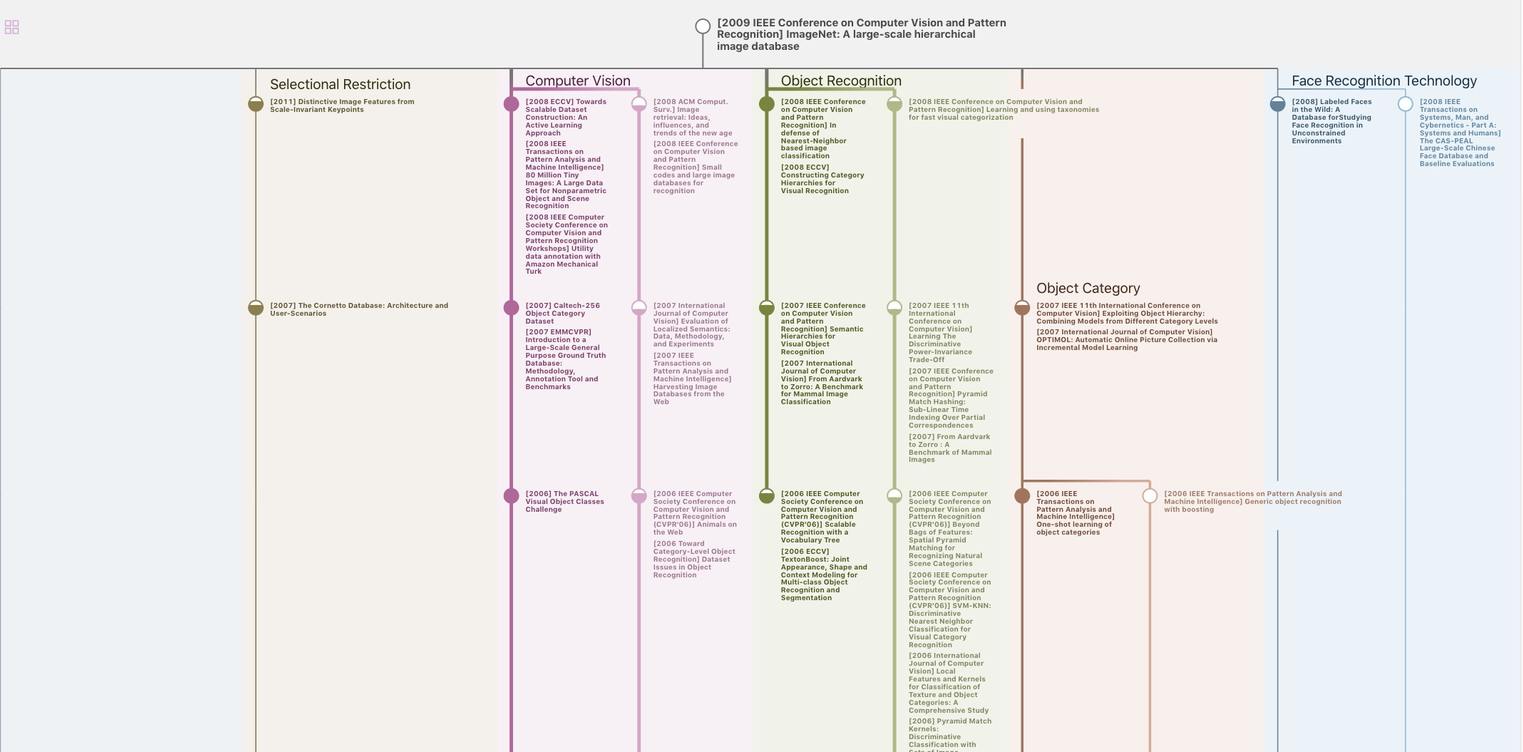
生成溯源树,研究论文发展脉络
Chat Paper
正在生成论文摘要