HPFL: hyper-network guided personalized federated learning for multi-center tuberculosis chest x-ray diagnosis
MULTIMEDIA TOOLS AND APPLICATIONS(2023)
摘要
Tuberculosis (TB) is one of the widespread infectious disease, and the early diagnosis and treatment can greatly improve the survival rate. Recently, machine learning has been introduced for assisting the diagnosis of TB, and to train a reliable diagnosis model, we need large amounts of data, which are often distributed in multiple medical centers. To protect the data privacy of different centers, we introduce federated learning (FL) in tuberculosis diagnosis. Since the data distributions of TB data vary significantly across different centers, we propose a personalized FL (PFL) method to explore the specific property of each client (i.e., medical center), and reduce its negative impacts from other clients. In particular, the contribution of each layer parameter is quantified by a hyper-network customized by the server for each client. Besides, a parameterization mechanism is introduced to update the hierarchical aggregation weights. To the best of our knowledge, this is the first PFL method for distributed TB diagnosis. Experimental results on several public datasets of chest X-ray images show that the proposed method significantly outperforms the state-of-the-art approaches in terms of both higher accuracy and faster convergence speed.
更多查看译文
关键词
Hyper-network, Federated learning, Tuberculosis, Chest x-ray
AI 理解论文
溯源树
样例
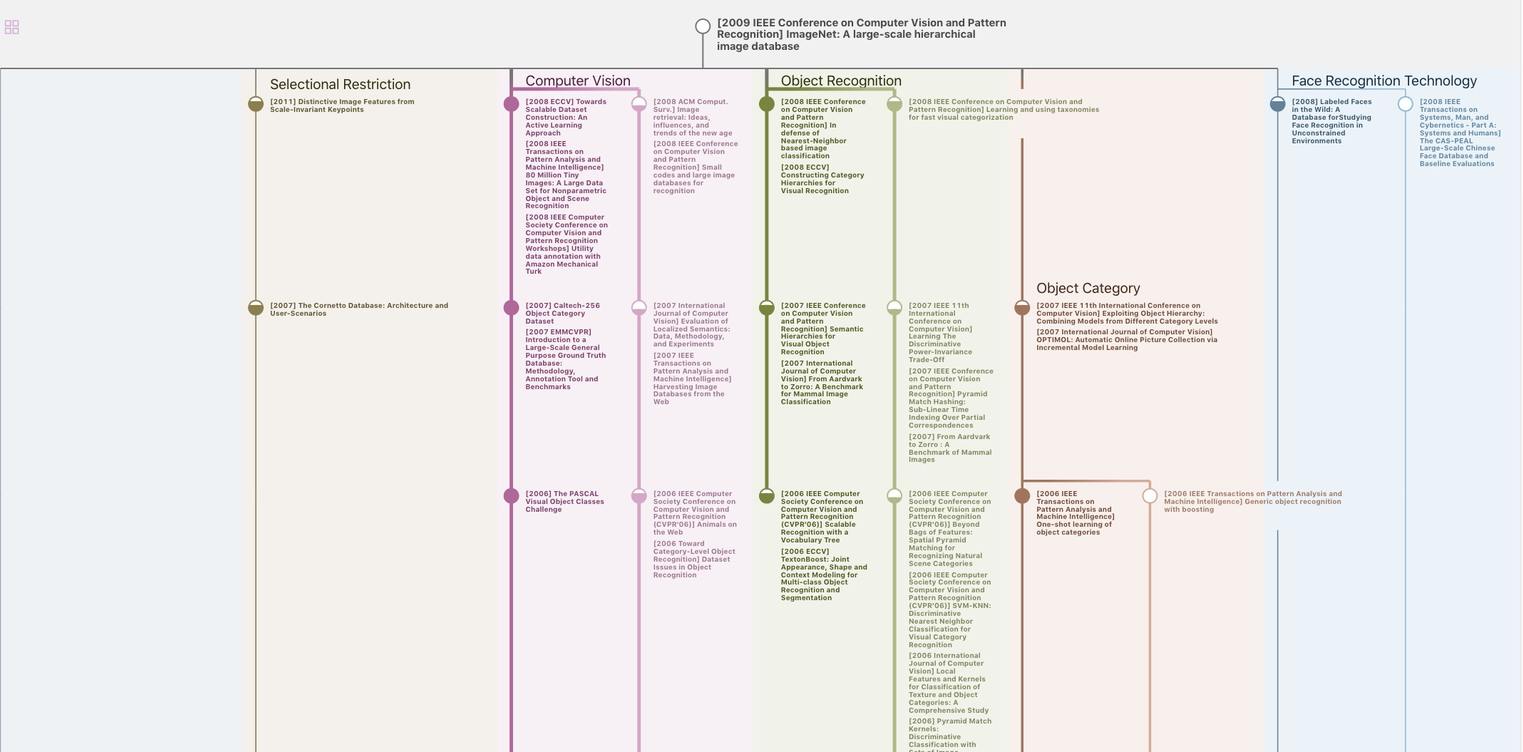
生成溯源树,研究论文发展脉络
Chat Paper
正在生成论文摘要