AP-Net: a metallic surface defect detection approach with lightweight adaptive attention and enhanced feature pyramid
CLUSTER COMPUTING-THE JOURNAL OF NETWORKS SOFTWARE TOOLS AND APPLICATIONS(2023)
摘要
Surface defect detection is essential for ensuring the quality of metallic products. Many excellent surface defect detectors have been designed in recent years. Most detection methods achieve success by using attention and feature pyramid modules. However, most attention modules consider only simple global information in channel features and incur heavy computational costs. Furthermore, the existing feature pyramids fail to effectively utilize the information from all multi-level features. To alleviate these issues, we design a metallic surface defect detector, named AP-Net. Specifically, we propose a lightweight adaptive attention module (LAA) and an enhanced feature pyramid module (EFP). We perform extensive experiments on three datasets. The experimental results show that the detection accuracies of the representative detectors can be significantly improved using our LAA and EFP.
更多查看译文
关键词
Surface defect detection,Object detection,Deep learning,Attention,Feature pyramid
AI 理解论文
溯源树
样例
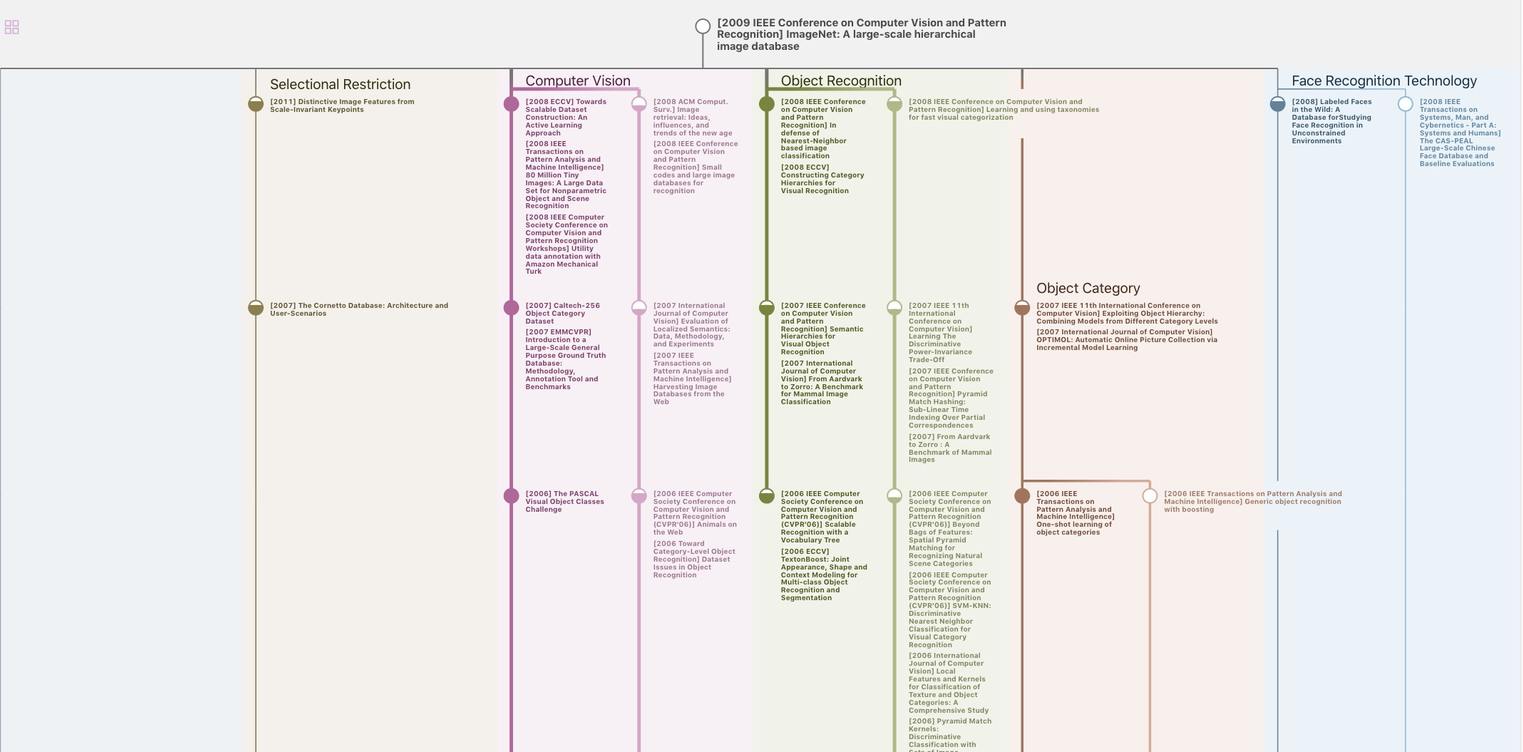
生成溯源树,研究论文发展脉络
Chat Paper
正在生成论文摘要