Grinding surface roughness prediction for silicon nitride ceramics: A dynamic grinding force and frequency domain approach
CERAMICS INTERNATIONAL(2023)
摘要
The existing surface roughness theoretical predictive models exhibit high parameter sensitivity and complexity, resulting in low reliability. Moreover, machine learning-based predictive models are constrained by the training parameter range, limiting their precision and generalization. In response to these limitations and from the frequency domain perspective, this paper proposes an innovative surface roughness (Ra) predictive model for silcon nitride ceramics grinding based on the dynamic grinding force model. First, a dynamic grinding force model was established using single grain scratch test results from both ductile and brittle removal stages, with model parameter estimation assisted by Genetic Algorithm. The results demonstrated a Mean Relative Error (MRE) of 19.51% between experimental and theoretical dynamic grinding force values, and a Relative Error (RE) of 9.37% for average grinding force. Subsequently, the frequency domain characteristics of both experimental grinding force signals and surface topography texture curves were extracted, which exhibited a significant correlation based on cosine similarity and Kendall's tau. Finally, leveraging the frequency domain characteristics of grinding force, the surface roughness predictive model was developed through the Convolutional Neural Network. The predictive model achieved an MRE of 12.79%, with random grinding experiments further confirming the model's accuracy with an MRE of 13.65%. These findings indicate that the proposed surface roughness predictive model offers high generalization capability and accurate prediction across a broader parameter range.
更多查看译文
关键词
Ceramic grinding,Dynamic grinding force model,Surface roughness prediction,Frequency-domain characteristics
AI 理解论文
溯源树
样例
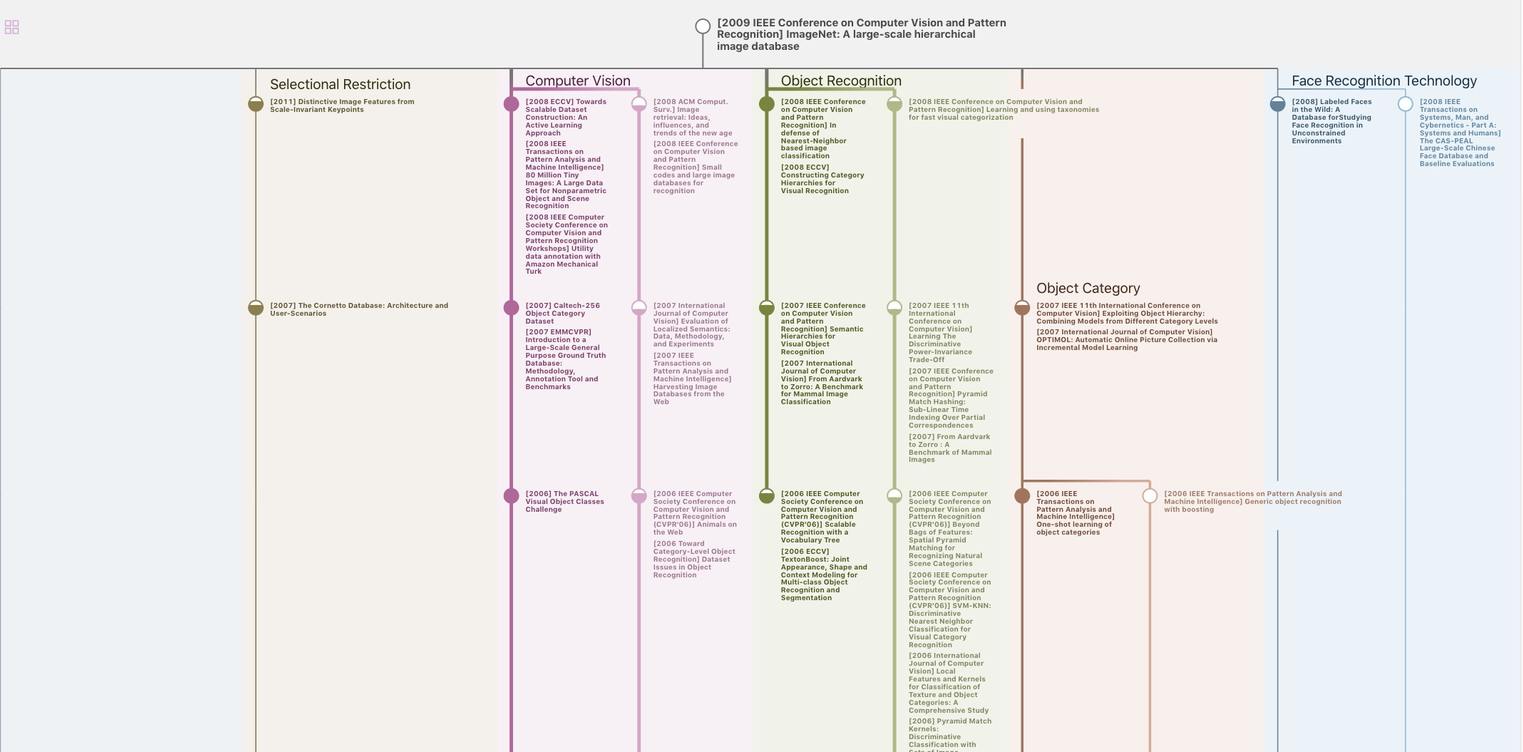
生成溯源树,研究论文发展脉络
Chat Paper
正在生成论文摘要