Attention-Guided Optimal Transport for Unsupervised Domain Adaptation with Class Structure Prior
NEURAL PROCESSING LETTERS(2023)
摘要
Unsupervised domain adaptation(UDA) methods based on optimal transport have been successfully used to improve cross-domain classification performance. Optimal transport aligns the distribution of source domain and target domain by minimizing the transport cost. However, the existing works based on optimal transport ignore the class-structure prior information of domains and do not adequately reflect the real data distribution. It always leads to be difficult in distinguishing target instances near the decision boundary. In this paper, we propose an end-to-end Attention-guided Optimal Transport (AOT) framework to achieve better domain adaptation. Concretely, first we introduce a weighted cost matrix based on the self-attention mechanism to reduce the bias caused by minibatch selection in training. It is realized by relating the prediction results in source and target domains. Meanwhile, a Jensen–Shannon divergence (JSD) regularization term is exploited to establish the mutual relationship between the feature space and the label space to achieve more reliable transport plan. Second, in order to enhance the discriminability of domain-invariant features using the class-structure prior, we also develop a pairwise metric learning strategy. It defines the positive/negative pairs by labels and enhances the class-structure prior by coupling feature and label similarities. Finally, we compare the proposed methods with ten SOTA approaches on multiple single source benchmarks and a multi-source benchmarks. The experimental results demonstrate that AOT achieves the best performance for classification tasks.
更多查看译文
关键词
Unsupervised domain adaptation,Weighted optimal transport,Attention-guided cost matrix,Class-structure prior
AI 理解论文
溯源树
样例
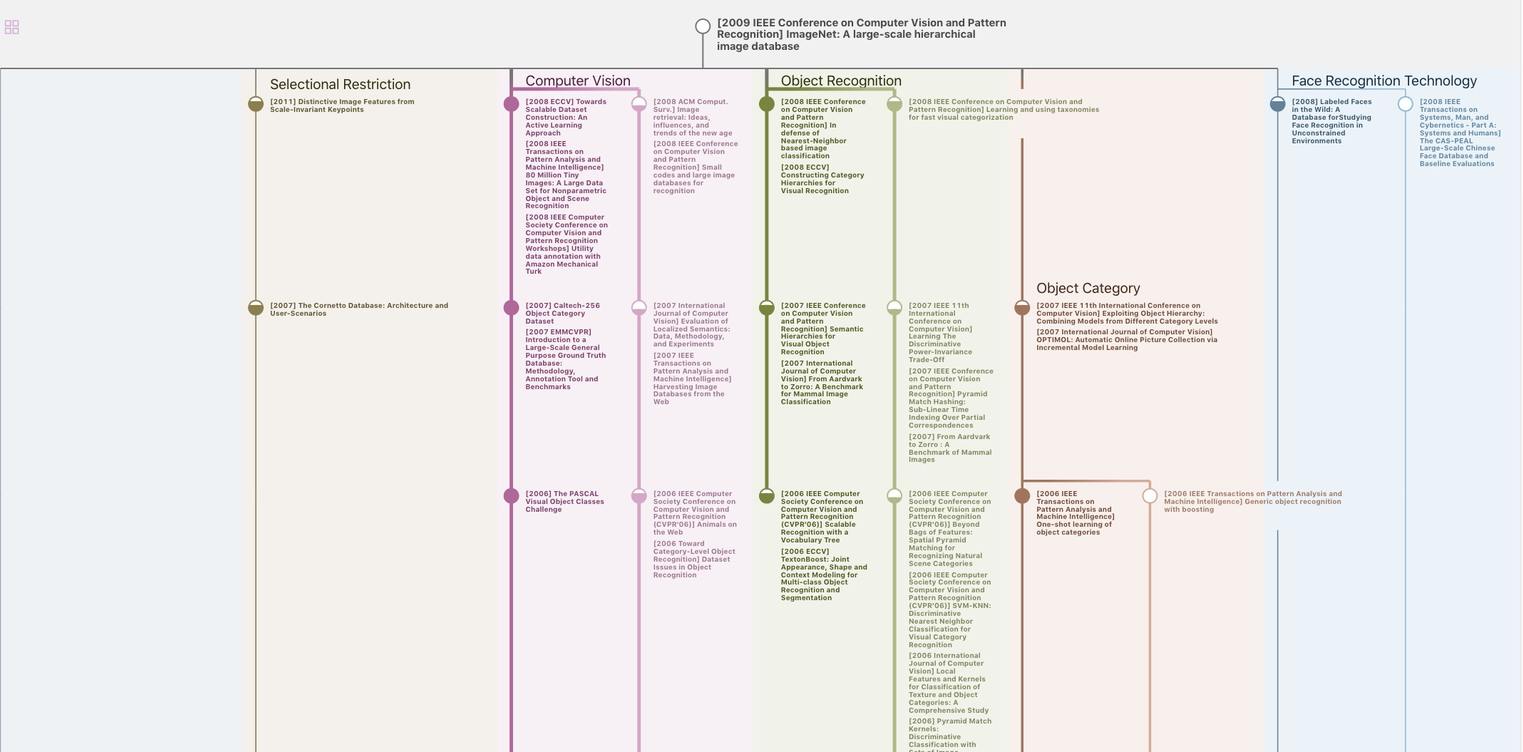
生成溯源树,研究论文发展脉络
Chat Paper
正在生成论文摘要