A novel lidar signal noise reduction algorithm based on improved deep belief network
PHYSICA SCRIPTA(2023)
摘要
To reduce noise in the lidar return signal, an improved deep belief network (DBN) denoising algorithm is proposed in this study. In the traditional implementation process of DBN, a multi-layer fully connected network is realized by stacking restricted Boltzmann machines (RBMs). However, the RBM is an undirected graph model, and there is no clear causal relationship between random variable nodes. The denoising autoencoder (DAE) can avoid this problem and produce field generalization performance by adding random contamination during training and stacking, thereby achieving better performance than the traditional DBN. In this study, a new multi-layer DBN called DADBN is implemented by stacking DAE and RBM. First, the multi-layer DAE is placed in the beginning layer of the network as the primary filter of the signal to provide data dimensionality reduction and feature extraction. Then, the RBM is used as the lower layer, the hidden layer is calculated according to the initial value of the visible layer by the contrast of the divergence algorithm, and the visible layer is reconstructed from the samples of the hidden layer. And the sparse representation penalty is added to the RBM model to solve the assimilation phenomenon of hidden layer nodes in the RBM model. It not only optimizes the log-likelihood function when training data, but also makes the probability of each hidden layer node being activated tend to a minimum value, so as to sparsely activate the sparse hidden layer nodes. The weight matrix W is obtained by computing the results of the hidden layer twice. Finally, the original input signal was reconstructed by Gibbs sampling layer and the reconstructed signal was decoded by the decoder to achieve the purpose of noise reduction. To verify its effectiveness, this method is compared with four other denoising methods: wavelet packet algorithm, complete ensemble empirical modal decomposition (CEEMDAN), wavelet transform and empirical mode decomposition (WT-EMD), and wavelet transform-variational mode decomposition (WT-VMD). The noise reduction effect of DADBN is better than that of the other four methods, thus effectively eliminating complex noise in the lidar detection signal.
更多查看译文
关键词
lidar,deep learning,deep belief network,denoising autoencoder,noise reduction
AI 理解论文
溯源树
样例
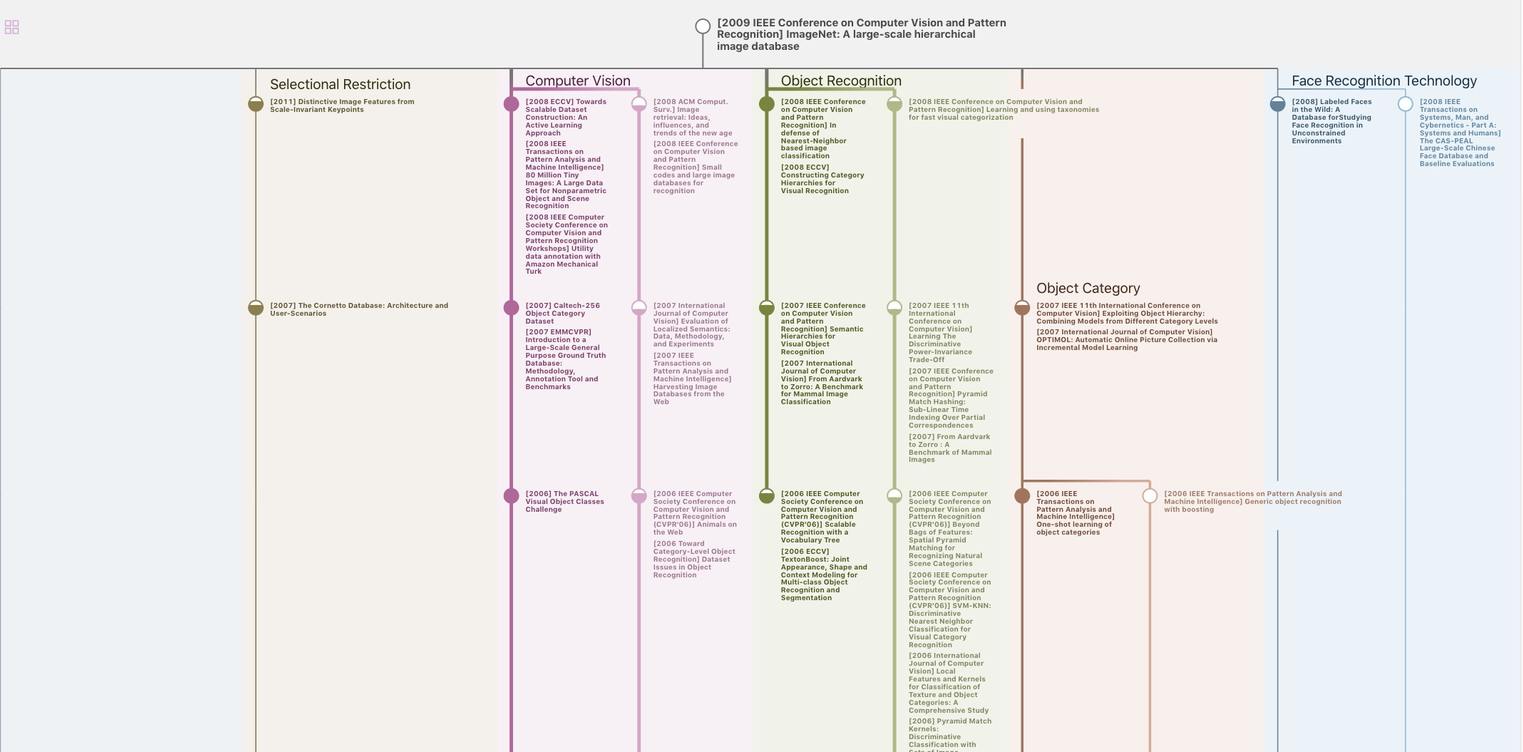
生成溯源树,研究论文发展脉络
Chat Paper
正在生成论文摘要