HPRE: Leveraging hierarchy-aware paired relation vectors for knowledge graph embedding
JOURNAL OF INTELLIGENT & FUZZY SYSTEMS(2023)
摘要
Knowledge graphs exhibit a typical hierarchical structure and find extensive applications in various artificial intelligence domains. However, large-scale knowledge graphs need to be completed, which limits the performance of knowledge graphs in downstream tasks. Knowledge graph embedding methods have emerged as a primary solution to enhance knowledge graph completeness. These methods aim to represent entities and relations as low-dimensional vectors, focusing on handling relation patterns and multi-relation types. Researchers need to pay more attention to the crucial feature of hierarchical relationships in real-world knowledge graphs. We propose a novel knowledge graph embedding model called Hierarchy-Aware Paired Relation Vectors Knowledge Graph Embedding (HPRE) to bridge this gap. By leveraging the power of 2D coordinates, HPRE adeptly model relation patterns, multi-relation types, and hierarchical features in the knowledge graph. Specifically, HPRE employs paired relation vectors to capture the distinct characteristics of head and tail entities, facilitating a better fit for relational patterns and multi-relation scenarios. Additionally, HPRE employs angular coordinates to differentiate entities at various levels of the hierarchy, effectively representing the hierarchical nature of the knowledge graph. The experimental results show that the HPRE model can effectively learn the hierarchical features of the knowledge graph and achieve state-of-the-art experimental results on multiple real-world datasets for the link prediction task.
更多查看译文
关键词
Knowledge graph completion,link prediction,knowledge graph embedding,knowledge graph representation
AI 理解论文
溯源树
样例
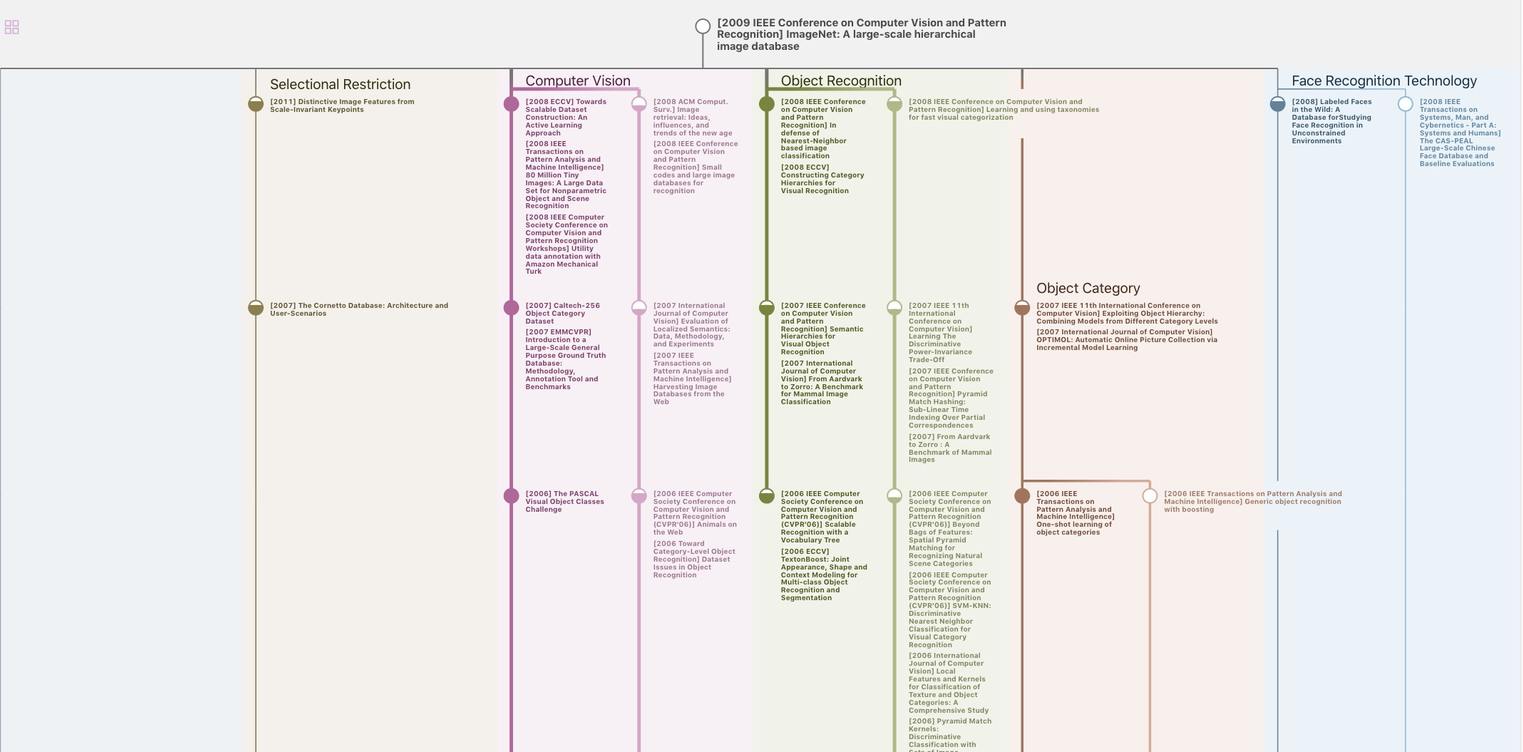
生成溯源树,研究论文发展脉络
Chat Paper
正在生成论文摘要