Grasping force estimation from two-channel electromyography signals using extreme learning machine
BIOMEDICAL ENGINEERING-APPLICATIONS BASIS COMMUNICATIONS(2024)
摘要
This research presents a method to select the best parameters of an extreme learning machine (ELM) for estimating grasping force from two-channel surface electromyography (sEMG) signals. Its advantages compared to the use of multi-channel sEMG signals include faster computing, lower electrode costs, and easier usage. The proposed method is appropriate for certain applications where time is very important but accuracy can be slightly compromised. The study recorded sEMG signals from 20 healthy volunteers, 10 males and 10 females, aged 22-52 years. The recorded sEMG signals were used in testing the proposed optimization method for grasping force estimation. It was found that the proposed method for optimizing the number of nodes, gain, and factor values would make the force estimation more efficient. The results show that the optimum number of nodes, gain, and factor values is 4, 0.05, and 1.0045, respectively. The resulting root mean square error and correlation coefficient values in force estimation from the ELM optimization method were 2.5642 and 0.9287, respectively, when the computing time was only 0.0038 s. These results show the feasibility of the proposed method for estimating force using only two-channel sEMG signals. As a result, real-time implementation, such as force estimation in robotic hand control for rehabilitation using sEMG signals, can be achieved efficiently.
更多查看译文
关键词
Electromyogram (EMG) signals,handgrip force,extreme learning machine,force estimation
AI 理解论文
溯源树
样例
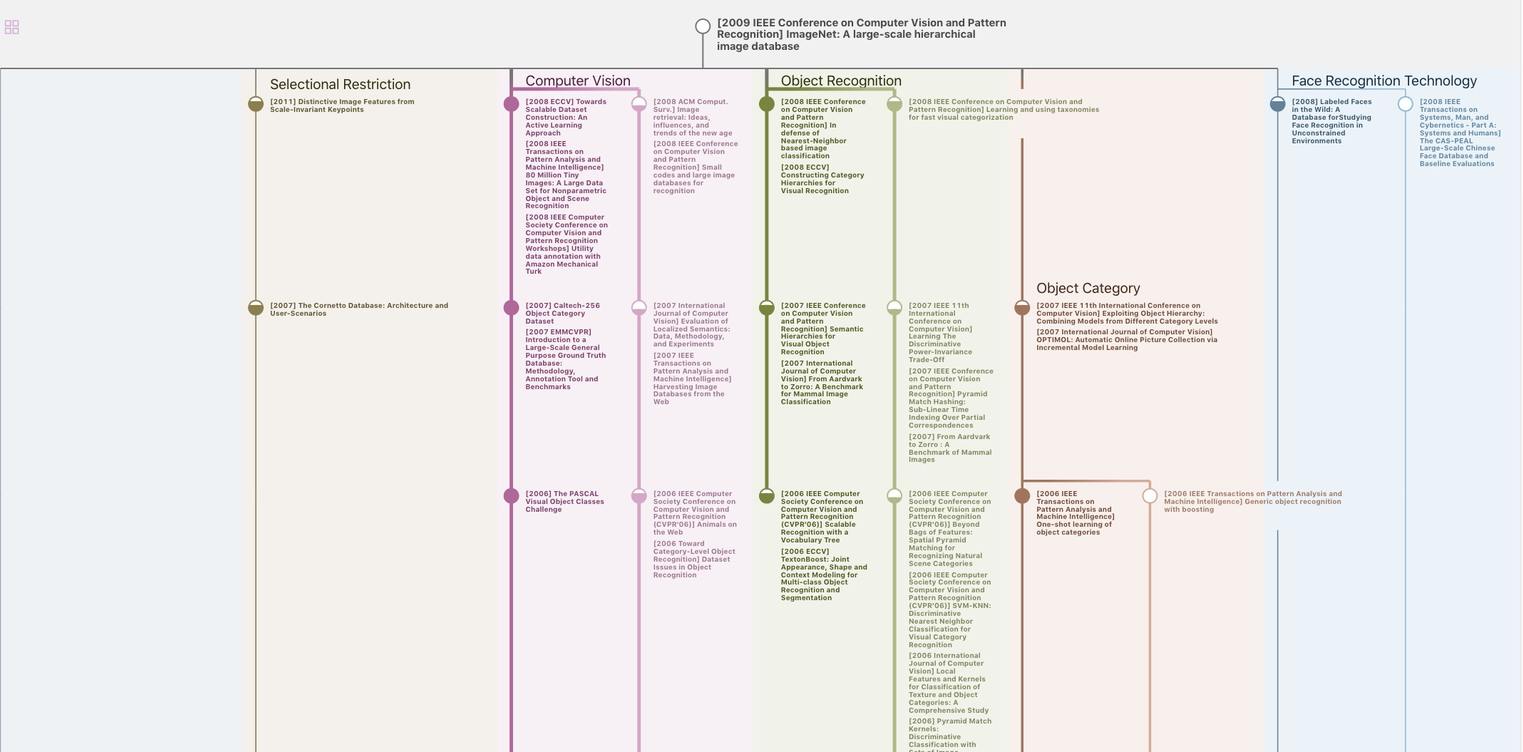
生成溯源树,研究论文发展脉络
Chat Paper
正在生成论文摘要