Bird-Snack: Bayesian inference of dust law R V distributions using SN Ia apparent colours at peak
MONTHLY NOTICES OF THE ROYAL ASTRONOMICAL SOCIETY(2023)
摘要
To reduce systematic uncertainties in Type Ia supernova (SN Ia) cosmology, the host galaxy dust law shape parameter, R-V, must be accurately constrained. We thus develop a computationally ine xpensiv e pipeline, (0:sc ) Bird-Snack( /0:sc), to rapidly infer dust population distributions from optical-near-infrared SN colours at peak brightness, and determine which analysis choices significantly impact the population mean R(V )inference, mu(RV). Our pipeline uses a 2D Gaussian process to measure peak BVriJH apparent magnitudes from SN light curves, and a hierarchical Bayesian model to simultaneously constrain population distributions of intrinsic and dust components. Fitting a low-to-moderate-reddening sample of 65 low-redshift SNe yields mu(RV) = 2 . 61(-0.35)(+0.38 ), with 68 per cent (95 per cent ) posterior upper bounds on the population dispersion, sigma R-V < 0 . 92(1 . 96). This result is robust to various analysis choices, including: the model for intrinsic colour variations, fitting the shape hyperparameter of a gamma dust extinction distribution, and cutting the sample based on the availability of data near peak. However, these choices may be important if statistical uncertainties are reduced. With larger near-future optical and near-infrared SN samples, BIRD-SNACK can be used to better constrain dust distributions, and investigate potential correlations with host galaxy properties. BIRD-SNACK is publicly available; the modular infrastructure facilitates rapid exploration of custom analysis choices, and quick fits to simulated data sets, for better interpretation of real-data inferences.
更多查看译文
关键词
cosmology: observations, methods: statistical, supernovae: general, dust, extinction
AI 理解论文
溯源树
样例
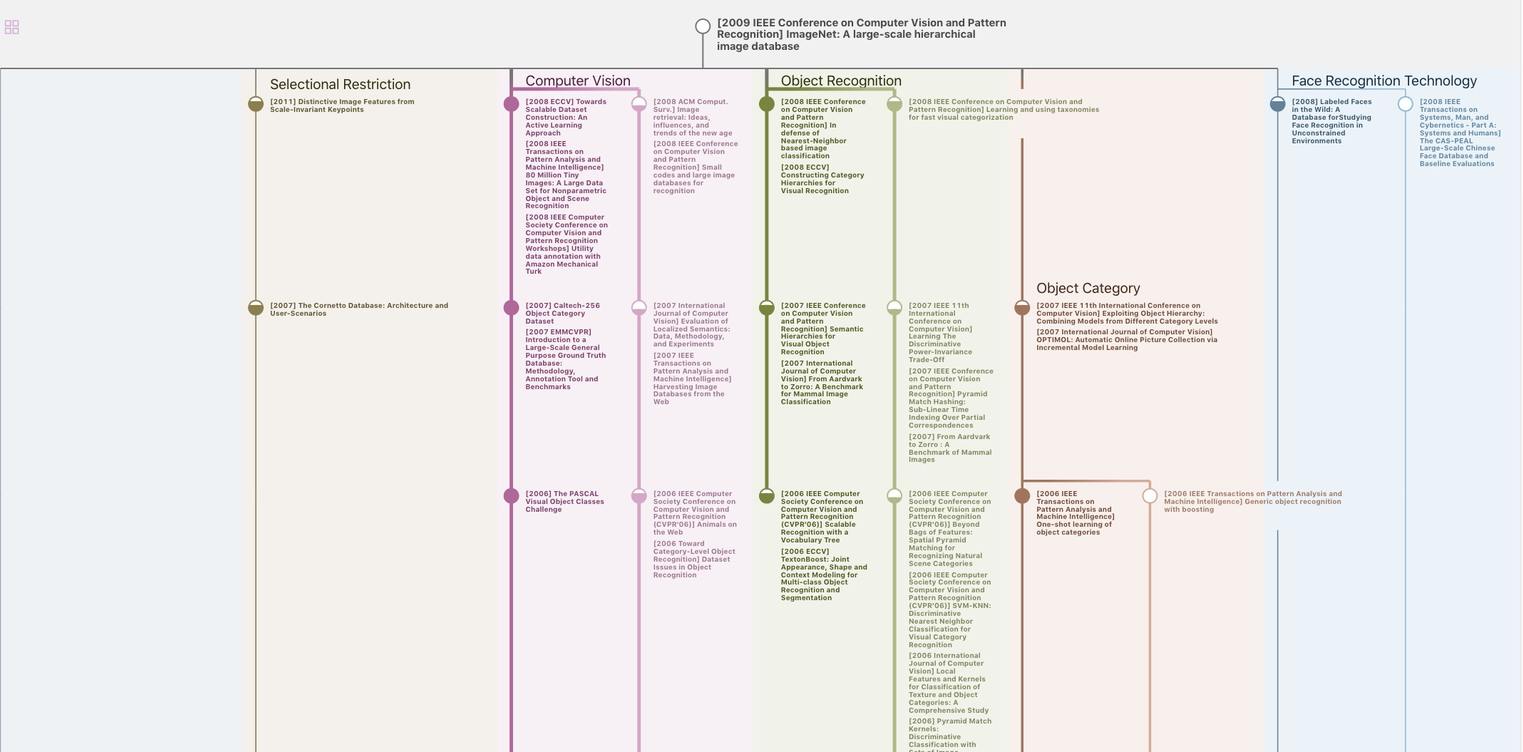
生成溯源树,研究论文发展脉络
Chat Paper
正在生成论文摘要