Unsupervised Learning Using EHR Data To Detect Distinct Subphenotypes Of Hypertension
HYPERTENSION(2023)
摘要
Introduction: Hypertension (HTN) is a complex condition with significant heterogeneity in presentation and treatment response. Identifying distinct subphenotypes of HTN may improve our understanding of its underlying mechanisms and guide more precise treatment or public health initiatives. Methods: Using EHR data from the OneFlorida+ research consortium (2012-2021), we identified a cohort of adult Floridians with newly-diagnosed HTN (first diagnosis following 2 outpatient BPs ≥140/90 mmHg & no prior antiHTN treatment). We extracted demographic and clinical data from the diagnosis visit and ≤1 year prior. We used hierarchical clustering to identify distinct subphenotypes within the HTN population. Results: A total of 40,741 patients were included (mean ± SD age, 61 ± 18 y; 55% women). Six subphenotypes (S1-6) were identified (Figure). For example, S1 was characterized by older age and higher BMI and T2DM prevalence, while S2 included younger individuals with lower BMI and more women, and S3 had low prevalence of HTN-related comorbidity. Distinctions in SDOH factors were also observed: despite lower comorbidity burden, the youngest subphenotype (S2) had high SDOH burden and presented with HTN 8-15 y earlier than higher income subphenotypes (e.g., S4 & S5). Conclusions: Unsupervised learning identified 6 HTN subphenotypes varying in demographic, socioeconomic and risk profiles. Further investigation into biological mechanisms of these subphenotypes and their relationships to social factors may enhance our ability to deliver targeted interventions that consider social policy implications in addition to the traditional behavioral and physiological interventions.
更多查看译文
关键词
ehr data,hypertension,distinct subphenotypes,unsupervised learning
AI 理解论文
溯源树
样例
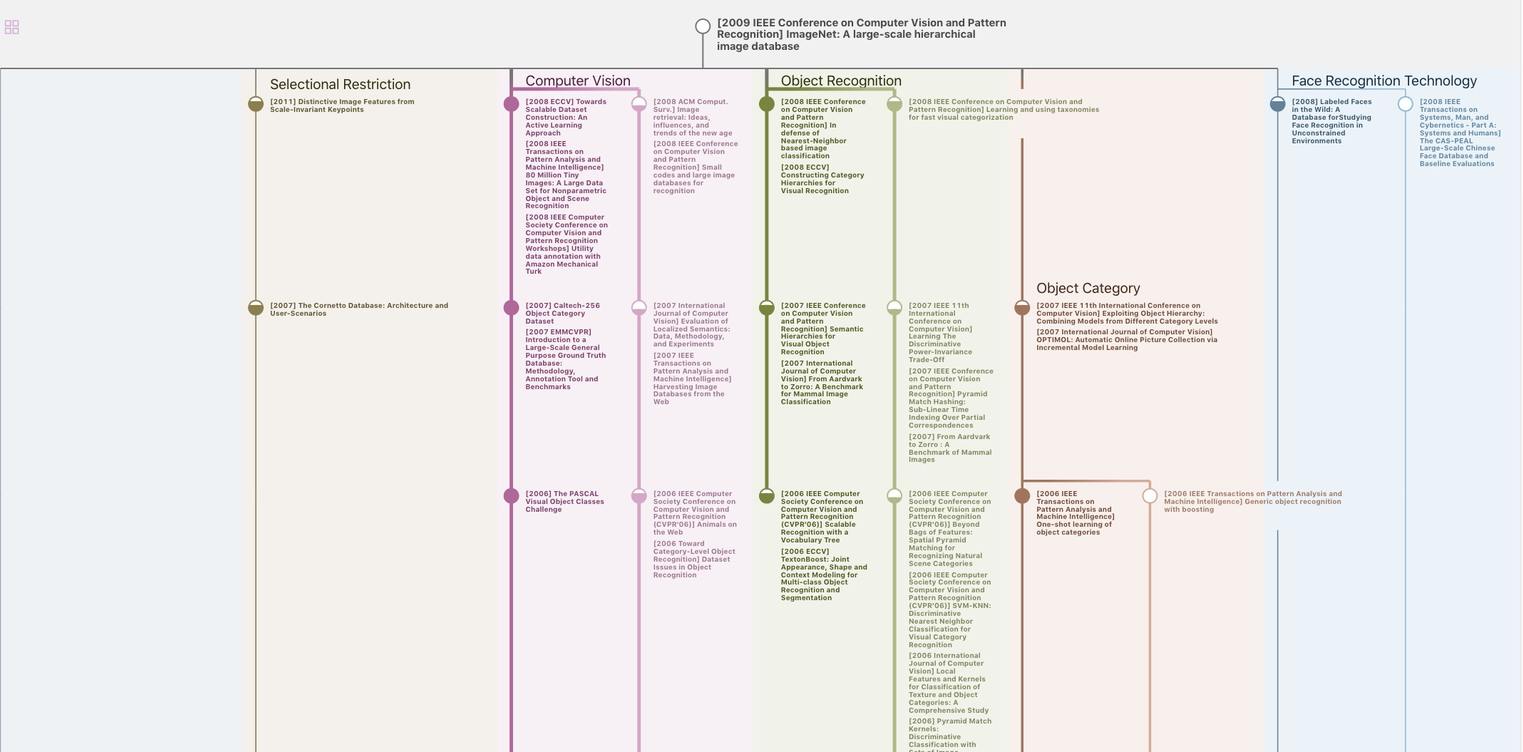
生成溯源树,研究论文发展脉络
Chat Paper
正在生成论文摘要