Enhanced approach of multilabel learning for the Arabic aspect category detection of the hotel reviews
COMPUTATIONAL INTELLIGENCE(2024)
摘要
In many fields, like aspect category detection (ACD) in aspect-based sentiment analysis, it is necessary to label each instance with more than one label at the same time. This study tackles the multilabel classification problem in the ACD task for the Arabic language. For this purpose, we used Arabic hotel reviews from the SemEval-2016 dataset, comprising 13,113 annotated tuples provided for training (10,509) and testing (2,604). To extract valuable information, we first propose specific data preprocessing. Then, we suggest using the dynamic weighted loss function and a data augmentation method to fix the problem with this dataset's imbalance. Using two possible approaches, we develop new ways to find different categories of things in a review sentence. The first is based on classifier chains using machine learning models. The second is based on transfer learning using pretrained AraBERT fine-tuning for contextual representation. Our findings show that both approaches outperformed the related works for ACD on the Arabic SemEval-2016. Moreover, we observed that AraBERT fine-tuning performed much better and achieved a promising F1$$ {F}_1 $$-score of 68.02%$$ 68.02\% $$.
更多查看译文
关键词
AraBERT,Arabic hotel reviews,aspect category,imbalanced data,multilabel classification,preprocessing
AI 理解论文
溯源树
样例
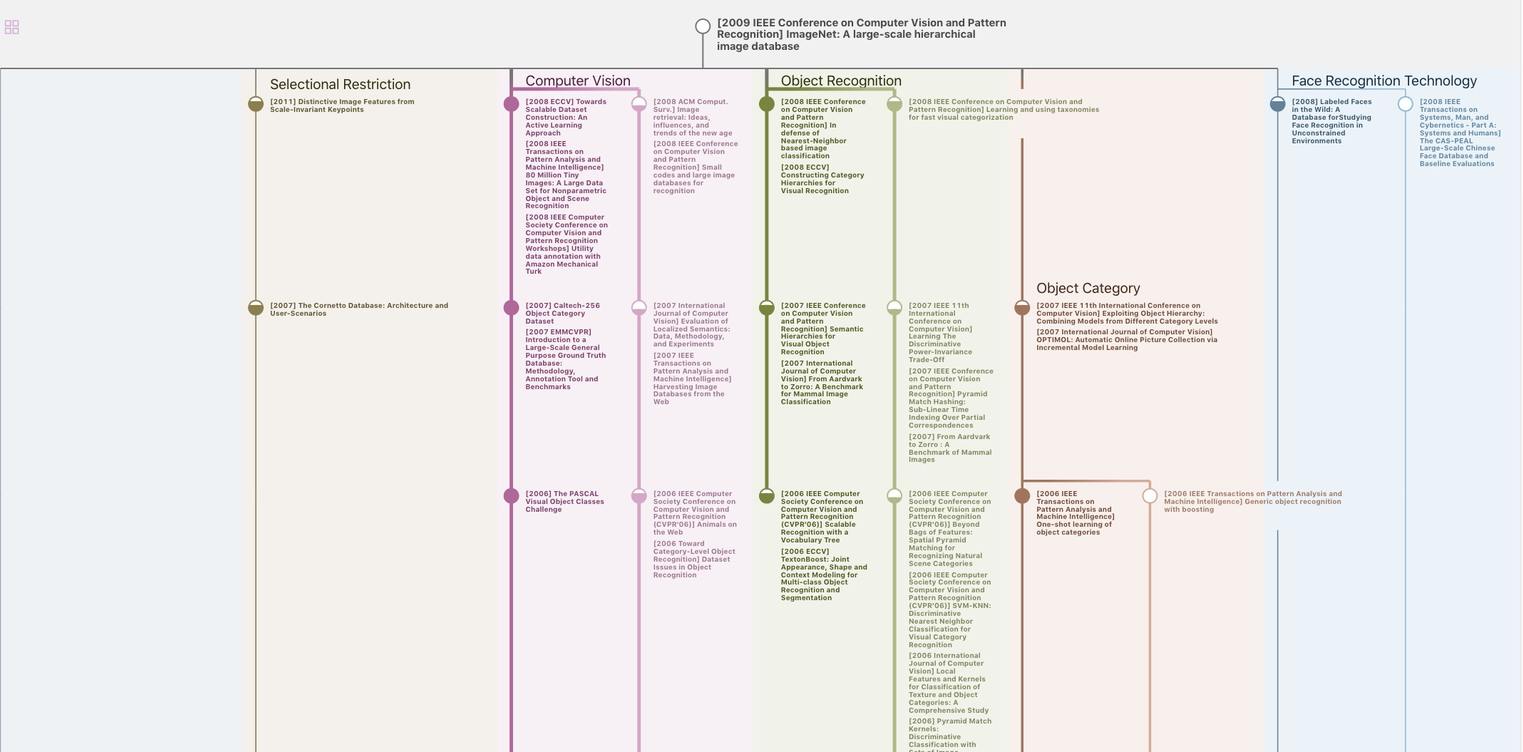
生成溯源树,研究论文发展脉络
Chat Paper
正在生成论文摘要