Neuropsych BrainAge: A biomarker for conversion from mild cognitive impairment to Alzheimer's disease
ALZHEIMER'S & DEMENTIA: DIAGNOSIS, ASSESSMENT & DISEASE MONITORING(2023)
摘要
INTRODUCTION BrainAge models based on neuroimaging data have diagnostic classification power but have replicability issues due to site and patient variability. BrainAge models trained on neuropsychological tests could help distinguish stable mild cognitive impairment (sMCI) from progressive MCI (pMCI) to Alzheimer's disease (AD).METHODS A linear regressor BrainAge model was trained on healthy controls using neuropsychological tests and neuroimaging features separately. The BrainAge delta, predicted age minus chronological age, was used to distinguish between sMCI and pMCI.RESULTS The cross-validated area under the receiver-operating characteristic (ROC) curve for sMCI versus pMCI was 0.91 for neuropsychological features in contrast to 0.68 for neuroimaging features. The BrainAge delta was correlated with the time to conversion, the time taken for a pMCI subject to convert to AD.DISCUSSION The BrainAge delta from neuropsychological tests is a good biomarker to distinguish between sMCI and pMCI. Other neurological and psychiatric disorders could be studied using this strategy.Highlights BrainAge models based on neuropsychological tests outperform models based on neuroimaging features when distinguishing between stable mild cognitive impairment (sMCI) from progressive MCI (pMCI) to Alzheimer's disease (AD). The combination of neuropsychological tests with neuroimaging features does not lead to an improvement in sMCI versus pMCI classification compared to using neuropsychological tests on their own. BrainAge delta of both neuroimaging and neuropsychological models was correlated with the time to conversion, the time taken for a pMCI subject to convert to AD.
更多查看译文
关键词
Alzheimer's disease, biomarker, BrainAge, mild cognitive impairment, neuropsychological test
AI 理解论文
溯源树
样例
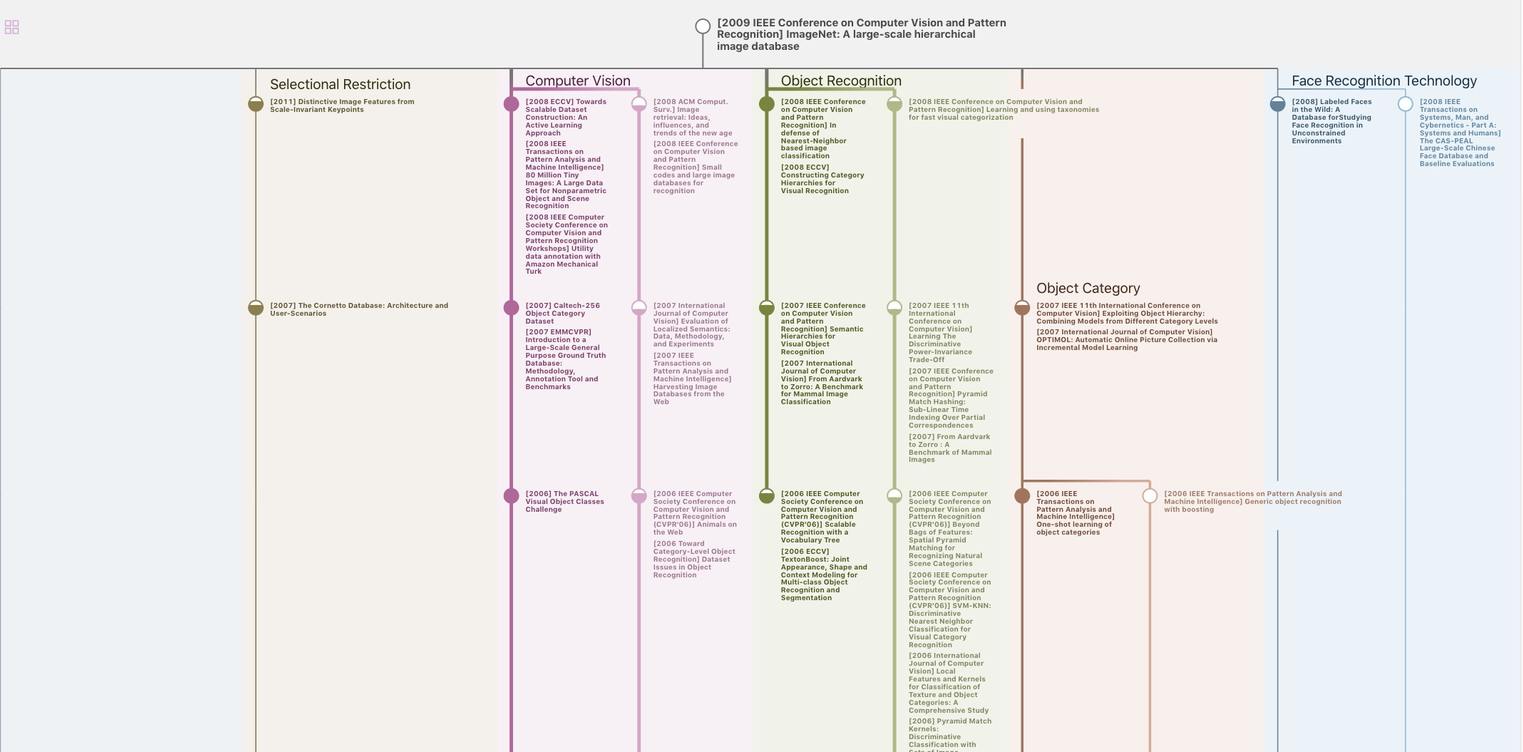
生成溯源树,研究论文发展脉络
Chat Paper
正在生成论文摘要