Multi-layer feature fusion for hyperspectral image classification
INTERNATIONAL JOURNAL OF REMOTE SENSING(2023)
摘要
Convolutional Neural Network (CNN) has been widely used in hyperspectral image (HSI) classification. It has obvious advantages in extracting spatial and spectral features. However, the classification performance depends not only on spatial and spectral information, but also on the sequence properties between spectral features. Transformer is an excellent model for establishing sequence relationship, however making spatial correlation loss. On the basis of preserving spatial correlation as much as possible, we propose a multi-layer feature fusion module (MLFF) to fully mine and fuse the representative spectral and spatial features and sequence relationship. The proposed MLFF is composed of Feature Transformation, Tensor-based Sobel and Transformer Fusion. Feature Transformation is implemented by transpose and convolution operations, which can get the high-level contextual features and increase the diversity of feature information. At the same time, we designed a Tensor-based Sobel module to better participate in the subsequent fusion of HSI edge and texture features. Finally, in the Transformer Fusion module, these features are fused by one feature per layer through the improved encoder and decoder. Besides, we add skip structure to maintain a good relationship between each layer and strengthen cross-layer connections. Through the above three modules, we can better acquire and fuse HSI spectral, spatial and edge features to achieve good classification accuracy. The experiments conducted on three public HSI datasets demonstrate that the proposed method has better performance compared with existing methods.
更多查看译文
关键词
hyperspectral image classification,fusion,multi-layer
AI 理解论文
溯源树
样例
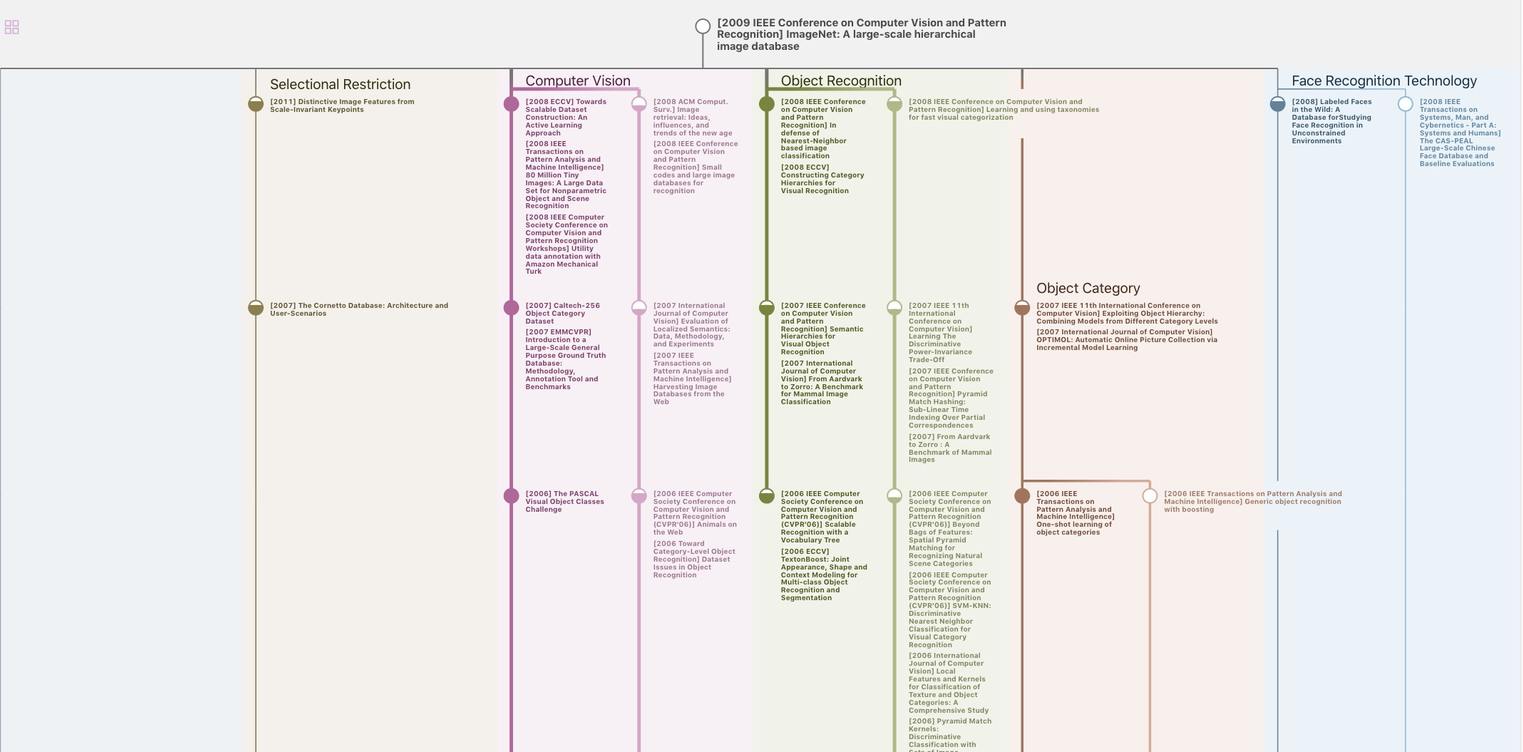
生成溯源树,研究论文发展脉络
Chat Paper
正在生成论文摘要