Multi-Source Deep Domain Adaptation with Weak Supervision for Time-Series Sensor Data
KDD '20: The 26th ACM SIGKDD Conference on Knowledge Discovery and Data Mining Virtual Event CA USA July, 2020(2020)
摘要
Domain adaptation (DA) offers a valuable means to reuse data and models for new problem domains. However, robust techniques have not yet been considered for time series data with varying amounts of data availability. In this paper, we make three main contributions to fill this gap. First, we propose a novel Convolutional deep Domain Adaptation model for Time Series data (CoDATS) that significantly improves accuracy and training time over state-of-the-art DA strategies on real-world sensor data benchmarks. By utilizing data from multiple source domains, we increase the usefulness of CoDATS to further improve accuracy over prior single-source methods, particularly on complex time series datasets that have high variability between domains. Second, we propose a novel Domain Adaptation with Weak Supervision (DA-WS) method by utilizing weak supervision in the form of target-domain label distributions, which may be easier to collect than additional data labels. Third, we perform comprehensive experiments on diverse real-world datasets to evaluate the effectiveness of our domain adaptation and weak supervision methods. Results show that CoDATS for single-source DA significantly improves over the state-of-the-art methods, and we achieve additional improvements in accuracy using data from multiple source domains and weakly supervised signals.
更多查看译文
关键词
weak supervision,adaptation,deep,multi-source,time-series
AI 理解论文
溯源树
样例
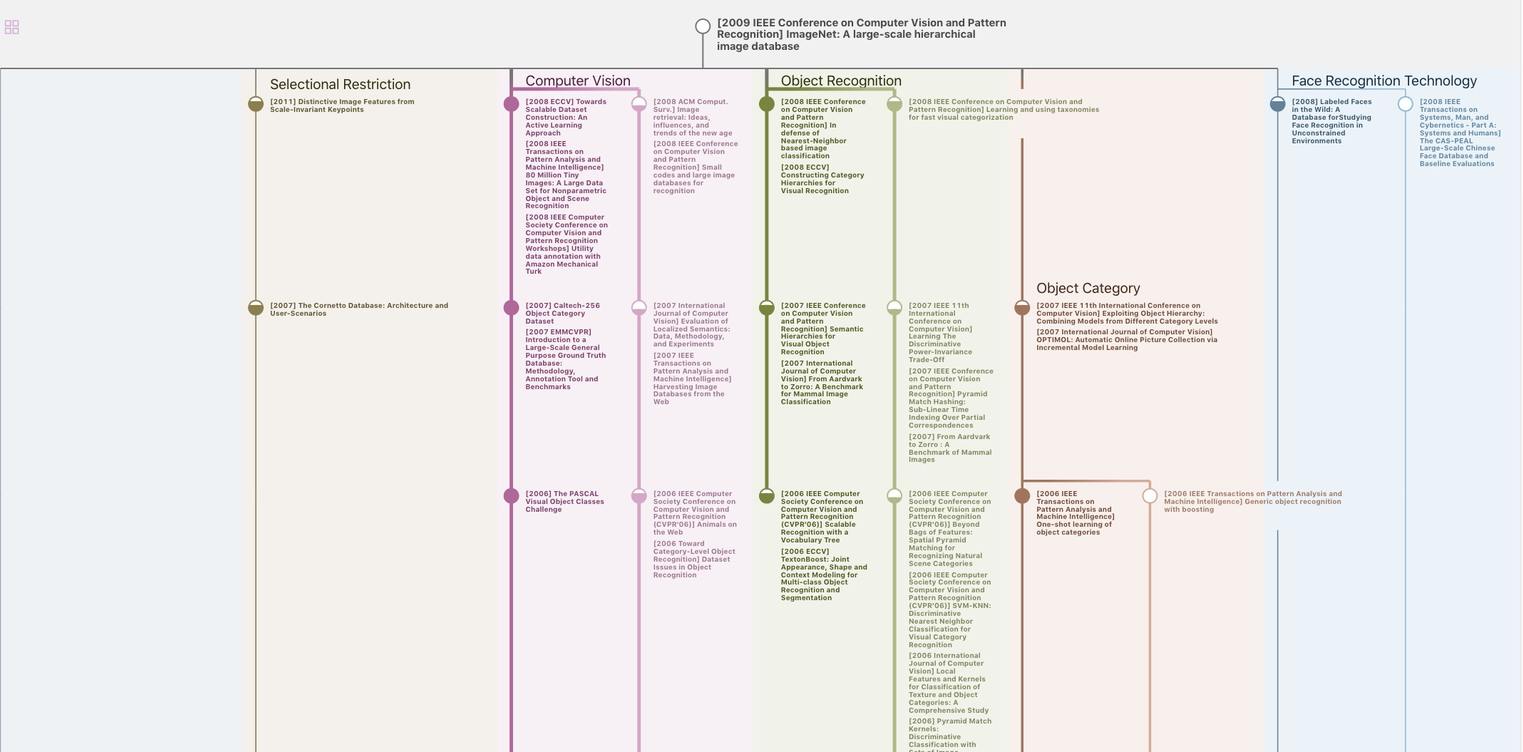
生成溯源树,研究论文发展脉络
Chat Paper
正在生成论文摘要