An improved fault diagnosis method for rolling bearings based on wavelet packet decomposition and network parameter optimization
MEASUREMENT SCIENCE AND TECHNOLOGY(2024)
摘要
The diagnosis of faults in rolling bearings plays a critical role in monitoring the condition and maintaining the performance of rotating machinery, while also preventing major accidents. In this article, a new approach to diagnosing faults in rolling bearings is proposed, using wavelet packet decomposition (WPD) for features extraction and the chaotic sparrow search optimization algorithms (CSSOAs) to optimize the parameters of a deep belief network (DBN). Firstly, the WPD method is used for the decomposition of vibration signals in rolling bearings, which are decomposed into three layers, and reconstruction is performed on the nodes of the last layer based on the decomposition. Furthermore, the energy characteristics of the reconstructed nodes are then utilized as inputs to DBN, and the CSSOA is employed to optimize the hyperparameters of DBN. Ultimately, a fault diagnosis model combining WPD with optimizing parameters is presented. This model is validated on bearing datasets from Case Western Reserve University (CWRU) and Jiangnan University (JNU). Experimental results indicate that the average accuracy achieved when modeling with WPD-CSSOA-DBN on the CWRU dataset is 98.24% , with a root mean square error of 0.0713. On the JNU bearing dataset, the modeling achieves an average accuracy of 95.15% with a root mean square error of 0.1018. Compared to other methods, this approach demonstrates stronger feature extraction capabilities and outstanding rolling bearing fault diagnosis abilities.
更多查看译文
关键词
rolling bearings fault diagnosis,wavelet packet decomposition,deep belief network,chaotic sparrow search optimization algorithms,optimizing parameters
AI 理解论文
溯源树
样例
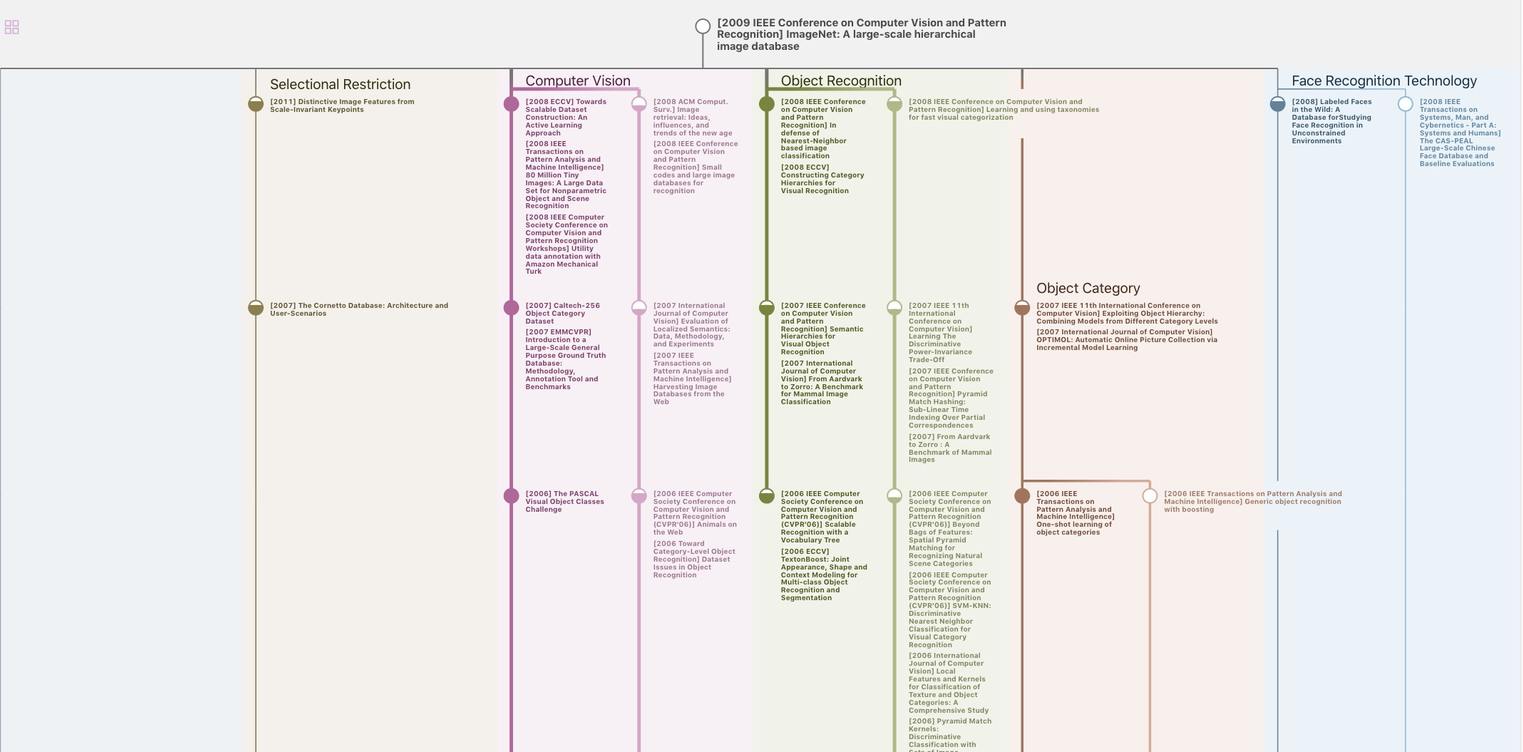
生成溯源树,研究论文发展脉络
Chat Paper
正在生成论文摘要