Hierarchical critic learning optimal control for lower limb exoskeleton robots with prescribed constraints
INTERNATIONAL JOURNAL OF ROBUST AND NONLINEAR CONTROL(2024)
摘要
This paper presents a hierarchical critic learning optimal control (H-CLOC) strategy for a lower limb exoskeleton system with prescribed state constraints. The prescribed state constraints of the exoskeleton are mathematically modeled by means of a state error transition function, which transforms the constrained state into an equivalent new unconstrained state. A hierarchical framework including a high-level motion trajectory planner and a low-level critic learning (CL) controller is proposed for optimal control of the exoskeleton. The estimation of human motion intentions is achieved using dynamic movement primitives (DMPs), which are capable of accurately replicating the original training trajectories during motion. The CL algorithm is implemented on the transformed unconstrained system while enhancing the learning capability of the proposed algorithm for the exoskeleton. The optimal control with prescribed constraints is analyzed for stability using the Lyapunov theorem. Finally, simulation results validate the effectiveness of the proposed H-CLOC strategy for lower limb exoskeletons.
更多查看译文
关键词
critic neural network,lower limb exoskeleton,nonlinear control,prescribed constraints
AI 理解论文
溯源树
样例
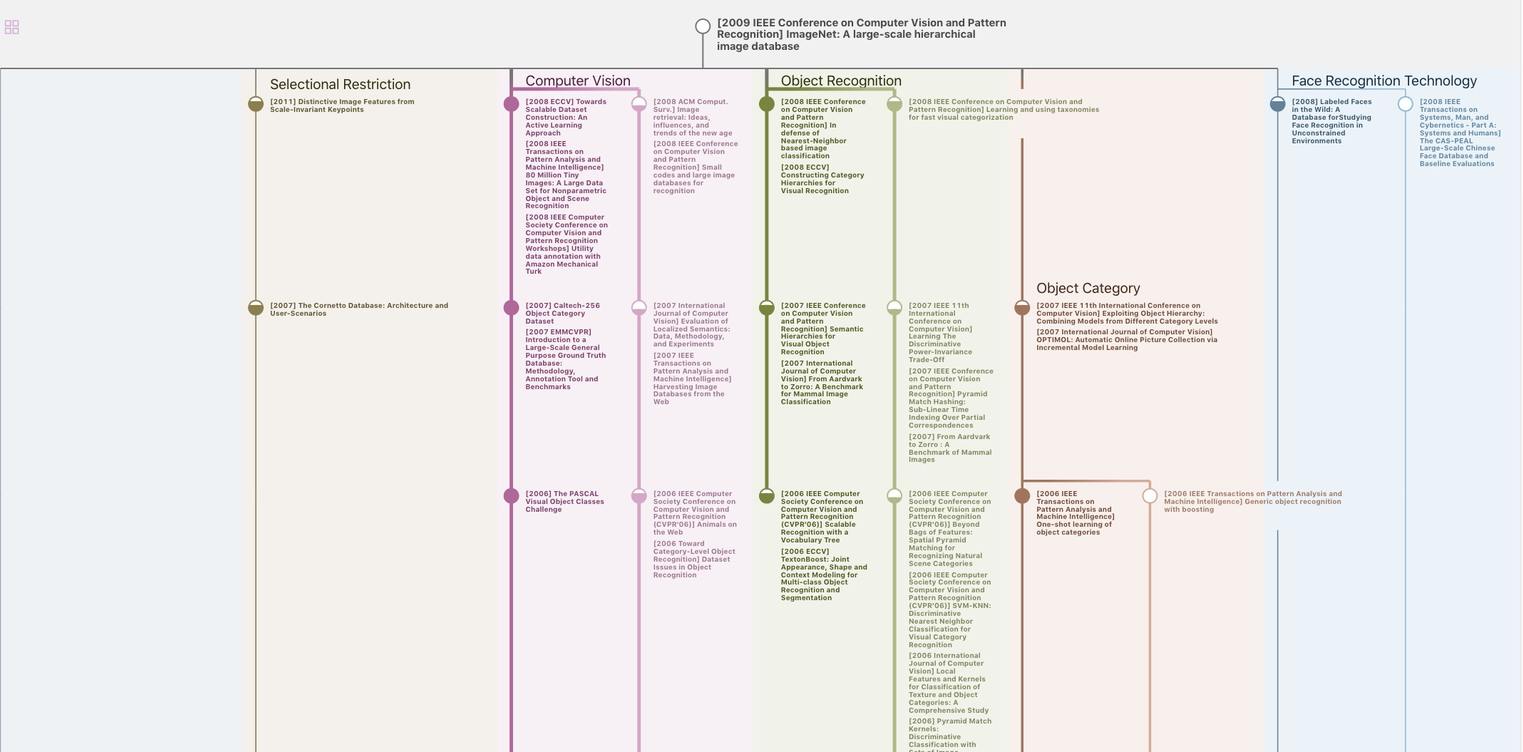
生成溯源树,研究论文发展脉络
Chat Paper
正在生成论文摘要