Optimization of sampling conditions to minimize sampling errors of both PM2.5 mass and its semi-volatile inorganic ion concentrations
AEROSOL SCIENCE AND TECHNOLOGY(2023)
摘要
The accurate measurement of PM2.5 and its inorganic matters (IMs) is crucial for compliance monitoring and understanding particle formation. However, semi-volatile IMs (SVIMs) like NH4+, NO3-, and Cl- tend to evaporate from particles, causing sampling artifacts. The evaporation loss occurs due to many factors making the quantitative prediction difficult. This study aimed to investigate the evaporation loss of SVIMs in PM2.5 under different sampling conditions. In the field tests, when a normal single Teflon filter (STF) sampler, which is like a Federal Reference Method (FRM) sampler, was used to sample PM2.5 at ambient conditions, a significant SVIM evaporation loss was observed, resulting in negative biases for total IMs (-25.68 +/- 3.25%) and PM2.5 concentrations (-9.87 +/- 4.27%). But if PM2.5 was sampled by a chilled Teflon filter sampler (CTF) at 4 degrees C following aerosol dehumidification so that relative humidity (RH) was controlled to within the 10-20% range (RHd), evaporation loss was minimized with a bias of < +/- 10% for both total IMs and PM2.5 based on the reference data. When RHd is below 10%, both IMs and PM2.5 are under-measured, but only PM2.5 is over-measured when RHd is >20%. A model considering predictable saturation ratios for NH4+, NO3-, and Cl- under various pressure drop, temperature and RH conditions was developed to predict accurately the actual concentrations of PM2.5 and its SVIMs for the STF. Additionally, the ISORROPIA-II model predicted SVIMs effectively for the CTF. In summary, using the CTF at optimized sampling conditions can achieve accurate measurement of both SVIMs and PM2.5 concentrations simultaneously.Copyright (c) 2023 American Association for Aerosol Research{Graphical Abstract}
更多查看译文
关键词
Kihong Park
AI 理解论文
溯源树
样例
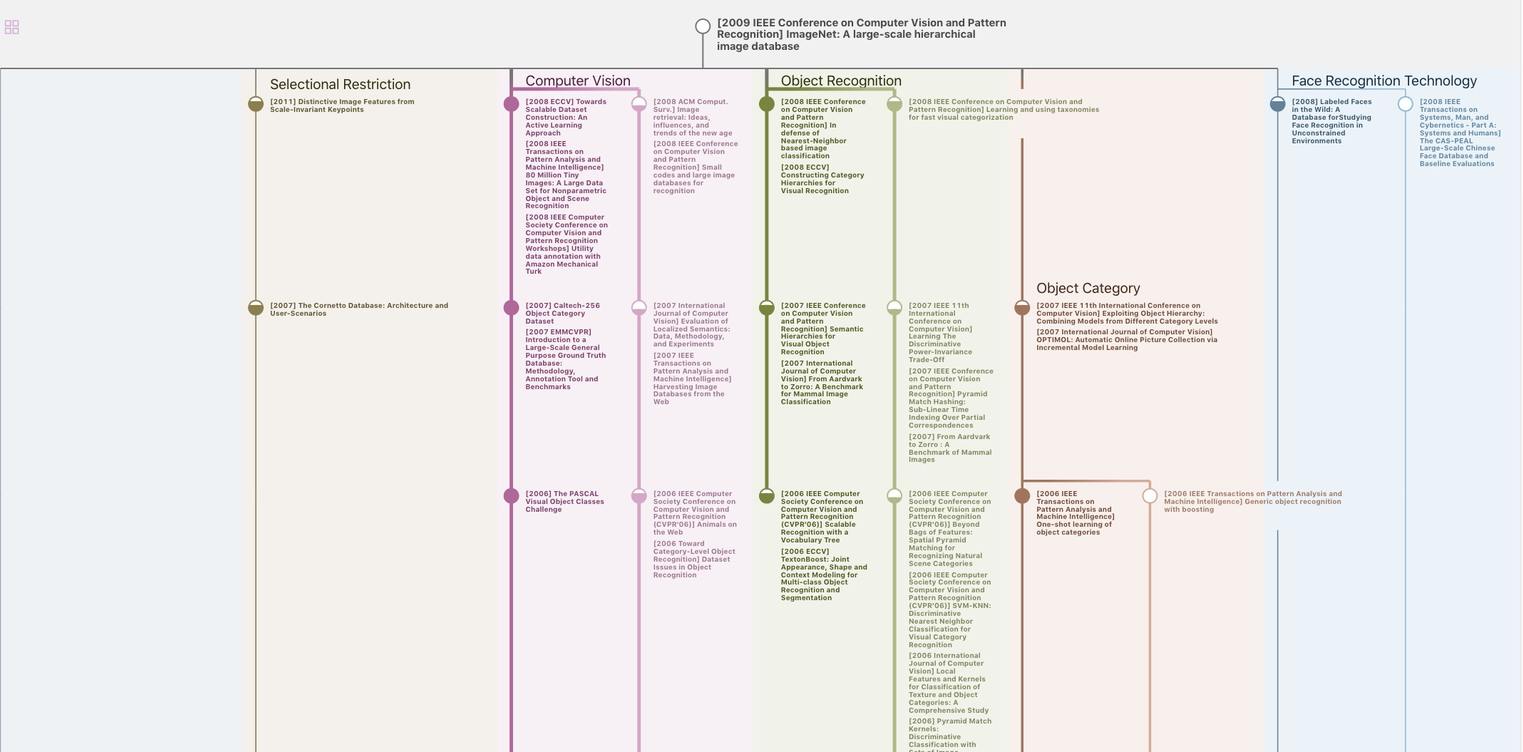
生成溯源树,研究论文发展脉络
Chat Paper
正在生成论文摘要