Additive margin networks with adaptive feature recalibration and its applications in Brain Stroke CT Image classification
IET IMAGE PROCESSING(2024)
摘要
In this paper, an additive margin network with adaptive feature recalibration, AMNets, for handling a wide range of visual tasks, is proposed. The new AMNets consists of three improvements on convolutional neural networks: (1) squeeze-and-excitation mechanism was embedded into the the residual block of single layer to improve the performance of the classification, (2) softmax loss was replaced by the additive margin softmax loss to minimize intra-class variance and maximize inter-class variance, and (3) Adam optimizer was exploited to update iteratively the network parameters. Experiments on the Brain Stroke CT Image Dataset show that our additive margin network is quite effective to improve state-of-the-art algorithms. Network algorithms have been extensively used in medical image field to pursuit a more accuracy of disease recognition. This paper proposes AMNets, an additive margin network with adaptive feature recalibration for handling a wide range of visual tasks in medicine.
更多查看译文
关键词
brain,image recognition,Convolutional neural networks,Additive margin softmax loss,Adaptive feature recalibration
AI 理解论文
溯源树
样例
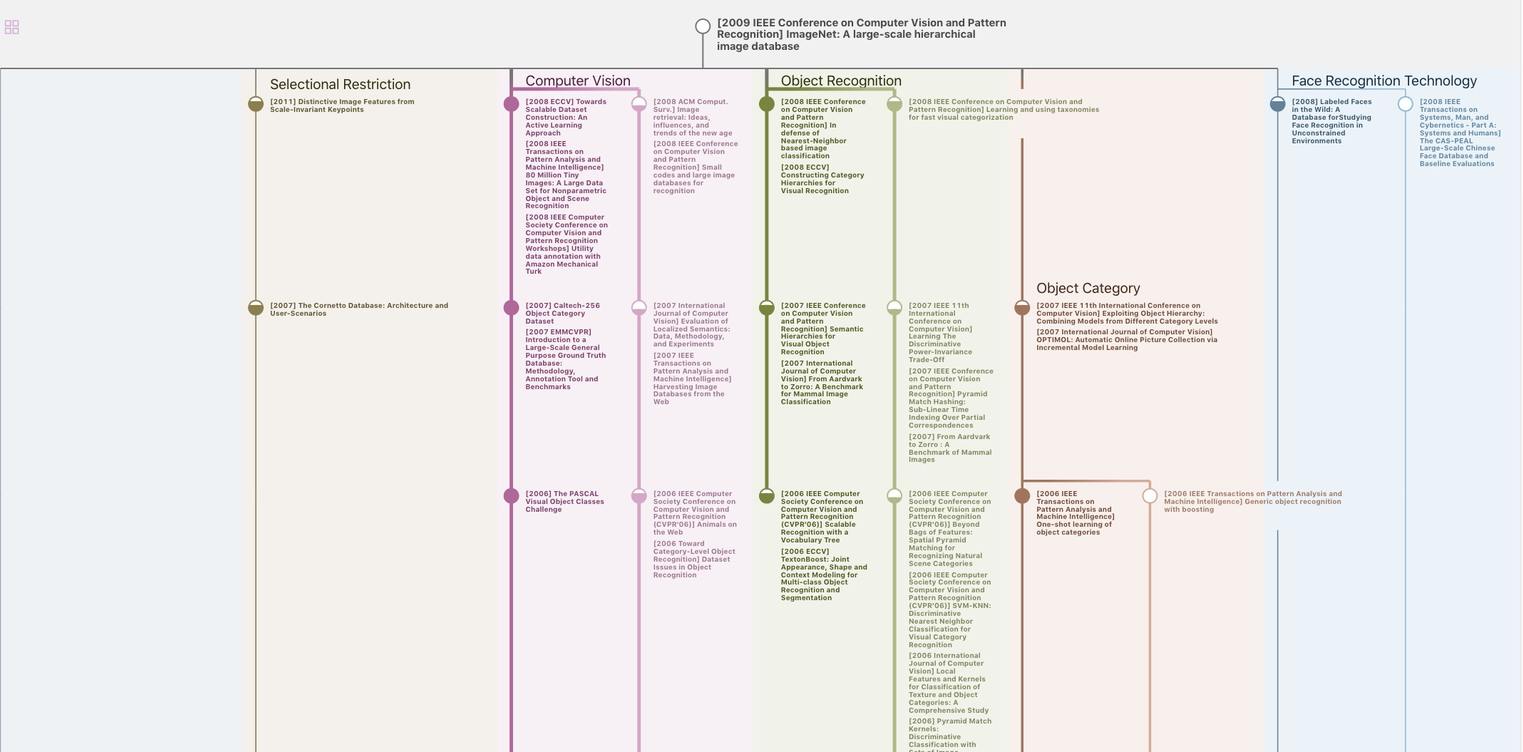
生成溯源树,研究论文发展脉络
Chat Paper
正在生成论文摘要